- En español – ExME
- Em português – EME

An introduction to different types of study design
Posted on 6th April 2021 by Hadi Abbas
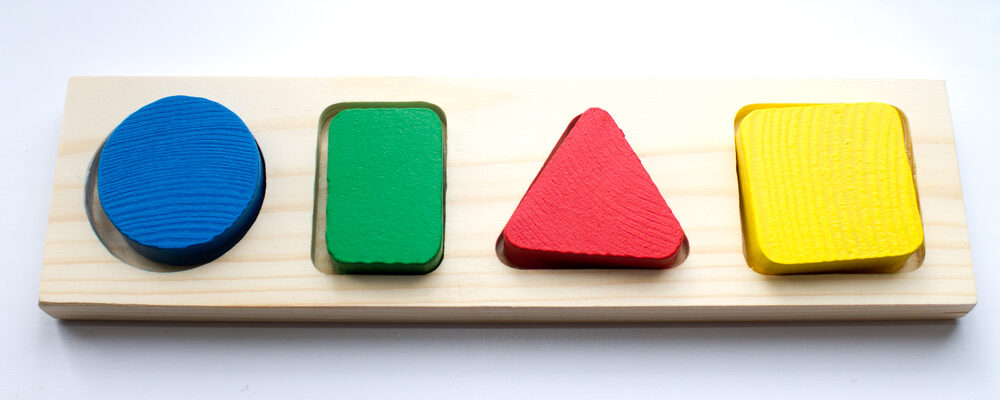
Study designs are the set of methods and procedures used to collect and analyze data in a study.
Broadly speaking, there are 2 types of study designs: descriptive studies and analytical studies.
Descriptive studies
- Describes specific characteristics in a population of interest
- The most common forms are case reports and case series
- In a case report, we discuss our experience with the patient’s symptoms, signs, diagnosis, and treatment
- In a case series, several patients with similar experiences are grouped.
Analytical Studies
Analytical studies are of 2 types: observational and experimental.
Observational studies are studies that we conduct without any intervention or experiment. In those studies, we purely observe the outcomes. On the other hand, in experimental studies, we conduct experiments and interventions.
Observational studies
Observational studies include many subtypes. Below, I will discuss the most common designs.
Cross-sectional study:
- This design is transverse where we take a specific sample at a specific time without any follow-up
- It allows us to calculate the frequency of disease ( p revalence ) or the frequency of a risk factor
- This design is easy to conduct
- For example – if we want to know the prevalence of migraine in a population, we can conduct a cross-sectional study whereby we take a sample from the population and calculate the number of patients with migraine headaches.
Cohort study:
- We conduct this study by comparing two samples from the population: one sample with a risk factor while the other lacks this risk factor
- It shows us the risk of developing the disease in individuals with the risk factor compared to those without the risk factor ( RR = relative risk )
- Prospective : we follow the individuals in the future to know who will develop the disease
- Retrospective : we look to the past to know who developed the disease (e.g. using medical records)
- This design is the strongest among the observational studies
- For example – to find out the relative risk of developing chronic obstructive pulmonary disease (COPD) among smokers, we take a sample including smokers and non-smokers. Then, we calculate the number of individuals with COPD among both.
Case-Control Study:
- We conduct this study by comparing 2 groups: one group with the disease (cases) and another group without the disease (controls)
- This design is always retrospective
- We aim to find out the odds of having a risk factor or an exposure if an individual has a specific disease (Odds ratio)
- Relatively easy to conduct
- For example – we want to study the odds of being a smoker among hypertensive patients compared to normotensive ones. To do so, we choose a group of patients diagnosed with hypertension and another group that serves as the control (normal blood pressure). Then we study their smoking history to find out if there is a correlation.
Experimental Studies
- Also known as interventional studies
- Can involve animals and humans
- Pre-clinical trials involve animals
- Clinical trials are experimental studies involving humans
- In clinical trials, we study the effect of an intervention compared to another intervention or placebo. As an example, I have listed the four phases of a drug trial:
I: We aim to assess the safety of the drug ( is it safe ? )
II: We aim to assess the efficacy of the drug ( does it work ? )
III: We want to know if this drug is better than the old treatment ( is it better ? )
IV: We follow-up to detect long-term side effects ( can it stay in the market ? )
- In randomized controlled trials, one group of participants receives the control, while the other receives the tested drug/intervention. Those studies are the best way to evaluate the efficacy of a treatment.
Finally, the figure below will help you with your understanding of different types of study designs.
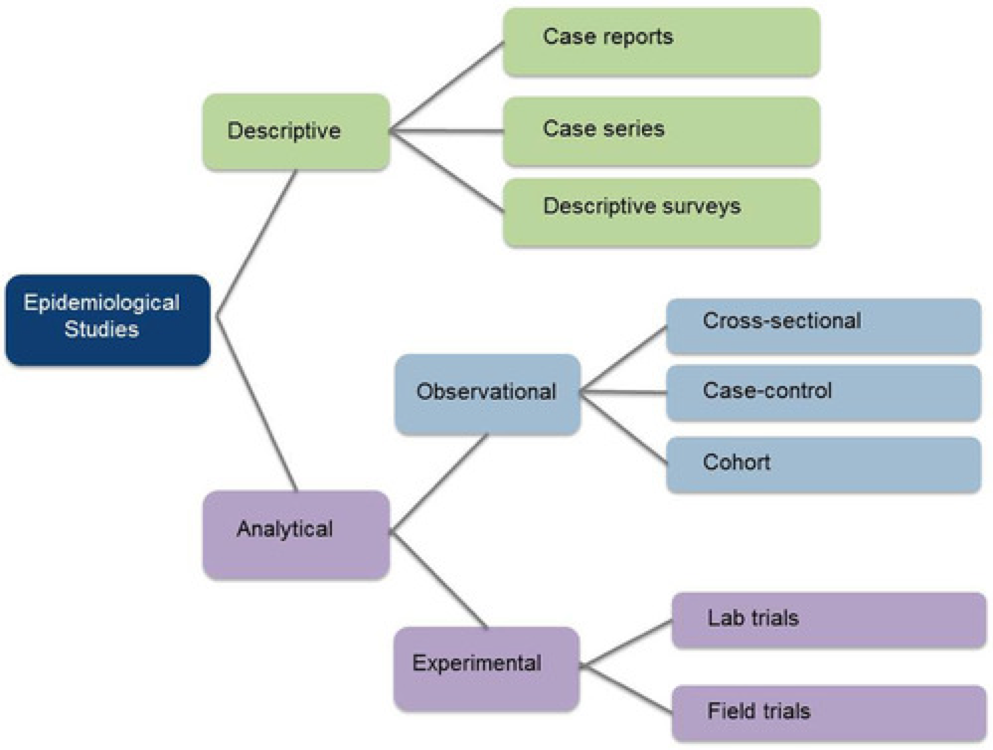
References (pdf)
You may also be interested in the following blogs for further reading:
An introduction to randomized controlled trials
Case-control and cohort studies: a brief overview
Cohort studies: prospective and retrospective designs
Prevalence vs Incidence: what is the difference?
Leave a Reply Cancel reply
Your email address will not be published. Required fields are marked *
Save my name, email, and website in this browser for the next time I comment.
No Comments on An introduction to different types of study design
you are amazing one!! if I get you I’m working with you! I’m student from Ethiopian higher education. health sciences student
Very informative and easy understandable
You are my kind of doctor. Do not lose sight of your objective.
Wow very erll explained and easy to understand
I’m Khamisu Habibu community health officer student from Abubakar Tafawa Balewa university teaching hospital Bauchi, Nigeria, I really appreciate your write up and you have make it clear for the learner. thank you
well understood,thank you so much
Well understood…thanks
Simply explained. Thank You.
Thanks a lot for this nice informative article which help me to understand different study designs that I felt difficult before
That’s lovely to hear, Mona, thank you for letting the author know how useful this was. If there are any other particular topics you think would be useful to you, and are not already on the website, please do let us know.
it is very informative and useful.
thank you statistician
Fabulous to hear, thank you John.
Thanks for this information
Thanks so much for this information….I have clearly known the types of study design Thanks
That’s so good to hear, Mirembe, thank you for letting the author know.
Very helpful article!! U have simplified everything for easy understanding
I’m a health science major currently taking statistics for health care workers…this is a challenging class…thanks for the simified feedback.
That’s good to hear this has helped you. Hopefully you will find some of the other blogs useful too. If you see any topics that are missing from the website, please do let us know!
Hello. I liked your presentation, the fact that you ranked them clearly is very helpful to understand for people like me who is a novelist researcher. However, I was expecting to read much more about the Experimental studies. So please direct me if you already have or will one day. Thank you
Dear Ay. My sincere apologies for not responding to your comment sooner. You may find it useful to filter the blogs by the topic of ‘Study design and research methods’ – here is a link to that filter: https://s4be.cochrane.org/blog/topic/study-design/ This will cover more detail about experimental studies. Or have a look on our library page for further resources there – you’ll find that on the ‘Resources’ drop down from the home page.
However, if there are specific things you feel you would like to learn about experimental studies, that are missing from the website, it would be great if you could let me know too. Thank you, and best of luck. Emma
Great job Mr Hadi. I advise you to prepare and study for the Australian Medical Board Exams as soon as you finish your undergrad study in Lebanon. Good luck and hope we can meet sometime in the future. Regards ;)
You have give a good explaination of what am looking for. However, references am not sure of where to get them from.
Subscribe to our newsletter
You will receive our monthly newsletter and free access to Trip Premium.
Related Articles
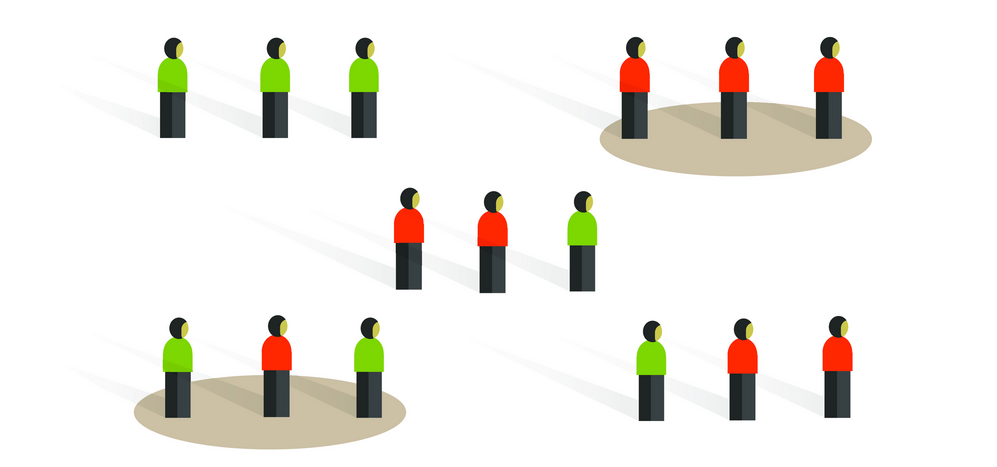
Cluster Randomized Trials: Concepts
This blog summarizes the concepts of cluster randomization, and the logistical and statistical considerations while designing a cluster randomized controlled trial.
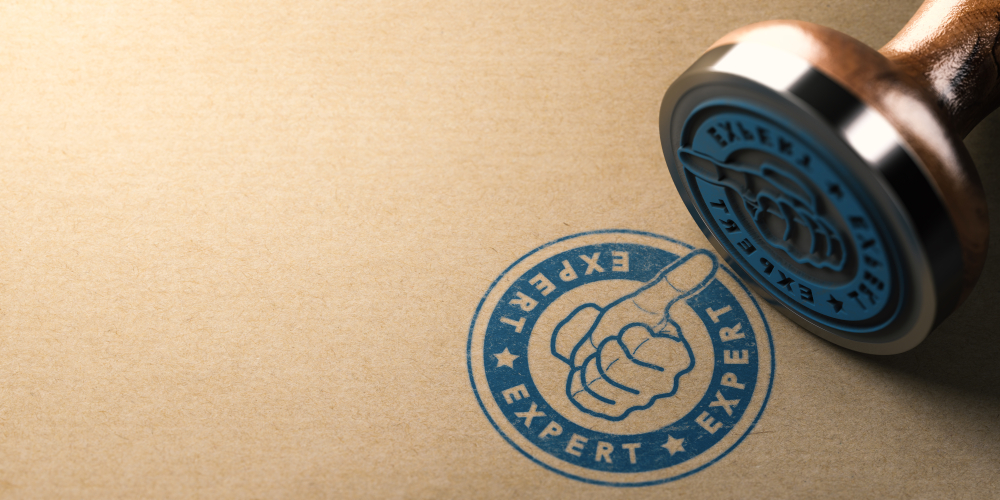
Expertise-based Randomized Controlled Trials
This blog summarizes the concepts of Expertise-based randomized controlled trials with a focus on the advantages and challenges associated with this type of study.
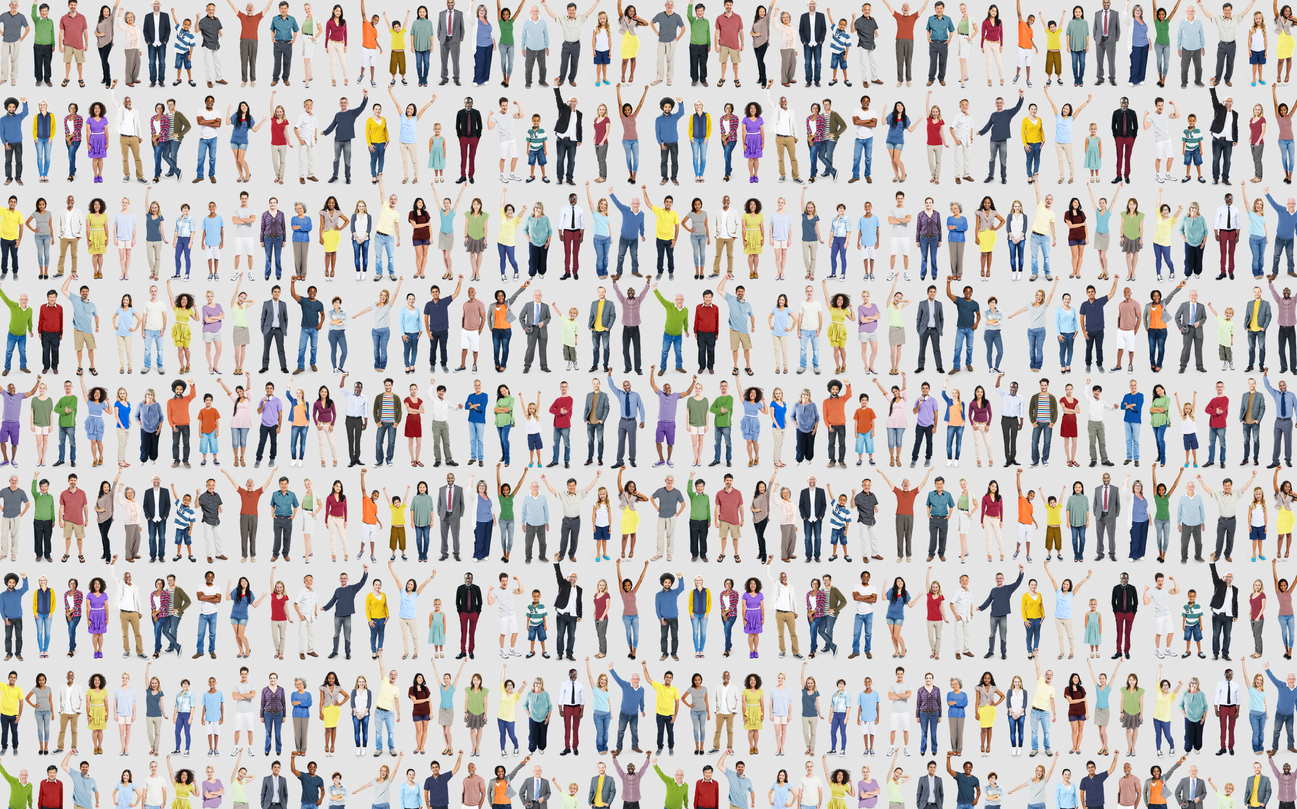
A well-designed cohort study can provide powerful results. This blog introduces prospective and retrospective cohort studies, discussing the advantages, disadvantages and use of these type of study designs.
- Privacy Policy

Home » Research Design – Types, Methods and Examples
Research Design – Types, Methods and Examples
Table of Contents

Research Design
Definition:
Research design refers to the overall strategy or plan for conducting a research study. It outlines the methods and procedures that will be used to collect and analyze data, as well as the goals and objectives of the study. Research design is important because it guides the entire research process and ensures that the study is conducted in a systematic and rigorous manner.
Types of Research Design
Types of Research Design are as follows:
Descriptive Research Design
This type of research design is used to describe a phenomenon or situation. It involves collecting data through surveys, questionnaires, interviews, and observations. The aim of descriptive research is to provide an accurate and detailed portrayal of a particular group, event, or situation. It can be useful in identifying patterns, trends, and relationships in the data.
Correlational Research Design
Correlational research design is used to determine if there is a relationship between two or more variables. This type of research design involves collecting data from participants and analyzing the relationship between the variables using statistical methods. The aim of correlational research is to identify the strength and direction of the relationship between the variables.
Experimental Research Design
Experimental research design is used to investigate cause-and-effect relationships between variables. This type of research design involves manipulating one variable and measuring the effect on another variable. It usually involves randomly assigning participants to groups and manipulating an independent variable to determine its effect on a dependent variable. The aim of experimental research is to establish causality.
Quasi-experimental Research Design
Quasi-experimental research design is similar to experimental research design, but it lacks one or more of the features of a true experiment. For example, there may not be random assignment to groups or a control group. This type of research design is used when it is not feasible or ethical to conduct a true experiment.
Case Study Research Design
Case study research design is used to investigate a single case or a small number of cases in depth. It involves collecting data through various methods, such as interviews, observations, and document analysis. The aim of case study research is to provide an in-depth understanding of a particular case or situation.
Longitudinal Research Design
Longitudinal research design is used to study changes in a particular phenomenon over time. It involves collecting data at multiple time points and analyzing the changes that occur. The aim of longitudinal research is to provide insights into the development, growth, or decline of a particular phenomenon over time.
Structure of Research Design
The format of a research design typically includes the following sections:
- Introduction : This section provides an overview of the research problem, the research questions, and the importance of the study. It also includes a brief literature review that summarizes previous research on the topic and identifies gaps in the existing knowledge.
- Research Questions or Hypotheses: This section identifies the specific research questions or hypotheses that the study will address. These questions should be clear, specific, and testable.
- Research Methods : This section describes the methods that will be used to collect and analyze data. It includes details about the study design, the sampling strategy, the data collection instruments, and the data analysis techniques.
- Data Collection: This section describes how the data will be collected, including the sample size, data collection procedures, and any ethical considerations.
- Data Analysis: This section describes how the data will be analyzed, including the statistical techniques that will be used to test the research questions or hypotheses.
- Results : This section presents the findings of the study, including descriptive statistics and statistical tests.
- Discussion and Conclusion : This section summarizes the key findings of the study, interprets the results, and discusses the implications of the findings. It also includes recommendations for future research.
- References : This section lists the sources cited in the research design.
Example of Research Design
An Example of Research Design could be:
Research question: Does the use of social media affect the academic performance of high school students?
Research design:
- Research approach : The research approach will be quantitative as it involves collecting numerical data to test the hypothesis.
- Research design : The research design will be a quasi-experimental design, with a pretest-posttest control group design.
- Sample : The sample will be 200 high school students from two schools, with 100 students in the experimental group and 100 students in the control group.
- Data collection : The data will be collected through surveys administered to the students at the beginning and end of the academic year. The surveys will include questions about their social media usage and academic performance.
- Data analysis : The data collected will be analyzed using statistical software. The mean scores of the experimental and control groups will be compared to determine whether there is a significant difference in academic performance between the two groups.
- Limitations : The limitations of the study will be acknowledged, including the fact that social media usage can vary greatly among individuals, and the study only focuses on two schools, which may not be representative of the entire population.
- Ethical considerations: Ethical considerations will be taken into account, such as obtaining informed consent from the participants and ensuring their anonymity and confidentiality.
How to Write Research Design
Writing a research design involves planning and outlining the methodology and approach that will be used to answer a research question or hypothesis. Here are some steps to help you write a research design:
- Define the research question or hypothesis : Before beginning your research design, you should clearly define your research question or hypothesis. This will guide your research design and help you select appropriate methods.
- Select a research design: There are many different research designs to choose from, including experimental, survey, case study, and qualitative designs. Choose a design that best fits your research question and objectives.
- Develop a sampling plan : If your research involves collecting data from a sample, you will need to develop a sampling plan. This should outline how you will select participants and how many participants you will include.
- Define variables: Clearly define the variables you will be measuring or manipulating in your study. This will help ensure that your results are meaningful and relevant to your research question.
- Choose data collection methods : Decide on the data collection methods you will use to gather information. This may include surveys, interviews, observations, experiments, or secondary data sources.
- Create a data analysis plan: Develop a plan for analyzing your data, including the statistical or qualitative techniques you will use.
- Consider ethical concerns : Finally, be sure to consider any ethical concerns related to your research, such as participant confidentiality or potential harm.
When to Write Research Design
Research design should be written before conducting any research study. It is an important planning phase that outlines the research methodology, data collection methods, and data analysis techniques that will be used to investigate a research question or problem. The research design helps to ensure that the research is conducted in a systematic and logical manner, and that the data collected is relevant and reliable.
Ideally, the research design should be developed as early as possible in the research process, before any data is collected. This allows the researcher to carefully consider the research question, identify the most appropriate research methodology, and plan the data collection and analysis procedures in advance. By doing so, the research can be conducted in a more efficient and effective manner, and the results are more likely to be valid and reliable.
Purpose of Research Design
The purpose of research design is to plan and structure a research study in a way that enables the researcher to achieve the desired research goals with accuracy, validity, and reliability. Research design is the blueprint or the framework for conducting a study that outlines the methods, procedures, techniques, and tools for data collection and analysis.
Some of the key purposes of research design include:
- Providing a clear and concise plan of action for the research study.
- Ensuring that the research is conducted ethically and with rigor.
- Maximizing the accuracy and reliability of the research findings.
- Minimizing the possibility of errors, biases, or confounding variables.
- Ensuring that the research is feasible, practical, and cost-effective.
- Determining the appropriate research methodology to answer the research question(s).
- Identifying the sample size, sampling method, and data collection techniques.
- Determining the data analysis method and statistical tests to be used.
- Facilitating the replication of the study by other researchers.
- Enhancing the validity and generalizability of the research findings.
Applications of Research Design
There are numerous applications of research design in various fields, some of which are:
- Social sciences: In fields such as psychology, sociology, and anthropology, research design is used to investigate human behavior and social phenomena. Researchers use various research designs, such as experimental, quasi-experimental, and correlational designs, to study different aspects of social behavior.
- Education : Research design is essential in the field of education to investigate the effectiveness of different teaching methods and learning strategies. Researchers use various designs such as experimental, quasi-experimental, and case study designs to understand how students learn and how to improve teaching practices.
- Health sciences : In the health sciences, research design is used to investigate the causes, prevention, and treatment of diseases. Researchers use various designs, such as randomized controlled trials, cohort studies, and case-control studies, to study different aspects of health and healthcare.
- Business : Research design is used in the field of business to investigate consumer behavior, marketing strategies, and the impact of different business practices. Researchers use various designs, such as survey research, experimental research, and case studies, to study different aspects of the business world.
- Engineering : In the field of engineering, research design is used to investigate the development and implementation of new technologies. Researchers use various designs, such as experimental research and case studies, to study the effectiveness of new technologies and to identify areas for improvement.
Advantages of Research Design
Here are some advantages of research design:
- Systematic and organized approach : A well-designed research plan ensures that the research is conducted in a systematic and organized manner, which makes it easier to manage and analyze the data.
- Clear objectives: The research design helps to clarify the objectives of the study, which makes it easier to identify the variables that need to be measured, and the methods that need to be used to collect and analyze data.
- Minimizes bias: A well-designed research plan minimizes the chances of bias, by ensuring that the data is collected and analyzed objectively, and that the results are not influenced by the researcher’s personal biases or preferences.
- Efficient use of resources: A well-designed research plan helps to ensure that the resources (time, money, and personnel) are used efficiently and effectively, by focusing on the most important variables and methods.
- Replicability: A well-designed research plan makes it easier for other researchers to replicate the study, which enhances the credibility and reliability of the findings.
- Validity: A well-designed research plan helps to ensure that the findings are valid, by ensuring that the methods used to collect and analyze data are appropriate for the research question.
- Generalizability : A well-designed research plan helps to ensure that the findings can be generalized to other populations, settings, or situations, which increases the external validity of the study.
Research Design Vs Research Methodology
Research Design | Research Methodology |
---|---|
The plan and structure for conducting research that outlines the procedures to be followed to collect and analyze data. | The set of principles, techniques, and tools used to carry out the research plan and achieve research objectives. |
Describes the overall approach and strategy used to conduct research, including the type of data to be collected, the sources of data, and the methods for collecting and analyzing data. | Refers to the techniques and methods used to gather, analyze and interpret data, including sampling techniques, data collection methods, and data analysis techniques. |
Helps to ensure that the research is conducted in a systematic, rigorous, and valid way, so that the results are reliable and can be used to make sound conclusions. | Includes a set of procedures and tools that enable researchers to collect and analyze data in a consistent and valid manner, regardless of the research design used. |
Common research designs include experimental, quasi-experimental, correlational, and descriptive studies. | Common research methodologies include qualitative, quantitative, and mixed-methods approaches. |
Determines the overall structure of the research project and sets the stage for the selection of appropriate research methodologies. | Guides the researcher in selecting the most appropriate research methods based on the research question, research design, and other contextual factors. |
Helps to ensure that the research project is feasible, relevant, and ethical. | Helps to ensure that the data collected is accurate, valid, and reliable, and that the research findings can be interpreted and generalized to the population of interest. |
About the author
Muhammad Hassan
Researcher, Academic Writer, Web developer
You may also like

Research Methodology – Types, Examples and...

Purpose of Research – Objectives and Applications

Research Paper Conclusion – Writing Guide and...

Research Paper Title – Writing Guide and Example

Significance of the Study – Examples and Writing...

Conceptual Framework – Types, Methodology and...
Leave a comment x.
Save my name, email, and website in this browser for the next time I comment.

Research Design 101
Everything You Need To Get Started (With Examples)
By: Derek Jansen (MBA) | Reviewers: Eunice Rautenbach (DTech) & Kerryn Warren (PhD) | April 2023
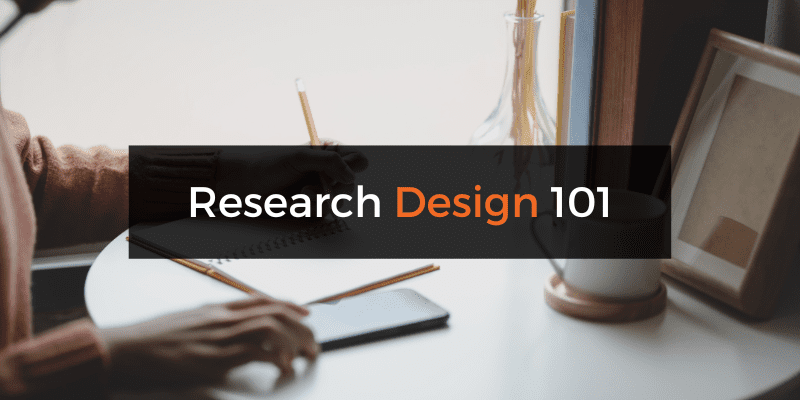
Navigating the world of research can be daunting, especially if you’re a first-time researcher. One concept you’re bound to run into fairly early in your research journey is that of “ research design ”. Here, we’ll guide you through the basics using practical examples , so that you can approach your research with confidence.
Overview: Research Design 101
What is research design.
- Research design types for quantitative studies
- Video explainer : quantitative research design
- Research design types for qualitative studies
- Video explainer : qualitative research design
- How to choose a research design
- Key takeaways
Research design refers to the overall plan, structure or strategy that guides a research project , from its conception to the final data analysis. A good research design serves as the blueprint for how you, as the researcher, will collect and analyse data while ensuring consistency, reliability and validity throughout your study.
Understanding different types of research designs is essential as helps ensure that your approach is suitable given your research aims, objectives and questions , as well as the resources you have available to you. Without a clear big-picture view of how you’ll design your research, you run the risk of potentially making misaligned choices in terms of your methodology – especially your sampling , data collection and data analysis decisions.
The problem with defining research design…
One of the reasons students struggle with a clear definition of research design is because the term is used very loosely across the internet, and even within academia.
Some sources claim that the three research design types are qualitative, quantitative and mixed methods , which isn’t quite accurate (these just refer to the type of data that you’ll collect and analyse). Other sources state that research design refers to the sum of all your design choices, suggesting it’s more like a research methodology . Others run off on other less common tangents. No wonder there’s confusion!
In this article, we’ll clear up the confusion. We’ll explain the most common research design types for both qualitative and quantitative research projects, whether that is for a full dissertation or thesis, or a smaller research paper or article.
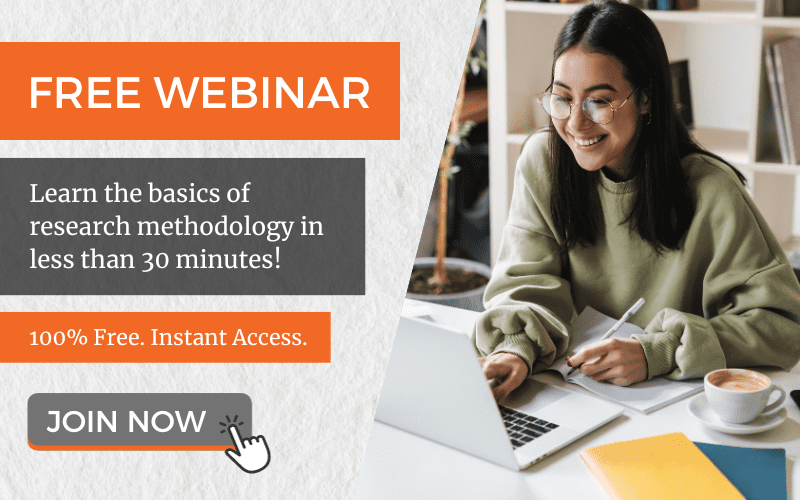
Research Design: Quantitative Studies
Quantitative research involves collecting and analysing data in a numerical form. Broadly speaking, there are four types of quantitative research designs: descriptive , correlational , experimental , and quasi-experimental .
Descriptive Research Design
As the name suggests, descriptive research design focuses on describing existing conditions, behaviours, or characteristics by systematically gathering information without manipulating any variables. In other words, there is no intervention on the researcher’s part – only data collection.
For example, if you’re studying smartphone addiction among adolescents in your community, you could deploy a survey to a sample of teens asking them to rate their agreement with certain statements that relate to smartphone addiction. The collected data would then provide insight regarding how widespread the issue may be – in other words, it would describe the situation.
The key defining attribute of this type of research design is that it purely describes the situation . In other words, descriptive research design does not explore potential relationships between different variables or the causes that may underlie those relationships. Therefore, descriptive research is useful for generating insight into a research problem by describing its characteristics . By doing so, it can provide valuable insights and is often used as a precursor to other research design types.
Correlational Research Design
Correlational design is a popular choice for researchers aiming to identify and measure the relationship between two or more variables without manipulating them . In other words, this type of research design is useful when you want to know whether a change in one thing tends to be accompanied by a change in another thing.
For example, if you wanted to explore the relationship between exercise frequency and overall health, you could use a correlational design to help you achieve this. In this case, you might gather data on participants’ exercise habits, as well as records of their health indicators like blood pressure, heart rate, or body mass index. Thereafter, you’d use a statistical test to assess whether there’s a relationship between the two variables (exercise frequency and health).
As you can see, correlational research design is useful when you want to explore potential relationships between variables that cannot be manipulated or controlled for ethical, practical, or logistical reasons. It is particularly helpful in terms of developing predictions , and given that it doesn’t involve the manipulation of variables, it can be implemented at a large scale more easily than experimental designs (which will look at next).
That said, it’s important to keep in mind that correlational research design has limitations – most notably that it cannot be used to establish causality . In other words, correlation does not equal causation . To establish causality, you’ll need to move into the realm of experimental design, coming up next…
Need a helping hand?
Experimental Research Design
Experimental research design is used to determine if there is a causal relationship between two or more variables . With this type of research design, you, as the researcher, manipulate one variable (the independent variable) while controlling others (dependent variables). Doing so allows you to observe the effect of the former on the latter and draw conclusions about potential causality.
For example, if you wanted to measure if/how different types of fertiliser affect plant growth, you could set up several groups of plants, with each group receiving a different type of fertiliser, as well as one with no fertiliser at all. You could then measure how much each plant group grew (on average) over time and compare the results from the different groups to see which fertiliser was most effective.
Overall, experimental research design provides researchers with a powerful way to identify and measure causal relationships (and the direction of causality) between variables. However, developing a rigorous experimental design can be challenging as it’s not always easy to control all the variables in a study. This often results in smaller sample sizes , which can reduce the statistical power and generalisability of the results.
Moreover, experimental research design requires random assignment . This means that the researcher needs to assign participants to different groups or conditions in a way that each participant has an equal chance of being assigned to any group (note that this is not the same as random sampling ). Doing so helps reduce the potential for bias and confounding variables . This need for random assignment can lead to ethics-related issues . For example, withholding a potentially beneficial medical treatment from a control group may be considered unethical in certain situations.
Quasi-Experimental Research Design
Quasi-experimental research design is used when the research aims involve identifying causal relations , but one cannot (or doesn’t want to) randomly assign participants to different groups (for practical or ethical reasons). Instead, with a quasi-experimental research design, the researcher relies on existing groups or pre-existing conditions to form groups for comparison.
For example, if you were studying the effects of a new teaching method on student achievement in a particular school district, you may be unable to randomly assign students to either group and instead have to choose classes or schools that already use different teaching methods. This way, you still achieve separate groups, without having to assign participants to specific groups yourself.
Naturally, quasi-experimental research designs have limitations when compared to experimental designs. Given that participant assignment is not random, it’s more difficult to confidently establish causality between variables, and, as a researcher, you have less control over other variables that may impact findings.
All that said, quasi-experimental designs can still be valuable in research contexts where random assignment is not possible and can often be undertaken on a much larger scale than experimental research, thus increasing the statistical power of the results. What’s important is that you, as the researcher, understand the limitations of the design and conduct your quasi-experiment as rigorously as possible, paying careful attention to any potential confounding variables .
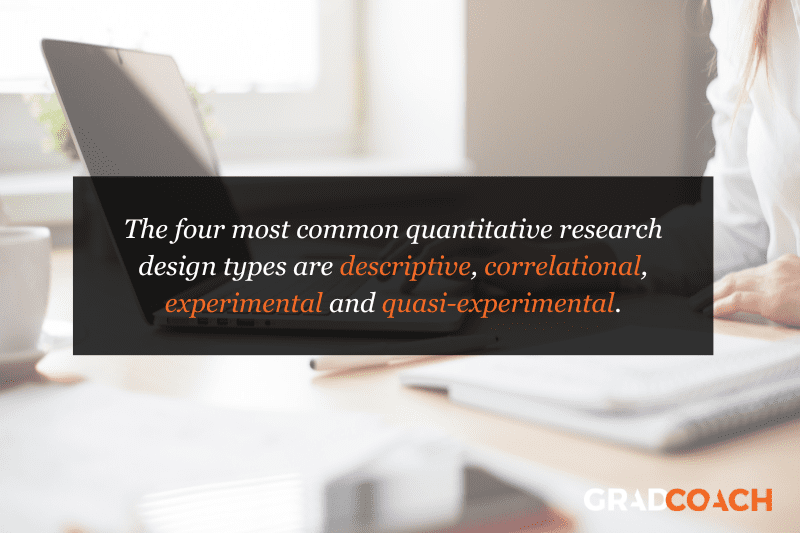
Research Design: Qualitative Studies
There are many different research design types when it comes to qualitative studies, but here we’ll narrow our focus to explore the “Big 4”. Specifically, we’ll look at phenomenological design, grounded theory design, ethnographic design, and case study design.
Phenomenological Research Design
Phenomenological design involves exploring the meaning of lived experiences and how they are perceived by individuals. This type of research design seeks to understand people’s perspectives , emotions, and behaviours in specific situations. Here, the aim for researchers is to uncover the essence of human experience without making any assumptions or imposing preconceived ideas on their subjects.
For example, you could adopt a phenomenological design to study why cancer survivors have such varied perceptions of their lives after overcoming their disease. This could be achieved by interviewing survivors and then analysing the data using a qualitative analysis method such as thematic analysis to identify commonalities and differences.
Phenomenological research design typically involves in-depth interviews or open-ended questionnaires to collect rich, detailed data about participants’ subjective experiences. This richness is one of the key strengths of phenomenological research design but, naturally, it also has limitations. These include potential biases in data collection and interpretation and the lack of generalisability of findings to broader populations.
Grounded Theory Research Design
Grounded theory (also referred to as “GT”) aims to develop theories by continuously and iteratively analysing and comparing data collected from a relatively large number of participants in a study. It takes an inductive (bottom-up) approach, with a focus on letting the data “speak for itself”, without being influenced by preexisting theories or the researcher’s preconceptions.
As an example, let’s assume your research aims involved understanding how people cope with chronic pain from a specific medical condition, with a view to developing a theory around this. In this case, grounded theory design would allow you to explore this concept thoroughly without preconceptions about what coping mechanisms might exist. You may find that some patients prefer cognitive-behavioural therapy (CBT) while others prefer to rely on herbal remedies. Based on multiple, iterative rounds of analysis, you could then develop a theory in this regard, derived directly from the data (as opposed to other preexisting theories and models).
Grounded theory typically involves collecting data through interviews or observations and then analysing it to identify patterns and themes that emerge from the data. These emerging ideas are then validated by collecting more data until a saturation point is reached (i.e., no new information can be squeezed from the data). From that base, a theory can then be developed .
As you can see, grounded theory is ideally suited to studies where the research aims involve theory generation , especially in under-researched areas. Keep in mind though that this type of research design can be quite time-intensive , given the need for multiple rounds of data collection and analysis.
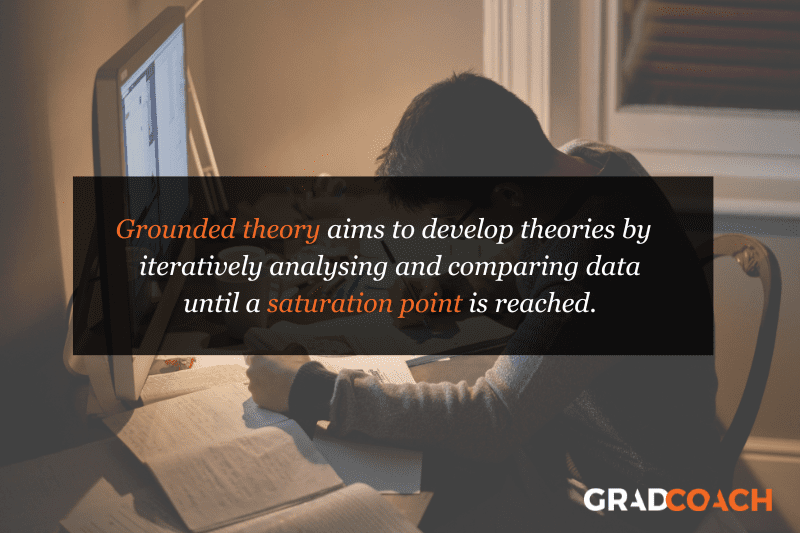
Ethnographic Research Design
Ethnographic design involves observing and studying a culture-sharing group of people in their natural setting to gain insight into their behaviours, beliefs, and values. The focus here is on observing participants in their natural environment (as opposed to a controlled environment). This typically involves the researcher spending an extended period of time with the participants in their environment, carefully observing and taking field notes .
All of this is not to say that ethnographic research design relies purely on observation. On the contrary, this design typically also involves in-depth interviews to explore participants’ views, beliefs, etc. However, unobtrusive observation is a core component of the ethnographic approach.
As an example, an ethnographer may study how different communities celebrate traditional festivals or how individuals from different generations interact with technology differently. This may involve a lengthy period of observation, combined with in-depth interviews to further explore specific areas of interest that emerge as a result of the observations that the researcher has made.
As you can probably imagine, ethnographic research design has the ability to provide rich, contextually embedded insights into the socio-cultural dynamics of human behaviour within a natural, uncontrived setting. Naturally, however, it does come with its own set of challenges, including researcher bias (since the researcher can become quite immersed in the group), participant confidentiality and, predictably, ethical complexities . All of these need to be carefully managed if you choose to adopt this type of research design.
Case Study Design
With case study research design, you, as the researcher, investigate a single individual (or a single group of individuals) to gain an in-depth understanding of their experiences, behaviours or outcomes. Unlike other research designs that are aimed at larger sample sizes, case studies offer a deep dive into the specific circumstances surrounding a person, group of people, event or phenomenon, generally within a bounded setting or context .
As an example, a case study design could be used to explore the factors influencing the success of a specific small business. This would involve diving deeply into the organisation to explore and understand what makes it tick – from marketing to HR to finance. In terms of data collection, this could include interviews with staff and management, review of policy documents and financial statements, surveying customers, etc.
While the above example is focused squarely on one organisation, it’s worth noting that case study research designs can have different variation s, including single-case, multiple-case and longitudinal designs. As you can see in the example, a single-case design involves intensely examining a single entity to understand its unique characteristics and complexities. Conversely, in a multiple-case design , multiple cases are compared and contrasted to identify patterns and commonalities. Lastly, in a longitudinal case design , a single case or multiple cases are studied over an extended period of time to understand how factors develop over time.
As you can see, a case study research design is particularly useful where a deep and contextualised understanding of a specific phenomenon or issue is desired. However, this strength is also its weakness. In other words, you can’t generalise the findings from a case study to the broader population. So, keep this in mind if you’re considering going the case study route.
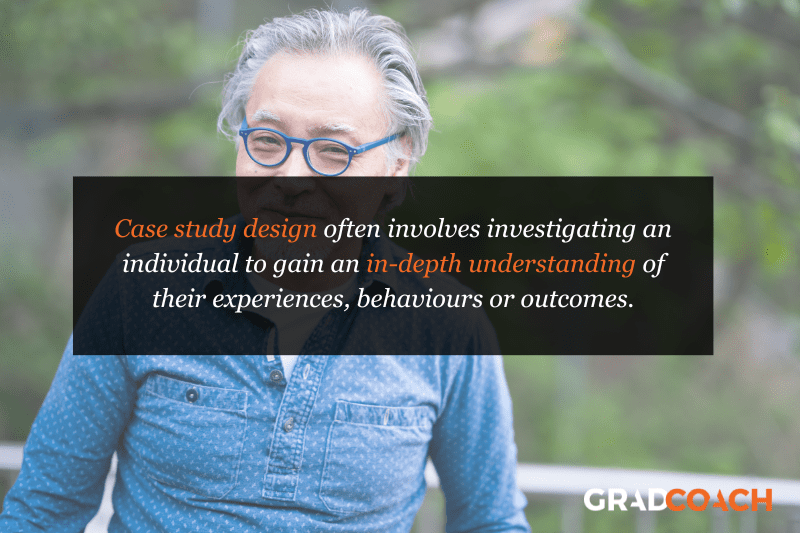
How To Choose A Research Design
Having worked through all of these potential research designs, you’d be forgiven for feeling a little overwhelmed and wondering, “ But how do I decide which research design to use? ”. While we could write an entire post covering that alone, here are a few factors to consider that will help you choose a suitable research design for your study.
Data type: The first determining factor is naturally the type of data you plan to be collecting – i.e., qualitative or quantitative. This may sound obvious, but we have to be clear about this – don’t try to use a quantitative research design on qualitative data (or vice versa)!
Research aim(s) and question(s): As with all methodological decisions, your research aim and research questions will heavily influence your research design. For example, if your research aims involve developing a theory from qualitative data, grounded theory would be a strong option. Similarly, if your research aims involve identifying and measuring relationships between variables, one of the experimental designs would likely be a better option.
Time: It’s essential that you consider any time constraints you have, as this will impact the type of research design you can choose. For example, if you’ve only got a month to complete your project, a lengthy design such as ethnography wouldn’t be a good fit.
Resources: Take into account the resources realistically available to you, as these need to factor into your research design choice. For example, if you require highly specialised lab equipment to execute an experimental design, you need to be sure that you’ll have access to that before you make a decision.
Keep in mind that when it comes to research, it’s important to manage your risks and play as conservatively as possible. If your entire project relies on you achieving a huge sample, having access to niche equipment or holding interviews with very difficult-to-reach participants, you’re creating risks that could kill your project. So, be sure to think through your choices carefully and make sure that you have backup plans for any existential risks. Remember that a relatively simple methodology executed well generally will typically earn better marks than a highly-complex methodology executed poorly.
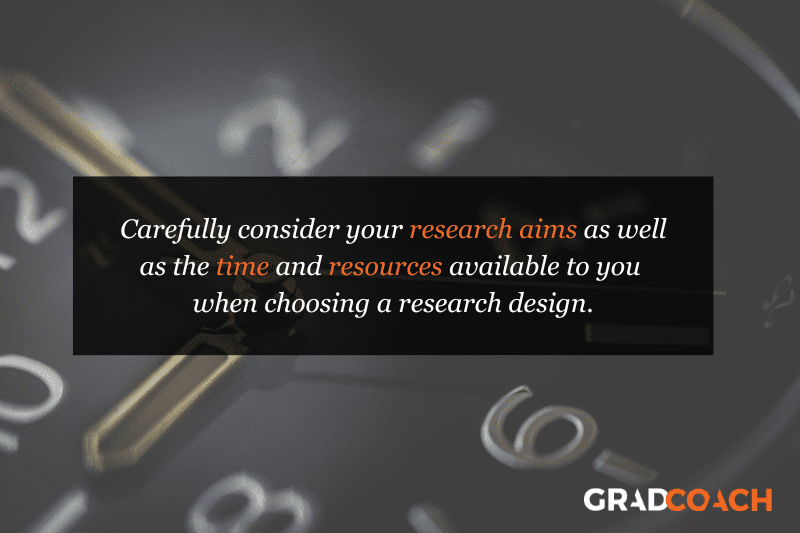
Recap: Key Takeaways
We’ve covered a lot of ground here. Let’s recap by looking at the key takeaways:
- Research design refers to the overall plan, structure or strategy that guides a research project, from its conception to the final analysis of data.
- Research designs for quantitative studies include descriptive , correlational , experimental and quasi-experimenta l designs.
- Research designs for qualitative studies include phenomenological , grounded theory , ethnographic and case study designs.
- When choosing a research design, you need to consider a variety of factors, including the type of data you’ll be working with, your research aims and questions, your time and the resources available to you.
If you need a helping hand with your research design (or any other aspect of your research), check out our private coaching services .
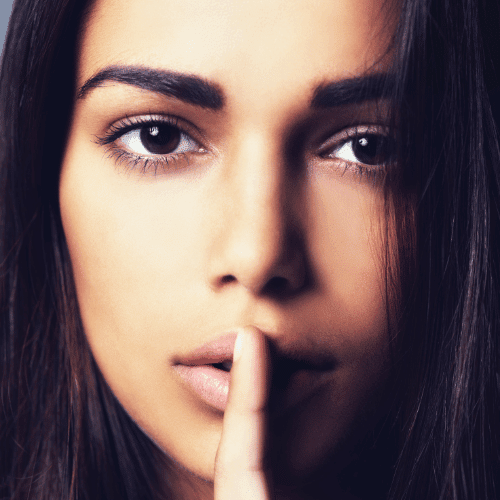
Psst... there’s more!
This post was based on one of our popular Research Bootcamps . If you're working on a research project, you'll definitely want to check this out ...
11 Comments
Is there any blog article explaining more on Case study research design? Is there a Case study write-up template? Thank you.
Thanks this was quite valuable to clarify such an important concept.
Thanks for this simplified explanations. it is quite very helpful.
This was really helpful. thanks
Thank you for your explanation. I think case study research design and the use of secondary data in researches needs to be talked about more in your videos and articles because there a lot of case studies research design tailored projects out there.
Please is there any template for a case study research design whose data type is a secondary data on your repository?
This post is very clear, comprehensive and has been very helpful to me. It has cleared the confusion I had in regard to research design and methodology.
This post is helpful, easy to understand, and deconstructs what a research design is. Thanks
This post is really helpful.
how to cite this page
Thank you very much for the post. It is wonderful and has cleared many worries in my mind regarding research designs. I really appreciate .
how can I put this blog as my reference(APA style) in bibliography part?
Submit a Comment Cancel reply
Your email address will not be published. Required fields are marked *
Save my name, email, and website in this browser for the next time I comment.
- Print Friendly
Understanding Research Study Designs

Table of Contents
In order to find the best possible evidence, it helps to understand the basic designs of research studies. The following basic definitions and examples of clinical research designs follow the “ levels of evidence.”
Case Series and Case Reports
Case control studies, cohort studies, randomized controlled studies, double-blind method, meta-analyses, systematic reviews.
These consist either of collections of reports on the treatment of individual patients with the same condition, or of reports on a single patient.
- Case series/reports are used to illustrate an aspect of a condition, the treatment or the adverse reaction to treatment.
- Example : You have a patient that has a condition that you are unfamiliar with. You would search for case reports that could help you decide on a direction of treatment or to assist on a diagnosis.
- Case series/reports have no control group (one to compare outcomes), so they have no statistical validity.
- The benefits of case series/reports are that they are easy to understand and can be written up in a very short period of time.

Patients who already have a certain condition are compared with people who do not.
- Case control studies are generally designed to estimate the odds (using an odds ratio) of developing the studied condition/disease. They can determine if there is an associational relationship between condition and risk factor
- Example: A study in which colon cancer patients are asked what kinds of food they have eaten in the past and the answers are compared with a selected control group.
- Case control studies are less reliable than either randomized controlled trials or cohort studies.
- A major drawback to case control studies is that one cannot directly obtain absolute risk (i.e. incidence) of a bad outcome.
- The advantages of case control studies are they can be done quickly and are very efficient for conditions/diseases with rare outcomes.

Also called longitudinal studies, involve a case-defined population who presently have a certain exposure and/or receive a particular treatment that are followed over time and compared with another group who are not affected by the exposure under investigation.
- Cohort studies may be either prospective (i.e., exposure factors are identified at the beginning of a study and a defined population is followed into the future), or historical/retrospective (i.e., past medical records for the defined population are used to identify exposure factors).
- Cohort studies are used to establish causation of a disease or to evaluate the outcome/impact of treatment, when randomized controlled clinical trials are not possible.
- Example: One of the more well-know examples of a cohort study is the Framingham Heart Study, which followed generations of residents of Framingham, Massachusetts.
- Cohort studies are not as reliable as randomized controlled studies, since the two groups may differ in ways other than the variable under study.
- Other problems with cohort studies are that they require a large sample size, are inefficient for rare outcomes, and can take long periods of time.

This is a study in which 1) There are two groups, one treatment group and one control group. The treatment group receives the treatment under investigation, and the control group receives either no treatment (placebo) or standard treatment. 2) Patients are randomly assigned to all groups.
- Randomized controlled trials are considered the “gold standard” in medical research. They lend themselves best to answering questions about the effectiveness of different therapies or interventions.
- Randomization helps avoid the bias in choice of patients-to-treatment that a physician might be subject to. It also increases the probability that differences between the groups can be attributed to the treatment(s) under study.
- Having a control group allows for a comparison of treatments – e.g., treatment A produced favorable results 56% of the time versus treatment B in which only 25% of patients had favorable results.
- There are certain types of questions on which randomized controlled studies cannot be done for ethical reasons, for instance, if patients were asked to undertake harmful experiences (like smoking) or denied any treatment beyond a placebo when there are known effective treatments.

A type of randomized controlled clinical trial/study in which neither medical staff/physician nor the patient knows which of several possible treatments/therapies the patient is receiving.
- Example : Studies of treatments that consist essentially of taking pills are very easy to do double blind – the patient takes one of two pills of identical size, shape, and color, and neither the patient nor the physician needs to know which is which.
- A double blind study is the most rigorous clinical research design because, in addition to the randomization of subjects, which reduces the risk of bias, it can eliminate or minimize the placebo effect which is a further challenge to the validity of a study.

Meta-analysis is a systematic, objective way to combine data from many studies, usually from randomized controlled clinical trials, and arrive at a pooled estimate of treatment effectiveness and statistical significance.
- Meta-analysis can also combine data from case/control and cohort studies. The advantage to merging these data is that it increases sample size and allows for analyses that would not otherwise be possible.
- They should not be confused with reviews of the literature or systematic reviews.
- Two problems with meta-analysis are publication bias (studies showing no effect or little effect are often not published and just “filed” away) and the quality of the design of the studies from which data is pulled. This can lead to misleading results when all the data on the subject from “published” literature are summarized.

A systematic review is a comprehensive survey of a topic that takes great care to find all relevant studies of the highest level of evidence, published and unpublished, assess each study, synthesize the findings from individual studies in an unbiased, explicit and reproducible way and present a balanced and impartial summary of the findings with due consideration of any flaws in the evidence. In this way it can be used for the evaluation of either existing or new technologies and practices.
A systematic review is more rigorous than a traditional literature review and attempts to reduce the influence of bias. In order to do this, a systematic review follows a formal process:
- Clearly formulated research question
- Published & unpublished (conferences, company reports, “file drawer reports”, etc.) literature is carefully searched for relevant research
- Identified research is assessed according to an explicit methodology
- Results of the critical assessment of the individual studies are combined
- Final results are placed in context, addressing such issues are quality of the included studies, impact of bias and the applicability of the findings
- The difference between a systematic review and a meta-analysis is that a systematic review looks at the whole picture (qualitative view), while a meta-analysis looks for the specific statistical picture (quantitative view).

R esearch Process in the Health Sciences (35:37 min): Overview of the scientific research process in the health sciences. Follows the seven steps: defining the problem, reviewing the literature, formulating a hypothesis, choosing a research design, collecting data, analyzing the data and interpretation and report writing. Includes a set of additional readings and library resources.
Research Study Designs in the Health Sciences (29:36 min): An overview of research study designs used by health sciences researchers. Covers case reports/case series, case control studies, cohort studies, correlational studies, cross-sectional studies, experimental studies (including randomized control trials), systematic reviews and meta-analysis. Additional readings and library resources are also provided.

Types of Study Design
- 📖 Geeky Medics OSCE Book
- ⚡ Geeky Medics Bundles
- ✨ 1300+ OSCE Stations
- ✅ OSCE Checklist PDF Booklet
- 🧠 UKMLA AKT Question Bank
- 💊 PSA Question Bank
- 💉 Clinical Skills App
- 🗂️ Flashcard Collections | OSCE , Medicine , Surgery , Anatomy
- 💬 SCA Cases for MRCGP
To be the first to know about our latest videos subscribe to our YouTube channel 🙌
Table of Contents
Suggest an improvement
- Hidden Post Title
- Hidden Post URL
- Hidden Post ID
- Type of issue * N/A Fix spelling/grammar issue Add or fix a link Add or fix an image Add more detail Improve the quality of the writing Fix a factual error
- Please provide as much detail as possible * You don't need to tell us which article this feedback relates to, as we automatically capture that information for you.
- Your Email (optional) This allows us to get in touch for more details if required.
- Which organ is responsible for pumping blood around the body? * Enter a five letter word in lowercase
- Phone This field is for validation purposes and should be left unchanged.
Introduction
Study designs are frameworks used in medical research to gather data and explore a specific research question .
Choosing an appropriate study design is one of many essential considerations before conducting research to minimise bias and yield valid results .
This guide provides a summary of study designs commonly used in medical research, their characteristics, advantages and disadvantages.
Case-report and case-series
A case report is a detailed description of a patient’s medical history, diagnosis, treatment, and outcome. A case report typically documents unusual or rare cases or reports new or unexpected clinical findings .
A case series is a similar study that involves a group of patients sharing a similar disease or condition. A case series involves a comprehensive review of medical records for each patient to identify common features or disease patterns. Case series help better understand a disease’s presentation, diagnosis, and treatment.
While a case report focuses on a single patient, a case series involves a group of patients to provide a broader perspective on a specific disease. Both case reports and case series are important tools for understanding rare or unusual diseases .
Advantages of case series and case reports include:
- Able to describe rare or poorly understood conditions or diseases
- Helpful in generating hypotheses and identifying patterns or trends in patient populations
- Can be conducted relatively quickly and at a lower cost compared to other research designs
Disadvantages
Disadvantages of case series and case reports include:
- Prone to selection bias , meaning that the patients included in the series may not be representative of the general population
- Lack a control group, which makes it difficult to conclude the effectiveness of different treatments or interventions
- They are descriptive and cannot establish causality or control for confounding factors
Cross-sectional study
A cross-sectional study aims to measure the prevalence or frequency of a disease in a population at a specific point in time. In other words, it provides a “ snapshot ” of the population at a single moment in time.
Cross-sectional studies are unique from other study designs in that they collect data on the exposure and the outcome of interest from a sample of individuals in the population. This type of data is used to investigate the distribution of health-related conditions and behaviours in different populations, which is especially useful for guiding the development of public health interventions .
Example of a cross-sectional study
A cross-sectional study might investigate the prevalence of hypertension (the outcome) in a sample of adults in a particular region. The researchers would measure blood pressure levels in each participant and gather information on other factors that could influence blood pressure, such as age, sex, weight, and lifestyle habits (exposure).
Advantages of cross-sectional studies include:
- Relatively quick and inexpensive to conduct compared to other study designs, such as cohort or case-control studies
- They can provide a snapshot of the prevalence and distribution of a particular health condition in a population
- They can help to identify patterns and associations between exposure and outcome variables, which can be used to generate hypotheses for further research
Disadvantages of cross-sectional studies include:
- They cannot establish causality , as they do not follow participants over time and cannot determine the temporal sequence between exposure and outcome
- Prone to selection bias , as the sample may not represent the entire population being studied
- They cannot account for confounding variables , which may affect the relationship between the exposure and outcome of interest
Case-control study
A case-control study compares people who have developed a disease of interest ( cases ) with people who have not developed the disease ( controls ) to identify potential risk factors associated with the disease.
Once cases and controls have been identified, researchers then collect information about related risk factors , such as age, sex, lifestyle factors, or environmental exposures, from individuals. By comparing the prevalence of risk factors between the cases and the controls, researchers can determine the association between the risk factors and the disease.
Example of a case-control study
A case-control study design might involve comparing a group of individuals with lung cancer (cases) to a group of individuals without lung cancer (controls) to assess the association between smoking (risk factor) and the development of lung cancer.
Advantages of case-control studies include:
- Useful for studying rare diseases , as they allow researchers to selectively recruit cases with the disease of interest
- Useful for investigating potential risk factors for a disease, as the researchers can collect data on many different factors from both cases and controls
- Can be helpful in situations where it is not ethical or practical to manipulate exposure levels or randomise study participants
Disadvantages of case-control studies include:
- Prone to selection bias , as the controls may not be representative of the general population or may have different underlying risk factors than the cases
- Cannot establish causality , as they can only identify associations between factors and disease
- May be limited by the availability of suitable controls , as finding appropriate controls who have similar characteristics to the cases can be challenging
Cohort study
A cohort study follows a group of individuals (a cohort) over time to investigate the relationship between an exposure or risk factor and a particular outcome or health condition. Cohort studies can be further classified into prospective or retrospective cohort studies.
Prospective cohort study
A prospective cohort study is a study in which the researchers select a group of individuals who do not have a particular disease or outcome of interest at the start of the study.
They then follow this cohort over time to track the number of patients who develop the outcome . Before the start of the study, information on exposure(s) of interest may also be collected.
Example of a prospective cohort study
A prospective cohort study might follow a group of individuals who have never smoked and measure their exposure to tobacco smoke over time to investigate the relationship between smoking and lung cancer .
Retrospective cohort study
In contrast, a retrospective cohort study is a study in which the researchers select a group of individuals who have already been exposed to something (e.g. smoking) and look back in time (for example, through patient charts) to see if they developed the outcome (e.g. lung cancer ).
The key difference in retrospective cohort studies is that data on exposure and outcome are collected after the outcome has occurred.
Example of a retrospective cohort study
A retrospective cohort study might look at the medical records of smokers and see if they developed a particular adverse event such as lung cancer.
Advantages of cohort studies include:
- Generally considered to be the most appropriate study design for investigating the temporal relationship between exposure and outcome
- Can provide estimates of incidence and relative risk , which are useful for quantifying the strength of the association between exposure and outcome
- Can be used to investigate multiple outcomes or endpoints associated with a particular exposure, which can help to identify unexpected effects or outcomes
Disadvantages of cohort studies include:
- Can be expensive and time-consuming to conduct, particularly for long-term follow-up
- May suffer from selection bias , as the sample may not be representative of the entire population being studied
- May suffer from attrition bias , as participants may drop out or be lost to follow-up over time
Meta-analysis
A meta-analysis is a type of study that involves extracting outcome data from all relevant studies in the literature and combining the results of multiple studies to produce an overall estimate of the effect size of an intervention or exposure.
Meta-analysis is often conducted alongside a systematic review and can be considered a study of studies . By doing this, researchers provide a more comprehensive and reliable estimate of the overall effect size and their confidence interval (a measure of precision).
Meta-analyses can be conducted for a wide range of research questions , including evaluating the effectiveness of medical interventions, identifying risk factors for disease, or assessing the accuracy of diagnostic tests. They are particularly useful when the results of individual studies are inconsistent or when the sample sizes of individual studies are small, as a meta-analysis can provide a more precise estimate of the true effect size.
When conducting a meta-analysis, researchers must carefully assess the risk of bias in each study to enhance the validity of the meta-analysis. Many aspects of research studies are prone to bias , such as the methodology and the reporting of results. Where studies exhibit a high risk of bias, authors may opt to exclude the study from the analysis or perform a subgroup or sensitivity analysis.
Advantages of a meta-analysis include:
- Combine the results of multiple studies, resulting in a larger sample size and increased statistical power, to provide a more comprehensive and precise estimate of the effect size of an intervention or outcome
- Can help to identify sources of heterogeneity or variability in the results of individual studies by exploring the influence of different study characteristics or subgroups
- Can help to resolve conflicting results or controversies in the literature by providing a more robust estimate of the effect size
Disadvantages of a meta-analysis include:
- Susceptible to publication bias , where studies with statistically significant or positive results are more likely to be published than studies with nonsignificant or negative results. This bias can lead to an overestimation of the treatment effect in a meta-analysis
- May not be appropriate if the studies included are too heterogeneous , as this can make it difficult to draw meaningful conclusions from the pooled results
- Depend on the quality and completeness of the data available from the individual studies and may be limited by the lack of data on certain outcomes or subgroups
Ecological study
An ecological study assesses the relationship between outcome and exposure at a population level or among groups of people rather than studying individuals directly.
The main goal of an ecological study is to observe and analyse patterns or trends at the population level and to identify potential associations or correlations between environmental factors or exposures and health outcomes.
Ecological studies focus on collecting data on population health outcomes , such as disease or mortality rates, and environmental factors or exposures, such as air pollution, temperature, or socioeconomic status.
Example of an ecological study
An ecological study might be used when comparing smoking rates and lung cancer incidence across different countries.
Advantages of an ecological study include:
- Provide insights into how social, economic, and environmental factors may impact health outcomes in real-world settings , which can inform public health policies and interventions
- Cost-effective and efficient, often using existing data or readily available data, such as data from national or regional databases
Disadvantages of an ecological study include:
- Ecological fallacy occurs when conclusions about individual-level associations are drawn from population-level differences
- Ecological studies rely on population-level (i.e. aggregate) rather than individual-level data; they cannot establish causal relationships between exposures and outcomes, as the studies do not account for differences or confounders at the individual level
Randomised controlled trial
A randomised controlled trial (RCT) is an important study design commonly used in medical research to determine the effectiveness of a treatment or intervention . It is considered the gold standard in research design because it allows researchers to draw cause-and-effect conclusions about the effects of an intervention.
In an RCT, participants are randomly assigned to two or more groups. One group receives the intervention being tested, such as a new drug or a specific medical procedure. In contrast, the other group is a control group and receives either no intervention or a placebo .
Randomisation ensures that each participant has an equal chance of being assigned to either group, thereby minimising selection bias . To reduce bias, an RCT often uses a technique called blinding , in which study participants, researchers, or analysts are kept unaware of participant assignment during the study. The participants are then followed over time, and outcome measures are collected and compared to determine if there is any statistical difference between the intervention and control groups.
Example of a randomised controlled trial
An RCT might be employed to evaluate the effectiveness of a new smoking cessation program in helping individuals quit smoking compared to the existing standard of care.
Advantages of an RCT include:
- Considered the most reliable study design for establishing causal relationships between interventions and outcomes and determining the effectiveness of interventions
- Randomisation of participants to intervention and control groups ensures that the groups are similar at the outset, reducing the risk of selection bias and enhancing internal validity
- Using a control group allows researchers to compare with the group that received the intervention while controlling for confounding factors
Disadvantages of an RCT include:
- Can raise ethical concerns ; for example, it may be considered unethical to withhold an intervention from a control group, especially if the intervention is known to be effective
- Can be expensive and time-consuming to conduct, requiring resources for participant recruitment, randomisation, data collection, and analysis
- Often have strict inclusion and exclusion criteria , which may limit the generalisability of the findings to broader populations
- May not always be feasible or practical for certain research questions, especially in rare diseases or when studying long-term outcomes
Dr Chris Jefferies
- Yuliya L, Qazi MA (eds.). Toronto Notes 2022. Toronto: Toronto Notes for Medical Students Inc; 2022.
- Le T, Bhushan V, Qui C, Chalise A, Kaparaliotis P, Coleman C, Kallianos K. First Aid for the USMLE Step 1 2023. New York: McGraw-Hill Education; 2023.
- Rothman KJ, Greenland S, Lash T. Modern Epidemiology. 3 rd ed. Philadelphia: Lippincott Williams & Wilkins; 2008.
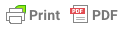
Other pages
- Product Bundles 🎉
- Join the Team 🙌
- Institutional Licence 📚
- OSCE Station Creator Tool 🩺
- Create and Share Flashcards 🗂️
- OSCE Group Chat 💬
- Newsletter 📰
- Advertise With Us
Join the community
Educational resources and simple solutions for your research journey

What is Research Design? Understand Types of Research Design, with Examples
Have you been wondering “ what is research design ?” or “what are some research design examples ?” Are you unsure about the research design elements or which of the different types of research design best suit your study? Don’t worry! In this article, we’ve got you covered!
Table of Contents
What is research design?
Have you been wondering “ what is research design ?” or “what are some research design examples ?” Don’t worry! In this article, we’ve got you covered!
A research design is the plan or framework used to conduct a research study. It involves outlining the overall approach and methods that will be used to collect and analyze data in order to answer research questions or test hypotheses. A well-designed research study should have a clear and well-defined research question, a detailed plan for collecting data, and a method for analyzing and interpreting the results. A well-thought-out research design addresses all these features.
Research design elements
Research design elements include the following:
- Clear purpose: The research question or hypothesis must be clearly defined and focused.
- Sampling: This includes decisions about sample size, sampling method, and criteria for inclusion or exclusion. The approach varies for different research design types .
- Data collection: This research design element involves the process of gathering data or information from the study participants or sources. It includes decisions about what data to collect, how to collect it, and the tools or instruments that will be used.
- Data analysis: All research design types require analysis and interpretation of the data collected. This research design element includes decisions about the statistical tests or methods that will be used to analyze the data, as well as any potential confounding variables or biases that may need to be addressed.
- Type of research methodology: This includes decisions about the overall approach for the study.
- Time frame: An important research design element is the time frame, which includes decisions about the duration of the study, the timeline for data collection and analysis, and follow-up periods.
- Ethical considerations: The research design must include decisions about ethical considerations such as informed consent, confidentiality, and participant protection.
- Resources: A good research design takes into account decisions about the budget, staffing, and other resources needed to carry out the study.
The elements of research design should be carefully planned and executed to ensure the validity and reliability of the study findings. Let’s go deeper into the concepts of research design .

Characteristics of research design
Some basic characteristics of research design are common to different research design types . These characteristics of research design are as follows:
- Neutrality : Right from the study assumptions to setting up the study, a neutral stance must be maintained, free of pre-conceived notions. The researcher’s expectations or beliefs should not color the findings or interpretation of the findings. Accordingly, a good research design should address potential sources of bias and confounding factors to be able to yield unbiased and neutral results.
- Reliability : Reliability is one of the characteristics of research design that refers to consistency in measurement over repeated measures and fewer random errors. A reliable research design must allow for results to be consistent, with few errors due to chance.
- Validity : Validity refers to the minimization of nonrandom (systematic) errors. A good research design must employ measurement tools that ensure validity of the results.
- Generalizability: The outcome of the research design should be applicable to a larger population and not just a small sample . A generalized method means the study can be conducted on any part of a population with similar accuracy.
- Flexibility: A research design should allow for changes to be made to the research plan as needed, based on the data collected and the outcomes of the study
A well-planned research design is critical for conducting a scientifically rigorous study that will generate neutral, reliable, valid, and generalizable results. At the same time, it should allow some level of flexibility.
Different types of research design
A research design is essential to systematically investigate, understand, and interpret phenomena of interest. Let’s look at different types of research design and research design examples .
Broadly, research design types can be divided into qualitative and quantitative research.
Qualitative research is subjective and exploratory. It determines relationships between collected data and observations. It is usually carried out through interviews with open-ended questions, observations that are described in words, etc.
Quantitative research is objective and employs statistical approaches. It establishes the cause-and-effect relationship among variables using different statistical and computational methods. This type of research is usually done using surveys and experiments.
Qualitative research vs. Quantitative research
Deals with subjective aspects, e.g., experiences, beliefs, perspectives, and concepts. | Measures different types of variables and describes frequencies, averages, correlations, etc. |
Deals with non-numerical data, such as words, images, and observations. | Tests hypotheses about relationships between variables. Results are presented numerically and statistically. |
In qualitative research design, data are collected via direct observations, interviews, focus groups, and naturally occurring data. Methods for conducting qualitative research are grounded theory, thematic analysis, and discourse analysis.
| Quantitative research design is empirical. Data collection methods involved are experiments, surveys, and observations expressed in numbers. The research design categories under this are descriptive, experimental, correlational, diagnostic, and explanatory. |
Data analysis involves interpretation and narrative analysis. | Data analysis involves statistical analysis and hypothesis testing. |
The reasoning used to synthesize data is inductive.
| The reasoning used to synthesize data is deductive.
|
Typically used in fields such as sociology, linguistics, and anthropology. | Typically used in fields such as economics, ecology, statistics, and medicine. |
Example: Focus group discussions with women farmers about climate change perception.
| Example: Testing the effectiveness of a new treatment for insomnia. |
Qualitative research design types and qualitative research design examples
The following will familiarize you with the research design categories in qualitative research:
- Grounded theory: This design is used to investigate research questions that have not previously been studied in depth. Also referred to as exploratory design , it creates sequential guidelines, offers strategies for inquiry, and makes data collection and analysis more efficient in qualitative research.
Example: A researcher wants to study how people adopt a certain app. The researcher collects data through interviews and then analyzes the data to look for patterns. These patterns are used to develop a theory about how people adopt that app.
- Thematic analysis: This design is used to compare the data collected in past research to find similar themes in qualitative research.
Example: A researcher examines an interview transcript to identify common themes, say, topics or patterns emerging repeatedly.
- Discourse analysis : This research design deals with language or social contexts used in data gathering in qualitative research.
Example: Identifying ideological frameworks and viewpoints of writers of a series of policies.
Quantitative research design types and quantitative research design examples
Note the following research design categories in quantitative research:
- Descriptive research design : This quantitative research design is applied where the aim is to identify characteristics, frequencies, trends, and categories. It may not often begin with a hypothesis. The basis of this research type is a description of an identified variable. This research design type describes the “what,” “when,” “where,” or “how” of phenomena (but not the “why”).
Example: A study on the different income levels of people who use nutritional supplements regularly.
- Correlational research design : Correlation reflects the strength and/or direction of the relationship among variables. The direction of a correlation can be positive or negative. Correlational research design helps researchers establish a relationship between two variables without the researcher controlling any of them.
Example : An example of correlational research design could be studying the correlation between time spent watching crime shows and aggressive behavior in teenagers.
- Diagnostic research design : In diagnostic design, the researcher aims to understand the underlying cause of a specific topic or phenomenon (usually an area of improvement) and find the most effective solution. In simpler terms, a researcher seeks an accurate “diagnosis” of a problem and identifies a solution.
Example : A researcher analyzing customer feedback and reviews to identify areas where an app can be improved.
- Explanatory research design : In explanatory research design , a researcher uses their ideas and thoughts on a topic to explore their theories in more depth. This design is used to explore a phenomenon when limited information is available. It can help increase current understanding of unexplored aspects of a subject. It is thus a kind of “starting point” for future research.
Example : Formulating hypotheses to guide future studies on delaying school start times for better mental health in teenagers.
- Causal research design : This can be considered a type of explanatory research. Causal research design seeks to define a cause and effect in its data. The researcher does not use a randomly chosen control group but naturally or pre-existing groupings. Importantly, the researcher does not manipulate the independent variable.
Example : Comparing school dropout levels and possible bullying events.
- Experimental research design : This research design is used to study causal relationships . One or more independent variables are manipulated, and their effect on one or more dependent variables is measured.
Example: Determining the efficacy of a new vaccine plan for influenza.
Benefits of research design
T here are numerous benefits of research design . These are as follows:
- Clear direction: Among the benefits of research design , the main one is providing direction to the research and guiding the choice of clear objectives, which help the researcher to focus on the specific research questions or hypotheses they want to investigate.
- Control: Through a proper research design , researchers can control variables, identify potential confounding factors, and use randomization to minimize bias and increase the reliability of their findings.
- Replication: Research designs provide the opportunity for replication. This helps to confirm the findings of a study and ensures that the results are not due to chance or other factors. Thus, a well-chosen research design also eliminates bias and errors.
- Validity: A research design ensures the validity of the research, i.e., whether the results truly reflect the phenomenon being investigated.
- Reliability: Benefits of research design also include reducing inaccuracies and ensuring the reliability of the research (i.e., consistency of the research results over time, across different samples, and under different conditions).
- Efficiency: A strong research design helps increase the efficiency of the research process. Researchers can use a variety of designs to investigate their research questions, choose the most appropriate research design for their study, and use statistical analysis to make the most of their data. By effectively describing the data necessary for an adequate test of the hypotheses and explaining how such data will be obtained, research design saves a researcher’s time.
Overall, an appropriately chosen and executed research design helps researchers to conduct high-quality research, draw meaningful conclusions, and contribute to the advancement of knowledge in their field.
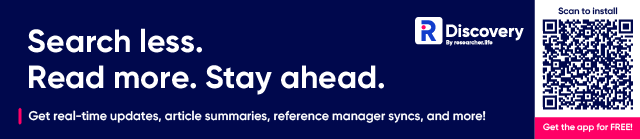
Frequently Asked Questions (FAQ) on Research Design
Q: What are th e main types of research design?
Broadly speaking there are two basic types of research design –
qualitative and quantitative research. Qualitative research is subjective and exploratory; it determines relationships between collected data and observations. It is usually carried out through interviews with open-ended questions, observations that are described in words, etc. Quantitative research , on the other hand, is more objective and employs statistical approaches. It establishes the cause-and-effect relationship among variables using different statistical and computational methods. This type of research design is usually done using surveys and experiments.
Q: How do I choose the appropriate research design for my study?
Choosing the appropriate research design for your study requires careful consideration of various factors. Start by clarifying your research objectives and the type of data you need to collect. Determine whether your study is exploratory, descriptive, or experimental in nature. Consider the availability of resources, time constraints, and the feasibility of implementing the different research designs. Review existing literature to identify similar studies and their research designs, which can serve as a guide. Ultimately, the chosen research design should align with your research questions, provide the necessary data to answer them, and be feasible given your own specific requirements/constraints.
Q: Can research design be modified during the course of a study?
Yes, research design can be modified during the course of a study based on emerging insights, practical constraints, or unforeseen circumstances. Research is an iterative process and, as new data is collected and analyzed, it may become necessary to adjust or refine the research design. However, any modifications should be made judiciously and with careful consideration of their impact on the study’s integrity and validity. It is advisable to document any changes made to the research design, along with a clear rationale for the modifications, in order to maintain transparency and allow for proper interpretation of the results.
Q: How can I ensure the validity and reliability of my research design?
Validity refers to the accuracy and meaningfulness of your study’s findings, while reliability relates to the consistency and stability of the measurements or observations. To enhance validity, carefully define your research variables, use established measurement scales or protocols, and collect data through appropriate methods. Consider conducting a pilot study to identify and address any potential issues before full implementation. To enhance reliability, use standardized procedures, conduct inter-rater or test-retest reliability checks, and employ appropriate statistical techniques for data analysis. It is also essential to document and report your methodology clearly, allowing for replication and scrutiny by other researchers.
Editage All Access is a subscription-based platform that unifies the best AI tools and services designed to speed up, simplify, and streamline every step of a researcher’s journey. The Editage All Access Pack is a one-of-a-kind subscription that unlocks full access to an AI writing assistant, literature recommender, journal finder, scientific illustration tool, and exclusive discounts on professional publication services from Editage.
Based on 22+ years of experience in academia, Editage All Access empowers researchers to put their best research forward and move closer to success. Explore our top AI Tools pack, AI Tools + Publication Services pack, or Build Your Own Plan. Find everything a researcher needs to succeed, all in one place – Get All Access now starting at just $14 a month !
Related Posts

What are the Best Research Funding Sources

Inductive vs. Deductive Research Approach
- USC Libraries
- Research Guides
Organizing Your Social Sciences Research Paper
- Types of Research Designs
- Purpose of Guide
- Design Flaws to Avoid
- Independent and Dependent Variables
- Glossary of Research Terms
- Reading Research Effectively
- Narrowing a Topic Idea
- Broadening a Topic Idea
- Extending the Timeliness of a Topic Idea
- Academic Writing Style
- Applying Critical Thinking
- Choosing a Title
- Making an Outline
- Paragraph Development
- Research Process Video Series
- Executive Summary
- The C.A.R.S. Model
- Background Information
- The Research Problem/Question
- Theoretical Framework
- Citation Tracking
- Content Alert Services
- Evaluating Sources
- Primary Sources
- Secondary Sources
- Tiertiary Sources
- Scholarly vs. Popular Publications
- Qualitative Methods
- Quantitative Methods
- Insiderness
- Using Non-Textual Elements
- Limitations of the Study
- Common Grammar Mistakes
- Writing Concisely
- Avoiding Plagiarism
- Footnotes or Endnotes?
- Further Readings
- Generative AI and Writing
- USC Libraries Tutorials and Other Guides
- Bibliography
Introduction
Before beginning your paper, you need to decide how you plan to design the study .
The research design refers to the overall strategy and analytical approach that you have chosen in order to integrate, in a coherent and logical way, the different components of the study, thus ensuring that the research problem will be thoroughly investigated. It constitutes the blueprint for the collection, measurement, and interpretation of information and data. Note that the research problem determines the type of design you choose, not the other way around!
De Vaus, D. A. Research Design in Social Research . London: SAGE, 2001; Trochim, William M.K. Research Methods Knowledge Base. 2006.
General Structure and Writing Style
The function of a research design is to ensure that the evidence obtained enables you to effectively address the research problem logically and as unambiguously as possible . In social sciences research, obtaining information relevant to the research problem generally entails specifying the type of evidence needed to test the underlying assumptions of a theory, to evaluate a program, or to accurately describe and assess meaning related to an observable phenomenon.
With this in mind, a common mistake made by researchers is that they begin their investigations before they have thought critically about what information is required to address the research problem. Without attending to these design issues beforehand, the overall research problem will not be adequately addressed and any conclusions drawn will run the risk of being weak and unconvincing. As a consequence, the overall validity of the study will be undermined.
The length and complexity of describing the research design in your paper can vary considerably, but any well-developed description will achieve the following :
- Identify the research problem clearly and justify its selection, particularly in relation to any valid alternative designs that could have been used,
- Review and synthesize previously published literature associated with the research problem,
- Clearly and explicitly specify hypotheses [i.e., research questions] central to the problem,
- Effectively describe the information and/or data which will be necessary for an adequate testing of the hypotheses and explain how such information and/or data will be obtained, and
- Describe the methods of analysis to be applied to the data in determining whether or not the hypotheses are true or false.
The research design is usually incorporated into the introduction of your paper . You can obtain an overall sense of what to do by reviewing studies that have utilized the same research design [e.g., using a case study approach]. This can help you develop an outline to follow for your own paper.
NOTE: Use the SAGE Research Methods Online and Cases and the SAGE Research Methods Videos databases to search for scholarly resources on how to apply specific research designs and methods . The Research Methods Online database contains links to more than 175,000 pages of SAGE publisher's book, journal, and reference content on quantitative, qualitative, and mixed research methodologies. Also included is a collection of case studies of social research projects that can be used to help you better understand abstract or complex methodological concepts. The Research Methods Videos database contains hours of tutorials, interviews, video case studies, and mini-documentaries covering the entire research process.
Creswell, John W. and J. David Creswell. Research Design: Qualitative, Quantitative, and Mixed Methods Approaches . 5th edition. Thousand Oaks, CA: Sage, 2018; De Vaus, D. A. Research Design in Social Research . London: SAGE, 2001; Gorard, Stephen. Research Design: Creating Robust Approaches for the Social Sciences . Thousand Oaks, CA: Sage, 2013; Leedy, Paul D. and Jeanne Ellis Ormrod. Practical Research: Planning and Design . Tenth edition. Boston, MA: Pearson, 2013; Vogt, W. Paul, Dianna C. Gardner, and Lynne M. Haeffele. When to Use What Research Design . New York: Guilford, 2012.
Action Research Design
Definition and Purpose
The essentials of action research design follow a characteristic cycle whereby initially an exploratory stance is adopted, where an understanding of a problem is developed and plans are made for some form of interventionary strategy. Then the intervention is carried out [the "action" in action research] during which time, pertinent observations are collected in various forms. The new interventional strategies are carried out, and this cyclic process repeats, continuing until a sufficient understanding of [or a valid implementation solution for] the problem is achieved. The protocol is iterative or cyclical in nature and is intended to foster deeper understanding of a given situation, starting with conceptualizing and particularizing the problem and moving through several interventions and evaluations.
What do these studies tell you ?
- This is a collaborative and adaptive research design that lends itself to use in work or community situations.
- Design focuses on pragmatic and solution-driven research outcomes rather than testing theories.
- When practitioners use action research, it has the potential to increase the amount they learn consciously from their experience; the action research cycle can be regarded as a learning cycle.
- Action research studies often have direct and obvious relevance to improving practice and advocating for change.
- There are no hidden controls or preemption of direction by the researcher.
What these studies don't tell you ?
- It is harder to do than conducting conventional research because the researcher takes on responsibilities of advocating for change as well as for researching the topic.
- Action research is much harder to write up because it is less likely that you can use a standard format to report your findings effectively [i.e., data is often in the form of stories or observation].
- Personal over-involvement of the researcher may bias research results.
- The cyclic nature of action research to achieve its twin outcomes of action [e.g. change] and research [e.g. understanding] is time-consuming and complex to conduct.
- Advocating for change usually requires buy-in from study participants.
Coghlan, David and Mary Brydon-Miller. The Sage Encyclopedia of Action Research . Thousand Oaks, CA: Sage, 2014; Efron, Sara Efrat and Ruth Ravid. Action Research in Education: A Practical Guide . New York: Guilford, 2013; Gall, Meredith. Educational Research: An Introduction . Chapter 18, Action Research. 8th ed. Boston, MA: Pearson/Allyn and Bacon, 2007; Gorard, Stephen. Research Design: Creating Robust Approaches for the Social Sciences . Thousand Oaks, CA: Sage, 2013; Kemmis, Stephen and Robin McTaggart. “Participatory Action Research.” In Handbook of Qualitative Research . Norman Denzin and Yvonna S. Lincoln, eds. 2nd ed. (Thousand Oaks, CA: SAGE, 2000), pp. 567-605; McNiff, Jean. Writing and Doing Action Research . London: Sage, 2014; Reason, Peter and Hilary Bradbury. Handbook of Action Research: Participative Inquiry and Practice . Thousand Oaks, CA: SAGE, 2001.
Case Study Design
A case study is an in-depth study of a particular research problem rather than a sweeping statistical survey or comprehensive comparative inquiry. It is often used to narrow down a very broad field of research into one or a few easily researchable examples. The case study research design is also useful for testing whether a specific theory and model actually applies to phenomena in the real world. It is a useful design when not much is known about an issue or phenomenon.
- Approach excels at bringing us to an understanding of a complex issue through detailed contextual analysis of a limited number of events or conditions and their relationships.
- A researcher using a case study design can apply a variety of methodologies and rely on a variety of sources to investigate a research problem.
- Design can extend experience or add strength to what is already known through previous research.
- Social scientists, in particular, make wide use of this research design to examine contemporary real-life situations and provide the basis for the application of concepts and theories and the extension of methodologies.
- The design can provide detailed descriptions of specific and rare cases.
- A single or small number of cases offers little basis for establishing reliability or to generalize the findings to a wider population of people, places, or things.
- Intense exposure to the study of a case may bias a researcher's interpretation of the findings.
- Design does not facilitate assessment of cause and effect relationships.
- Vital information may be missing, making the case hard to interpret.
- The case may not be representative or typical of the larger problem being investigated.
- If the criteria for selecting a case is because it represents a very unusual or unique phenomenon or problem for study, then your interpretation of the findings can only apply to that particular case.
Case Studies. Writing@CSU. Colorado State University; Anastas, Jeane W. Research Design for Social Work and the Human Services . Chapter 4, Flexible Methods: Case Study Design. 2nd ed. New York: Columbia University Press, 1999; Gerring, John. “What Is a Case Study and What Is It Good for?” American Political Science Review 98 (May 2004): 341-354; Greenhalgh, Trisha, editor. Case Study Evaluation: Past, Present and Future Challenges . Bingley, UK: Emerald Group Publishing, 2015; Mills, Albert J. , Gabrielle Durepos, and Eiden Wiebe, editors. Encyclopedia of Case Study Research . Thousand Oaks, CA: SAGE Publications, 2010; Stake, Robert E. The Art of Case Study Research . Thousand Oaks, CA: SAGE, 1995; Yin, Robert K. Case Study Research: Design and Theory . Applied Social Research Methods Series, no. 5. 3rd ed. Thousand Oaks, CA: SAGE, 2003.
Causal Design
Causality studies may be thought of as understanding a phenomenon in terms of conditional statements in the form, “If X, then Y.” This type of research is used to measure what impact a specific change will have on existing norms and assumptions. Most social scientists seek causal explanations that reflect tests of hypotheses. Causal effect (nomothetic perspective) occurs when variation in one phenomenon, an independent variable, leads to or results, on average, in variation in another phenomenon, the dependent variable.
Conditions necessary for determining causality:
- Empirical association -- a valid conclusion is based on finding an association between the independent variable and the dependent variable.
- Appropriate time order -- to conclude that causation was involved, one must see that cases were exposed to variation in the independent variable before variation in the dependent variable.
- Nonspuriousness -- a relationship between two variables that is not due to variation in a third variable.
- Causality research designs assist researchers in understanding why the world works the way it does through the process of proving a causal link between variables and by the process of eliminating other possibilities.
- Replication is possible.
- There is greater confidence the study has internal validity due to the systematic subject selection and equity of groups being compared.
- Not all relationships are causal! The possibility always exists that, by sheer coincidence, two unrelated events appear to be related [e.g., Punxatawney Phil could accurately predict the duration of Winter for five consecutive years but, the fact remains, he's just a big, furry rodent].
- Conclusions about causal relationships are difficult to determine due to a variety of extraneous and confounding variables that exist in a social environment. This means causality can only be inferred, never proven.
- If two variables are correlated, the cause must come before the effect. However, even though two variables might be causally related, it can sometimes be difficult to determine which variable comes first and, therefore, to establish which variable is the actual cause and which is the actual effect.
Beach, Derek and Rasmus Brun Pedersen. Causal Case Study Methods: Foundations and Guidelines for Comparing, Matching, and Tracing . Ann Arbor, MI: University of Michigan Press, 2016; Bachman, Ronet. The Practice of Research in Criminology and Criminal Justice . Chapter 5, Causation and Research Designs. 3rd ed. Thousand Oaks, CA: Pine Forge Press, 2007; Brewer, Ernest W. and Jennifer Kubn. “Causal-Comparative Design.” In Encyclopedia of Research Design . Neil J. Salkind, editor. (Thousand Oaks, CA: Sage, 2010), pp. 125-132; Causal Research Design: Experimentation. Anonymous SlideShare Presentation; Gall, Meredith. Educational Research: An Introduction . Chapter 11, Nonexperimental Research: Correlational Designs. 8th ed. Boston, MA: Pearson/Allyn and Bacon, 2007; Trochim, William M.K. Research Methods Knowledge Base. 2006.
Cohort Design
Often used in the medical sciences, but also found in the applied social sciences, a cohort study generally refers to a study conducted over a period of time involving members of a population which the subject or representative member comes from, and who are united by some commonality or similarity. Using a quantitative framework, a cohort study makes note of statistical occurrence within a specialized subgroup, united by same or similar characteristics that are relevant to the research problem being investigated, rather than studying statistical occurrence within the general population. Using a qualitative framework, cohort studies generally gather data using methods of observation. Cohorts can be either "open" or "closed."
- Open Cohort Studies [dynamic populations, such as the population of Los Angeles] involve a population that is defined just by the state of being a part of the study in question (and being monitored for the outcome). Date of entry and exit from the study is individually defined, therefore, the size of the study population is not constant. In open cohort studies, researchers can only calculate rate based data, such as, incidence rates and variants thereof.
- Closed Cohort Studies [static populations, such as patients entered into a clinical trial] involve participants who enter into the study at one defining point in time and where it is presumed that no new participants can enter the cohort. Given this, the number of study participants remains constant (or can only decrease).
- The use of cohorts is often mandatory because a randomized control study may be unethical. For example, you cannot deliberately expose people to asbestos, you can only study its effects on those who have already been exposed. Research that measures risk factors often relies upon cohort designs.
- Because cohort studies measure potential causes before the outcome has occurred, they can demonstrate that these “causes” preceded the outcome, thereby avoiding the debate as to which is the cause and which is the effect.
- Cohort analysis is highly flexible and can provide insight into effects over time and related to a variety of different types of changes [e.g., social, cultural, political, economic, etc.].
- Either original data or secondary data can be used in this design.
- In cases where a comparative analysis of two cohorts is made [e.g., studying the effects of one group exposed to asbestos and one that has not], a researcher cannot control for all other factors that might differ between the two groups. These factors are known as confounding variables.
- Cohort studies can end up taking a long time to complete if the researcher must wait for the conditions of interest to develop within the group. This also increases the chance that key variables change during the course of the study, potentially impacting the validity of the findings.
- Due to the lack of randominization in the cohort design, its external validity is lower than that of study designs where the researcher randomly assigns participants.
Healy P, Devane D. “Methodological Considerations in Cohort Study Designs.” Nurse Researcher 18 (2011): 32-36; Glenn, Norval D, editor. Cohort Analysis . 2nd edition. Thousand Oaks, CA: Sage, 2005; Levin, Kate Ann. Study Design IV: Cohort Studies. Evidence-Based Dentistry 7 (2003): 51–52; Payne, Geoff. “Cohort Study.” In The SAGE Dictionary of Social Research Methods . Victor Jupp, editor. (Thousand Oaks, CA: Sage, 2006), pp. 31-33; Study Design 101. Himmelfarb Health Sciences Library. George Washington University, November 2011; Cohort Study. Wikipedia.
Cross-Sectional Design
Cross-sectional research designs have three distinctive features: no time dimension; a reliance on existing differences rather than change following intervention; and, groups are selected based on existing differences rather than random allocation. The cross-sectional design can only measure differences between or from among a variety of people, subjects, or phenomena rather than a process of change. As such, researchers using this design can only employ a relatively passive approach to making causal inferences based on findings.
- Cross-sectional studies provide a clear 'snapshot' of the outcome and the characteristics associated with it, at a specific point in time.
- Unlike an experimental design, where there is an active intervention by the researcher to produce and measure change or to create differences, cross-sectional designs focus on studying and drawing inferences from existing differences between people, subjects, or phenomena.
- Entails collecting data at and concerning one point in time. While longitudinal studies involve taking multiple measures over an extended period of time, cross-sectional research is focused on finding relationships between variables at one moment in time.
- Groups identified for study are purposely selected based upon existing differences in the sample rather than seeking random sampling.
- Cross-section studies are capable of using data from a large number of subjects and, unlike observational studies, is not geographically bound.
- Can estimate prevalence of an outcome of interest because the sample is usually taken from the whole population.
- Because cross-sectional designs generally use survey techniques to gather data, they are relatively inexpensive and take up little time to conduct.
- Finding people, subjects, or phenomena to study that are very similar except in one specific variable can be difficult.
- Results are static and time bound and, therefore, give no indication of a sequence of events or reveal historical or temporal contexts.
- Studies cannot be utilized to establish cause and effect relationships.
- This design only provides a snapshot of analysis so there is always the possibility that a study could have differing results if another time-frame had been chosen.
- There is no follow up to the findings.
Bethlehem, Jelke. "7: Cross-sectional Research." In Research Methodology in the Social, Behavioural and Life Sciences . Herman J Adèr and Gideon J Mellenbergh, editors. (London, England: Sage, 1999), pp. 110-43; Bourque, Linda B. “Cross-Sectional Design.” In The SAGE Encyclopedia of Social Science Research Methods . Michael S. Lewis-Beck, Alan Bryman, and Tim Futing Liao. (Thousand Oaks, CA: 2004), pp. 230-231; Hall, John. “Cross-Sectional Survey Design.” In Encyclopedia of Survey Research Methods . Paul J. Lavrakas, ed. (Thousand Oaks, CA: Sage, 2008), pp. 173-174; Helen Barratt, Maria Kirwan. Cross-Sectional Studies: Design Application, Strengths and Weaknesses of Cross-Sectional Studies. Healthknowledge, 2009. Cross-Sectional Study. Wikipedia.
Descriptive Design
Descriptive research designs help provide answers to the questions of who, what, when, where, and how associated with a particular research problem; a descriptive study cannot conclusively ascertain answers to why. Descriptive research is used to obtain information concerning the current status of the phenomena and to describe "what exists" with respect to variables or conditions in a situation.
- The subject is being observed in a completely natural and unchanged natural environment. True experiments, whilst giving analyzable data, often adversely influence the normal behavior of the subject [a.k.a., the Heisenberg effect whereby measurements of certain systems cannot be made without affecting the systems].
- Descriptive research is often used as a pre-cursor to more quantitative research designs with the general overview giving some valuable pointers as to what variables are worth testing quantitatively.
- If the limitations are understood, they can be a useful tool in developing a more focused study.
- Descriptive studies can yield rich data that lead to important recommendations in practice.
- Appoach collects a large amount of data for detailed analysis.
- The results from a descriptive research cannot be used to discover a definitive answer or to disprove a hypothesis.
- Because descriptive designs often utilize observational methods [as opposed to quantitative methods], the results cannot be replicated.
- The descriptive function of research is heavily dependent on instrumentation for measurement and observation.
Anastas, Jeane W. Research Design for Social Work and the Human Services . Chapter 5, Flexible Methods: Descriptive Research. 2nd ed. New York: Columbia University Press, 1999; Given, Lisa M. "Descriptive Research." In Encyclopedia of Measurement and Statistics . Neil J. Salkind and Kristin Rasmussen, editors. (Thousand Oaks, CA: Sage, 2007), pp. 251-254; McNabb, Connie. Descriptive Research Methodologies. Powerpoint Presentation; Shuttleworth, Martyn. Descriptive Research Design, September 26, 2008; Erickson, G. Scott. "Descriptive Research Design." In New Methods of Market Research and Analysis . (Northampton, MA: Edward Elgar Publishing, 2017), pp. 51-77; Sahin, Sagufta, and Jayanta Mete. "A Brief Study on Descriptive Research: Its Nature and Application in Social Science." International Journal of Research and Analysis in Humanities 1 (2021): 11; K. Swatzell and P. Jennings. “Descriptive Research: The Nuts and Bolts.” Journal of the American Academy of Physician Assistants 20 (2007), pp. 55-56; Kane, E. Doing Your Own Research: Basic Descriptive Research in the Social Sciences and Humanities . London: Marion Boyars, 1985.
Experimental Design
A blueprint of the procedure that enables the researcher to maintain control over all factors that may affect the result of an experiment. In doing this, the researcher attempts to determine or predict what may occur. Experimental research is often used where there is time priority in a causal relationship (cause precedes effect), there is consistency in a causal relationship (a cause will always lead to the same effect), and the magnitude of the correlation is great. The classic experimental design specifies an experimental group and a control group. The independent variable is administered to the experimental group and not to the control group, and both groups are measured on the same dependent variable. Subsequent experimental designs have used more groups and more measurements over longer periods. True experiments must have control, randomization, and manipulation.
- Experimental research allows the researcher to control the situation. In so doing, it allows researchers to answer the question, “What causes something to occur?”
- Permits the researcher to identify cause and effect relationships between variables and to distinguish placebo effects from treatment effects.
- Experimental research designs support the ability to limit alternative explanations and to infer direct causal relationships in the study.
- Approach provides the highest level of evidence for single studies.
- The design is artificial, and results may not generalize well to the real world.
- The artificial settings of experiments may alter the behaviors or responses of participants.
- Experimental designs can be costly if special equipment or facilities are needed.
- Some research problems cannot be studied using an experiment because of ethical or technical reasons.
- Difficult to apply ethnographic and other qualitative methods to experimentally designed studies.
Anastas, Jeane W. Research Design for Social Work and the Human Services . Chapter 7, Flexible Methods: Experimental Research. 2nd ed. New York: Columbia University Press, 1999; Chapter 2: Research Design, Experimental Designs. School of Psychology, University of New England, 2000; Chow, Siu L. "Experimental Design." In Encyclopedia of Research Design . Neil J. Salkind, editor. (Thousand Oaks, CA: Sage, 2010), pp. 448-453; "Experimental Design." In Social Research Methods . Nicholas Walliman, editor. (London, England: Sage, 2006), pp, 101-110; Experimental Research. Research Methods by Dummies. Department of Psychology. California State University, Fresno, 2006; Kirk, Roger E. Experimental Design: Procedures for the Behavioral Sciences . 4th edition. Thousand Oaks, CA: Sage, 2013; Trochim, William M.K. Experimental Design. Research Methods Knowledge Base. 2006; Rasool, Shafqat. Experimental Research. Slideshare presentation.
Exploratory Design
An exploratory design is conducted about a research problem when there are few or no earlier studies to refer to or rely upon to predict an outcome . The focus is on gaining insights and familiarity for later investigation or undertaken when research problems are in a preliminary stage of investigation. Exploratory designs are often used to establish an understanding of how best to proceed in studying an issue or what methodology would effectively apply to gathering information about the issue.
The goals of exploratory research are intended to produce the following possible insights:
- Familiarity with basic details, settings, and concerns.
- Well grounded picture of the situation being developed.
- Generation of new ideas and assumptions.
- Development of tentative theories or hypotheses.
- Determination about whether a study is feasible in the future.
- Issues get refined for more systematic investigation and formulation of new research questions.
- Direction for future research and techniques get developed.
- Design is a useful approach for gaining background information on a particular topic.
- Exploratory research is flexible and can address research questions of all types (what, why, how).
- Provides an opportunity to define new terms and clarify existing concepts.
- Exploratory research is often used to generate formal hypotheses and develop more precise research problems.
- In the policy arena or applied to practice, exploratory studies help establish research priorities and where resources should be allocated.
- Exploratory research generally utilizes small sample sizes and, thus, findings are typically not generalizable to the population at large.
- The exploratory nature of the research inhibits an ability to make definitive conclusions about the findings. They provide insight but not definitive conclusions.
- The research process underpinning exploratory studies is flexible but often unstructured, leading to only tentative results that have limited value to decision-makers.
- Design lacks rigorous standards applied to methods of data gathering and analysis because one of the areas for exploration could be to determine what method or methodologies could best fit the research problem.
Cuthill, Michael. “Exploratory Research: Citizen Participation, Local Government, and Sustainable Development in Australia.” Sustainable Development 10 (2002): 79-89; Streb, Christoph K. "Exploratory Case Study." In Encyclopedia of Case Study Research . Albert J. Mills, Gabrielle Durepos and Eiden Wiebe, editors. (Thousand Oaks, CA: Sage, 2010), pp. 372-374; Taylor, P. J., G. Catalano, and D.R.F. Walker. “Exploratory Analysis of the World City Network.” Urban Studies 39 (December 2002): 2377-2394; Exploratory Research. Wikipedia.
Field Research Design
Sometimes referred to as ethnography or participant observation, designs around field research encompass a variety of interpretative procedures [e.g., observation and interviews] rooted in qualitative approaches to studying people individually or in groups while inhabiting their natural environment as opposed to using survey instruments or other forms of impersonal methods of data gathering. Information acquired from observational research takes the form of “ field notes ” that involves documenting what the researcher actually sees and hears while in the field. Findings do not consist of conclusive statements derived from numbers and statistics because field research involves analysis of words and observations of behavior. Conclusions, therefore, are developed from an interpretation of findings that reveal overriding themes, concepts, and ideas. More information can be found HERE .
- Field research is often necessary to fill gaps in understanding the research problem applied to local conditions or to specific groups of people that cannot be ascertained from existing data.
- The research helps contextualize already known information about a research problem, thereby facilitating ways to assess the origins, scope, and scale of a problem and to gage the causes, consequences, and means to resolve an issue based on deliberate interaction with people in their natural inhabited spaces.
- Enables the researcher to corroborate or confirm data by gathering additional information that supports or refutes findings reported in prior studies of the topic.
- Because the researcher in embedded in the field, they are better able to make observations or ask questions that reflect the specific cultural context of the setting being investigated.
- Observing the local reality offers the opportunity to gain new perspectives or obtain unique data that challenges existing theoretical propositions or long-standing assumptions found in the literature.
What these studies don't tell you
- A field research study requires extensive time and resources to carry out the multiple steps involved with preparing for the gathering of information, including for example, examining background information about the study site, obtaining permission to access the study site, and building trust and rapport with subjects.
- Requires a commitment to staying engaged in the field to ensure that you can adequately document events and behaviors as they unfold.
- The unpredictable nature of fieldwork means that researchers can never fully control the process of data gathering. They must maintain a flexible approach to studying the setting because events and circumstances can change quickly or unexpectedly.
- Findings can be difficult to interpret and verify without access to documents and other source materials that help to enhance the credibility of information obtained from the field [i.e., the act of triangulating the data].
- Linking the research problem to the selection of study participants inhabiting their natural environment is critical. However, this specificity limits the ability to generalize findings to different situations or in other contexts or to infer courses of action applied to other settings or groups of people.
- The reporting of findings must take into account how the researcher themselves may have inadvertently affected respondents and their behaviors.
Historical Design
The purpose of a historical research design is to collect, verify, and synthesize evidence from the past to establish facts that defend or refute a hypothesis. It uses secondary sources and a variety of primary documentary evidence, such as, diaries, official records, reports, archives, and non-textual information [maps, pictures, audio and visual recordings]. The limitation is that the sources must be both authentic and valid.
- The historical research design is unobtrusive; the act of research does not affect the results of the study.
- The historical approach is well suited for trend analysis.
- Historical records can add important contextual background required to more fully understand and interpret a research problem.
- There is often no possibility of researcher-subject interaction that could affect the findings.
- Historical sources can be used over and over to study different research problems or to replicate a previous study.
- The ability to fulfill the aims of your research are directly related to the amount and quality of documentation available to understand the research problem.
- Since historical research relies on data from the past, there is no way to manipulate it to control for contemporary contexts.
- Interpreting historical sources can be very time consuming.
- The sources of historical materials must be archived consistently to ensure access. This may especially challenging for digital or online-only sources.
- Original authors bring their own perspectives and biases to the interpretation of past events and these biases are more difficult to ascertain in historical resources.
- Due to the lack of control over external variables, historical research is very weak with regard to the demands of internal validity.
- It is rare that the entirety of historical documentation needed to fully address a research problem is available for interpretation, therefore, gaps need to be acknowledged.
Howell, Martha C. and Walter Prevenier. From Reliable Sources: An Introduction to Historical Methods . Ithaca, NY: Cornell University Press, 2001; Lundy, Karen Saucier. "Historical Research." In The Sage Encyclopedia of Qualitative Research Methods . Lisa M. Given, editor. (Thousand Oaks, CA: Sage, 2008), pp. 396-400; Marius, Richard. and Melvin E. Page. A Short Guide to Writing about History . 9th edition. Boston, MA: Pearson, 2015; Savitt, Ronald. “Historical Research in Marketing.” Journal of Marketing 44 (Autumn, 1980): 52-58; Gall, Meredith. Educational Research: An Introduction . Chapter 16, Historical Research. 8th ed. Boston, MA: Pearson/Allyn and Bacon, 2007.
Longitudinal Design
A longitudinal study follows the same sample over time and makes repeated observations. For example, with longitudinal surveys, the same group of people is interviewed at regular intervals, enabling researchers to track changes over time and to relate them to variables that might explain why the changes occur. Longitudinal research designs describe patterns of change and help establish the direction and magnitude of causal relationships. Measurements are taken on each variable over two or more distinct time periods. This allows the researcher to measure change in variables over time. It is a type of observational study sometimes referred to as a panel study.
- Longitudinal data facilitate the analysis of the duration of a particular phenomenon.
- Enables survey researchers to get close to the kinds of causal explanations usually attainable only with experiments.
- The design permits the measurement of differences or change in a variable from one period to another [i.e., the description of patterns of change over time].
- Longitudinal studies facilitate the prediction of future outcomes based upon earlier factors.
- The data collection method may change over time.
- Maintaining the integrity of the original sample can be difficult over an extended period of time.
- It can be difficult to show more than one variable at a time.
- This design often needs qualitative research data to explain fluctuations in the results.
- A longitudinal research design assumes present trends will continue unchanged.
- It can take a long period of time to gather results.
- There is a need to have a large sample size and accurate sampling to reach representativness.
Anastas, Jeane W. Research Design for Social Work and the Human Services . Chapter 6, Flexible Methods: Relational and Longitudinal Research. 2nd ed. New York: Columbia University Press, 1999; Forgues, Bernard, and Isabelle Vandangeon-Derumez. "Longitudinal Analyses." In Doing Management Research . Raymond-Alain Thiétart and Samantha Wauchope, editors. (London, England: Sage, 2001), pp. 332-351; Kalaian, Sema A. and Rafa M. Kasim. "Longitudinal Studies." In Encyclopedia of Survey Research Methods . Paul J. Lavrakas, ed. (Thousand Oaks, CA: Sage, 2008), pp. 440-441; Menard, Scott, editor. Longitudinal Research . Thousand Oaks, CA: Sage, 2002; Ployhart, Robert E. and Robert J. Vandenberg. "Longitudinal Research: The Theory, Design, and Analysis of Change.” Journal of Management 36 (January 2010): 94-120; Longitudinal Study. Wikipedia.
Meta-Analysis Design
Meta-analysis is an analytical methodology designed to systematically evaluate and summarize the results from a number of individual studies, thereby, increasing the overall sample size and the ability of the researcher to study effects of interest. The purpose is to not simply summarize existing knowledge, but to develop a new understanding of a research problem using synoptic reasoning. The main objectives of meta-analysis include analyzing differences in the results among studies and increasing the precision by which effects are estimated. A well-designed meta-analysis depends upon strict adherence to the criteria used for selecting studies and the availability of information in each study to properly analyze their findings. Lack of information can severely limit the type of analyzes and conclusions that can be reached. In addition, the more dissimilarity there is in the results among individual studies [heterogeneity], the more difficult it is to justify interpretations that govern a valid synopsis of results. A meta-analysis needs to fulfill the following requirements to ensure the validity of your findings:
- Clearly defined description of objectives, including precise definitions of the variables and outcomes that are being evaluated;
- A well-reasoned and well-documented justification for identification and selection of the studies;
- Assessment and explicit acknowledgment of any researcher bias in the identification and selection of those studies;
- Description and evaluation of the degree of heterogeneity among the sample size of studies reviewed; and,
- Justification of the techniques used to evaluate the studies.
- Can be an effective strategy for determining gaps in the literature.
- Provides a means of reviewing research published about a particular topic over an extended period of time and from a variety of sources.
- Is useful in clarifying what policy or programmatic actions can be justified on the basis of analyzing research results from multiple studies.
- Provides a method for overcoming small sample sizes in individual studies that previously may have had little relationship to each other.
- Can be used to generate new hypotheses or highlight research problems for future studies.
- Small violations in defining the criteria used for content analysis can lead to difficult to interpret and/or meaningless findings.
- A large sample size can yield reliable, but not necessarily valid, results.
- A lack of uniformity regarding, for example, the type of literature reviewed, how methods are applied, and how findings are measured within the sample of studies you are analyzing, can make the process of synthesis difficult to perform.
- Depending on the sample size, the process of reviewing and synthesizing multiple studies can be very time consuming.
Beck, Lewis W. "The Synoptic Method." The Journal of Philosophy 36 (1939): 337-345; Cooper, Harris, Larry V. Hedges, and Jeffrey C. Valentine, eds. The Handbook of Research Synthesis and Meta-Analysis . 2nd edition. New York: Russell Sage Foundation, 2009; Guzzo, Richard A., Susan E. Jackson and Raymond A. Katzell. “Meta-Analysis Analysis.” In Research in Organizational Behavior , Volume 9. (Greenwich, CT: JAI Press, 1987), pp 407-442; Lipsey, Mark W. and David B. Wilson. Practical Meta-Analysis . Thousand Oaks, CA: Sage Publications, 2001; Study Design 101. Meta-Analysis. The Himmelfarb Health Sciences Library, George Washington University; Timulak, Ladislav. “Qualitative Meta-Analysis.” In The SAGE Handbook of Qualitative Data Analysis . Uwe Flick, editor. (Los Angeles, CA: Sage, 2013), pp. 481-495; Walker, Esteban, Adrian V. Hernandez, and Micheal W. Kattan. "Meta-Analysis: It's Strengths and Limitations." Cleveland Clinic Journal of Medicine 75 (June 2008): 431-439.
Mixed-Method Design
- Narrative and non-textual information can add meaning to numeric data, while numeric data can add precision to narrative and non-textual information.
- Can utilize existing data while at the same time generating and testing a grounded theory approach to describe and explain the phenomenon under study.
- A broader, more complex research problem can be investigated because the researcher is not constrained by using only one method.
- The strengths of one method can be used to overcome the inherent weaknesses of another method.
- Can provide stronger, more robust evidence to support a conclusion or set of recommendations.
- May generate new knowledge new insights or uncover hidden insights, patterns, or relationships that a single methodological approach might not reveal.
- Produces more complete knowledge and understanding of the research problem that can be used to increase the generalizability of findings applied to theory or practice.
- A researcher must be proficient in understanding how to apply multiple methods to investigating a research problem as well as be proficient in optimizing how to design a study that coherently melds them together.
- Can increase the likelihood of conflicting results or ambiguous findings that inhibit drawing a valid conclusion or setting forth a recommended course of action [e.g., sample interview responses do not support existing statistical data].
- Because the research design can be very complex, reporting the findings requires a well-organized narrative, clear writing style, and precise word choice.
- Design invites collaboration among experts. However, merging different investigative approaches and writing styles requires more attention to the overall research process than studies conducted using only one methodological paradigm.
- Concurrent merging of quantitative and qualitative research requires greater attention to having adequate sample sizes, using comparable samples, and applying a consistent unit of analysis. For sequential designs where one phase of qualitative research builds on the quantitative phase or vice versa, decisions about what results from the first phase to use in the next phase, the choice of samples and estimating reasonable sample sizes for both phases, and the interpretation of results from both phases can be difficult.
- Due to multiple forms of data being collected and analyzed, this design requires extensive time and resources to carry out the multiple steps involved in data gathering and interpretation.
Burch, Patricia and Carolyn J. Heinrich. Mixed Methods for Policy Research and Program Evaluation . Thousand Oaks, CA: Sage, 2016; Creswell, John w. et al. Best Practices for Mixed Methods Research in the Health Sciences . Bethesda, MD: Office of Behavioral and Social Sciences Research, National Institutes of Health, 2010Creswell, John W. Research Design: Qualitative, Quantitative, and Mixed Methods Approaches . 4th edition. Thousand Oaks, CA: Sage Publications, 2014; Domínguez, Silvia, editor. Mixed Methods Social Networks Research . Cambridge, UK: Cambridge University Press, 2014; Hesse-Biber, Sharlene Nagy. Mixed Methods Research: Merging Theory with Practice . New York: Guilford Press, 2010; Niglas, Katrin. “How the Novice Researcher Can Make Sense of Mixed Methods Designs.” International Journal of Multiple Research Approaches 3 (2009): 34-46; Onwuegbuzie, Anthony J. and Nancy L. Leech. “Linking Research Questions to Mixed Methods Data Analysis Procedures.” The Qualitative Report 11 (September 2006): 474-498; Tashakorri, Abbas and John W. Creswell. “The New Era of Mixed Methods.” Journal of Mixed Methods Research 1 (January 2007): 3-7; Zhanga, Wanqing. “Mixed Methods Application in Health Intervention Research: A Multiple Case Study.” International Journal of Multiple Research Approaches 8 (2014): 24-35 .
Observational Design
This type of research design draws a conclusion by comparing subjects against a control group, in cases where the researcher has no control over the experiment. There are two general types of observational designs. In direct observations, people know that you are watching them. Unobtrusive measures involve any method for studying behavior where individuals do not know they are being observed. An observational study allows a useful insight into a phenomenon and avoids the ethical and practical difficulties of setting up a large and cumbersome research project.
- Observational studies are usually flexible and do not necessarily need to be structured around a hypothesis about what you expect to observe [data is emergent rather than pre-existing].
- The researcher is able to collect in-depth information about a particular behavior.
- Can reveal interrelationships among multifaceted dimensions of group interactions.
- You can generalize your results to real life situations.
- Observational research is useful for discovering what variables may be important before applying other methods like experiments.
- Observation research designs account for the complexity of group behaviors.
- Reliability of data is low because seeing behaviors occur over and over again may be a time consuming task and are difficult to replicate.
- In observational research, findings may only reflect a unique sample population and, thus, cannot be generalized to other groups.
- There can be problems with bias as the researcher may only "see what they want to see."
- There is no possibility to determine "cause and effect" relationships since nothing is manipulated.
- Sources or subjects may not all be equally credible.
- Any group that is knowingly studied is altered to some degree by the presence of the researcher, therefore, potentially skewing any data collected.
Atkinson, Paul and Martyn Hammersley. “Ethnography and Participant Observation.” In Handbook of Qualitative Research . Norman K. Denzin and Yvonna S. Lincoln, eds. (Thousand Oaks, CA: Sage, 1994), pp. 248-261; Observational Research. Research Methods by Dummies. Department of Psychology. California State University, Fresno, 2006; Patton Michael Quinn. Qualitiative Research and Evaluation Methods . Chapter 6, Fieldwork Strategies and Observational Methods. 3rd ed. Thousand Oaks, CA: Sage, 2002; Payne, Geoff and Judy Payne. "Observation." In Key Concepts in Social Research . The SAGE Key Concepts series. (London, England: Sage, 2004), pp. 158-162; Rosenbaum, Paul R. Design of Observational Studies . New York: Springer, 2010;Williams, J. Patrick. "Nonparticipant Observation." In The Sage Encyclopedia of Qualitative Research Methods . Lisa M. Given, editor.(Thousand Oaks, CA: Sage, 2008), pp. 562-563.
Philosophical Design
Understood more as an broad approach to examining a research problem than a methodological design, philosophical analysis and argumentation is intended to challenge deeply embedded, often intractable, assumptions underpinning an area of study. This approach uses the tools of argumentation derived from philosophical traditions, concepts, models, and theories to critically explore and challenge, for example, the relevance of logic and evidence in academic debates, to analyze arguments about fundamental issues, or to discuss the root of existing discourse about a research problem. These overarching tools of analysis can be framed in three ways:
- Ontology -- the study that describes the nature of reality; for example, what is real and what is not, what is fundamental and what is derivative?
- Epistemology -- the study that explores the nature of knowledge; for example, by what means does knowledge and understanding depend upon and how can we be certain of what we know?
- Axiology -- the study of values; for example, what values does an individual or group hold and why? How are values related to interest, desire, will, experience, and means-to-end? And, what is the difference between a matter of fact and a matter of value?
- Can provide a basis for applying ethical decision-making to practice.
- Functions as a means of gaining greater self-understanding and self-knowledge about the purposes of research.
- Brings clarity to general guiding practices and principles of an individual or group.
- Philosophy informs methodology.
- Refine concepts and theories that are invoked in relatively unreflective modes of thought and discourse.
- Beyond methodology, philosophy also informs critical thinking about epistemology and the structure of reality (metaphysics).
- Offers clarity and definition to the practical and theoretical uses of terms, concepts, and ideas.
- Limited application to specific research problems [answering the "So What?" question in social science research].
- Analysis can be abstract, argumentative, and limited in its practical application to real-life issues.
- While a philosophical analysis may render problematic that which was once simple or taken-for-granted, the writing can be dense and subject to unnecessary jargon, overstatement, and/or excessive quotation and documentation.
- There are limitations in the use of metaphor as a vehicle of philosophical analysis.
- There can be analytical difficulties in moving from philosophy to advocacy and between abstract thought and application to the phenomenal world.
Burton, Dawn. "Part I, Philosophy of the Social Sciences." In Research Training for Social Scientists . (London, England: Sage, 2000), pp. 1-5; Chapter 4, Research Methodology and Design. Unisa Institutional Repository (UnisaIR), University of South Africa; Jarvie, Ian C., and Jesús Zamora-Bonilla, editors. The SAGE Handbook of the Philosophy of Social Sciences . London: Sage, 2011; Labaree, Robert V. and Ross Scimeca. “The Philosophical Problem of Truth in Librarianship.” The Library Quarterly 78 (January 2008): 43-70; Maykut, Pamela S. Beginning Qualitative Research: A Philosophic and Practical Guide . Washington, DC: Falmer Press, 1994; McLaughlin, Hugh. "The Philosophy of Social Research." In Understanding Social Work Research . 2nd edition. (London: SAGE Publications Ltd., 2012), pp. 24-47; Stanford Encyclopedia of Philosophy . Metaphysics Research Lab, CSLI, Stanford University, 2013.
Sequential Design
- The researcher has a limitless option when it comes to sample size and the sampling schedule.
- Due to the repetitive nature of this research design, minor changes and adjustments can be done during the initial parts of the study to correct and hone the research method.
- This is a useful design for exploratory studies.
- There is very little effort on the part of the researcher when performing this technique. It is generally not expensive, time consuming, or workforce intensive.
- Because the study is conducted serially, the results of one sample are known before the next sample is taken and analyzed. This provides opportunities for continuous improvement of sampling and methods of analysis.
- The sampling method is not representative of the entire population. The only possibility of approaching representativeness is when the researcher chooses to use a very large sample size significant enough to represent a significant portion of the entire population. In this case, moving on to study a second or more specific sample can be difficult.
- The design cannot be used to create conclusions and interpretations that pertain to an entire population because the sampling technique is not randomized. Generalizability from findings is, therefore, limited.
- Difficult to account for and interpret variation from one sample to another over time, particularly when using qualitative methods of data collection.
Betensky, Rebecca. Harvard University, Course Lecture Note slides; Bovaird, James A. and Kevin A. Kupzyk. "Sequential Design." In Encyclopedia of Research Design . Neil J. Salkind, editor. (Thousand Oaks, CA: Sage, 2010), pp. 1347-1352; Cresswell, John W. Et al. “Advanced Mixed-Methods Research Designs.” In Handbook of Mixed Methods in Social and Behavioral Research . Abbas Tashakkori and Charles Teddle, eds. (Thousand Oaks, CA: Sage, 2003), pp. 209-240; Henry, Gary T. "Sequential Sampling." In The SAGE Encyclopedia of Social Science Research Methods . Michael S. Lewis-Beck, Alan Bryman and Tim Futing Liao, editors. (Thousand Oaks, CA: Sage, 2004), pp. 1027-1028; Nataliya V. Ivankova. “Using Mixed-Methods Sequential Explanatory Design: From Theory to Practice.” Field Methods 18 (February 2006): 3-20; Bovaird, James A. and Kevin A. Kupzyk. “Sequential Design.” In Encyclopedia of Research Design . Neil J. Salkind, ed. Thousand Oaks, CA: Sage, 2010; Sequential Analysis. Wikipedia.
Systematic Review
- A systematic review synthesizes the findings of multiple studies related to each other by incorporating strategies of analysis and interpretation intended to reduce biases and random errors.
- The application of critical exploration, evaluation, and synthesis methods separates insignificant, unsound, or redundant research from the most salient and relevant studies worthy of reflection.
- They can be use to identify, justify, and refine hypotheses, recognize and avoid hidden problems in prior studies, and explain data inconsistencies and conflicts in data.
- Systematic reviews can be used to help policy makers formulate evidence-based guidelines and regulations.
- The use of strict, explicit, and pre-determined methods of synthesis, when applied appropriately, provide reliable estimates about the effects of interventions, evaluations, and effects related to the overarching research problem investigated by each study under review.
- Systematic reviews illuminate where knowledge or thorough understanding of a research problem is lacking and, therefore, can then be used to guide future research.
- The accepted inclusion of unpublished studies [i.e., grey literature] ensures the broadest possible way to analyze and interpret research on a topic.
- Results of the synthesis can be generalized and the findings extrapolated into the general population with more validity than most other types of studies .
- Systematic reviews do not create new knowledge per se; they are a method for synthesizing existing studies about a research problem in order to gain new insights and determine gaps in the literature.
- The way researchers have carried out their investigations [e.g., the period of time covered, number of participants, sources of data analyzed, etc.] can make it difficult to effectively synthesize studies.
- The inclusion of unpublished studies can introduce bias into the review because they may not have undergone a rigorous peer-review process prior to publication. Examples may include conference presentations or proceedings, publications from government agencies, white papers, working papers, and internal documents from organizations, and doctoral dissertations and Master's theses.
Denyer, David and David Tranfield. "Producing a Systematic Review." In The Sage Handbook of Organizational Research Methods . David A. Buchanan and Alan Bryman, editors. ( Thousand Oaks, CA: Sage Publications, 2009), pp. 671-689; Foster, Margaret J. and Sarah T. Jewell, editors. Assembling the Pieces of a Systematic Review: A Guide for Librarians . Lanham, MD: Rowman and Littlefield, 2017; Gough, David, Sandy Oliver, James Thomas, editors. Introduction to Systematic Reviews . 2nd edition. Los Angeles, CA: Sage Publications, 2017; Gopalakrishnan, S. and P. Ganeshkumar. “Systematic Reviews and Meta-analysis: Understanding the Best Evidence in Primary Healthcare.” Journal of Family Medicine and Primary Care 2 (2013): 9-14; Gough, David, James Thomas, and Sandy Oliver. "Clarifying Differences between Review Designs and Methods." Systematic Reviews 1 (2012): 1-9; Khan, Khalid S., Regina Kunz, Jos Kleijnen, and Gerd Antes. “Five Steps to Conducting a Systematic Review.” Journal of the Royal Society of Medicine 96 (2003): 118-121; Mulrow, C. D. “Systematic Reviews: Rationale for Systematic Reviews.” BMJ 309:597 (September 1994); O'Dwyer, Linda C., and Q. Eileen Wafford. "Addressing Challenges with Systematic Review Teams through Effective Communication: A Case Report." Journal of the Medical Library Association 109 (October 2021): 643-647; Okoli, Chitu, and Kira Schabram. "A Guide to Conducting a Systematic Literature Review of Information Systems Research." Sprouts: Working Papers on Information Systems 10 (2010); Siddaway, Andy P., Alex M. Wood, and Larry V. Hedges. "How to Do a Systematic Review: A Best Practice Guide for Conducting and Reporting Narrative Reviews, Meta-analyses, and Meta-syntheses." Annual Review of Psychology 70 (2019): 747-770; Torgerson, Carole J. “Publication Bias: The Achilles’ Heel of Systematic Reviews?” British Journal of Educational Studies 54 (March 2006): 89-102; Torgerson, Carole. Systematic Reviews . New York: Continuum, 2003.
- << Previous: Purpose of Guide
- Next: Design Flaws to Avoid >>
- Last Updated: Aug 13, 2024 12:57 PM
- URL: https://libguides.usc.edu/writingguide
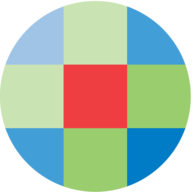
- Get new issue alerts Get alerts
- Submit a Manuscript
Secondary Logo
Journal logo.
Colleague's E-mail is Invalid
Your message has been successfully sent to your colleague.
Save my selection
Study designs
Part 1 – an overview and classification.
Ranganathan, Priya; Aggarwal, Rakesh 1
Department of Anaesthesiology, Tata Memorial Centre, Mumbai, Maharashtra, India
1 Department of Gastroenterology, Sanjay Gandhi Postgraduate Institute of Medical Sciences, Lucknow, Uttar Pradesh, India
Address for correspondence: Dr. Priya Ranganathan, Department of Anaesthesiology, Tata Memorial Centre, Ernest Borges Road, Parel, Mumbai - 400 012, Maharashtra, India. E-mail: [email protected]
This is an open access journal, and articles are distributed under the terms of the Creative Commons Attribution-NonCommercial-ShareAlike 4.0 License, which allows others to remix, tweak, and build upon the work non-commercially, as long as appropriate credit is given and the new creations are licensed under the identical terms.
There are several types of research study designs, each with its inherent strengths and flaws. The study design used to answer a particular research question depends on the nature of the question and the availability of resources. In this article, which is the first part of a series on “study designs,” we provide an overview of research study designs and their classification. The subsequent articles will focus on individual designs.
INTRODUCTION
Research study design is a framework, or the set of methods and procedures used to collect and analyze data on variables specified in a particular research problem.
Research study designs are of many types, each with its advantages and limitations. The type of study design used to answer a particular research question is determined by the nature of question, the goal of research, and the availability of resources. Since the design of a study can affect the validity of its results, it is important to understand the different types of study designs and their strengths and limitations.
There are some terms that are used frequently while classifying study designs which are described in the following sections.
A variable represents a measurable attribute that varies across study units, for example, individual participants in a study, or at times even when measured in an individual person over time. Some examples of variables include age, sex, weight, height, health status, alive/dead, diseased/healthy, annual income, smoking yes/no, and treated/untreated.
Exposure (or intervention) and outcome variables
A large proportion of research studies assess the relationship between two variables. Here, the question is whether one variable is associated with or responsible for change in the value of the other variable. Exposure (or intervention) refers to the risk factor whose effect is being studied. It is also referred to as the independent or the predictor variable. The outcome (or predicted or dependent) variable develops as a consequence of the exposure (or intervention). Typically, the term “exposure” is used when the “causative” variable is naturally determined (as in observational studies – examples include age, sex, smoking, and educational status), and the term “intervention” is preferred where the researcher assigns some or all participants to receive a particular treatment for the purpose of the study (experimental studies – e.g., administration of a drug). If a drug had been started in some individuals but not in the others, before the study started, this counts as exposure, and not as intervention – since the drug was not started specifically for the study.
Observational versus interventional (or experimental) studies
Observational studies are those where the researcher is documenting a naturally occurring relationship between the exposure and the outcome that he/she is studying. The researcher does not do any active intervention in any individual, and the exposure has already been decided naturally or by some other factor. For example, looking at the incidence of lung cancer in smokers versus nonsmokers, or comparing the antenatal dietary habits of mothers with normal and low-birth babies. In these studies, the investigator did not play any role in determining the smoking or dietary habit in individuals.
For an exposure to determine the outcome, it must precede the latter. Any variable that occurs simultaneously with or following the outcome cannot be causative, and hence is not considered as an “exposure.”
Observational studies can be either descriptive (nonanalytical) or analytical (inferential) – this is discussed later in this article.
Interventional studies are experiments where the researcher actively performs an intervention in some or all members of a group of participants. This intervention could take many forms – for example, administration of a drug or vaccine, performance of a diagnostic or therapeutic procedure, and introduction of an educational tool. For example, a study could randomly assign persons to receive aspirin or placebo for a specific duration and assess the effect on the risk of developing cerebrovascular events.
Descriptive versus analytical studies
Descriptive (or nonanalytical) studies, as the name suggests, merely try to describe the data on one or more characteristics of a group of individuals. These do not try to answer questions or establish relationships between variables. Examples of descriptive studies include case reports, case series, and cross-sectional surveys (please note that cross-sectional surveys may be analytical studies as well – this will be discussed in the next article in this series). Examples of descriptive studies include a survey of dietary habits among pregnant women or a case series of patients with an unusual reaction to a drug.
Analytical studies attempt to test a hypothesis and establish causal relationships between variables. In these studies, the researcher assesses the effect of an exposure (or intervention) on an outcome. As described earlier, analytical studies can be observational (if the exposure is naturally determined) or interventional (if the researcher actively administers the intervention).
Directionality of study designs
Based on the direction of inquiry, study designs may be classified as forward-direction or backward-direction. In forward-direction studies, the researcher starts with determining the exposure to a risk factor and then assesses whether the outcome occurs at a future time point. This design is known as a cohort study. For example, a researcher can follow a group of smokers and a group of nonsmokers to determine the incidence of lung cancer in each. In backward-direction studies, the researcher begins by determining whether the outcome is present (cases vs. noncases [also called controls]) and then traces the presence of prior exposure to a risk factor. These are known as case–control studies. For example, a researcher identifies a group of normal-weight babies and a group of low-birth weight babies and then asks the mothers about their dietary habits during the index pregnancy.
Prospective versus retrospective study designs
The terms “prospective” and “retrospective” refer to the timing of the research in relation to the development of the outcome. In retrospective studies, the outcome of interest has already occurred (or not occurred – e.g., in controls) in each individual by the time s/he is enrolled, and the data are collected either from records or by asking participants to recall exposures. There is no follow-up of participants. By contrast, in prospective studies, the outcome (and sometimes even the exposure or intervention) has not occurred when the study starts and participants are followed up over a period of time to determine the occurrence of outcomes. Typically, most cohort studies are prospective studies (though there may be retrospective cohorts), whereas case–control studies are retrospective studies. An interventional study has to be, by definition, a prospective study since the investigator determines the exposure for each study participant and then follows them to observe outcomes.
The terms “prospective” versus “retrospective” studies can be confusing. Let us think of an investigator who starts a case–control study. To him/her, the process of enrolling cases and controls over a period of several months appears prospective. Hence, the use of these terms is best avoided. Or, at the very least, one must be clear that the terms relate to work flow for each individual study participant, and not to the study as a whole.
Classification of study designs
Figure 1 depicts a simple classification of research study designs. The Centre for Evidence-based Medicine has put forward a useful three-point algorithm which can help determine the design of a research study from its methods section:[ 1 ]

- Does the study describe the characteristics of a sample or does it attempt to analyze (or draw inferences about) the relationship between two variables? – If no, then it is a descriptive study, and if yes, it is an analytical (inferential) study
- If analytical, did the investigator determine the exposure? – If no, it is an observational study, and if yes, it is an experimental study
- If observational, when was the outcome determined? – at the start of the study (case–control study), at the end of a period of follow-up (cohort study), or simultaneously (cross sectional).
In the next few pieces in the series, we will discuss various study designs in greater detail.
Financial support and sponsorship
Conflicts of interest.
There are no conflicts of interest.
Epidemiologic methods; research design; research methodology
- + Favorites
- View in Gallery
Readers Of this Article Also Read
Study designs: part 2 – descriptive studies, study designs: part 3 - analytical observational studies, research studies on screening tests, introduction to qualitative research methods – part i, investigator-initiated studies: challenges and solutions.

An official website of the United States government
The .gov means it’s official. Federal government websites often end in .gov or .mil. Before sharing sensitive information, make sure you’re on a federal government site.
The site is secure. The https:// ensures that you are connecting to the official website and that any information you provide is encrypted and transmitted securely.
- Publications
- Account settings
- My Bibliography
- Collections
- Citation manager
Save citation to file
Email citation, add to collections.
- Create a new collection
- Add to an existing collection
Add to My Bibliography
Your saved search, create a file for external citation management software, your rss feed.
- Search in PubMed
- Search in NLM Catalog
- Add to Search
Study designs: Part 1 - An overview and classification
Affiliations.
- 1 Department of Anaesthesiology, Tata Memorial Centre, Mumbai, Maharashtra, India.
- 2 Department of Gastroenterology, Sanjay Gandhi Postgraduate Institute of Medical Sciences, Lucknow, Uttar Pradesh, India.
- PMID: 30319950
- PMCID: PMC6176693
- DOI: 10.4103/picr.PICR_124_18
There are several types of research study designs, each with its inherent strengths and flaws. The study design used to answer a particular research question depends on the nature of the question and the availability of resources. In this article, which is the first part of a series on "study designs," we provide an overview of research study designs and their classification. The subsequent articles will focus on individual designs.
Keywords: Epidemiologic methods; research design; research methodology.
PubMed Disclaimer
Conflict of interest statement
There are no conflicts of interest.
Classification of research study designs
Similar articles
- Designing research: ex post facto designs. Giuffre M. Giuffre M. J Perianesth Nurs. 1997 Jun;12(3):191-5. doi: 10.1016/s1089-9472(97)80038-x. J Perianesth Nurs. 1997. PMID: 9214944 Review.
- A critical synopsis of the diagnostic and screening radiology outcomes literature. Blackmore CC, Black WC, Jarvik JG, Langlotz CP. Blackmore CC, et al. Acad Radiol. 1999 Jan;6 Suppl 1:S8-18. doi: 10.1016/s1076-6332(99)80078-6. Acad Radiol. 1999. PMID: 9891161
- An overview of research designs relevant to nursing: Part 1: Quantitative research designs. Sousa VD, Driessnack M, Mendes IA. Sousa VD, et al. Rev Lat Am Enfermagem. 2007 May-Jun;15(3):502-7. doi: 10.1590/s0104-11692007000300022. Rev Lat Am Enfermagem. 2007. PMID: 17653437
- How to Ask the Right Question and Find the Right Answer: Clinical Research for Transplant Nephrologists. Rodríguez-Ramírez S, Kim SJ. Rodríguez-Ramírez S, et al. Front Immunol. 2022 May 10;13:879200. doi: 10.3389/fimmu.2022.879200. eCollection 2022. Front Immunol. 2022. PMID: 35619692 Free PMC article. Review.
- Methods in epidemiology: observational study designs. DiPietro NA. DiPietro NA. Pharmacotherapy. 2010 Oct;30(10):973-84. doi: 10.1592/phco.30.10.973. Pharmacotherapy. 2010. PMID: 20874034 Review.
- "In sickness and in health": sickness absenteeism in Federal Highway Patrol Officers in the state of Rio Grande do Sul, Brazil. Vasconcelos Júnior JRE, Marins EF, Caputo EL. Vasconcelos Júnior JRE, et al. Rev Bras Med Trab. 2024 Feb 16;21(4):e20231068. doi: 10.47626/1679-4435-2023-1068. eCollection 2023 Oct-Dec. Rev Bras Med Trab. 2024. PMID: 39132279 Free PMC article.
- Comparison ultrasound-guided adductor canal block and surgeon-performed block for pain management after total knee arthroplasty: a prospective randomized controlled study. Cakmak MF, Horoz L, Arslan FN, Demir OU, Basarir K. Cakmak MF, et al. BMC Musculoskelet Disord. 2024 Aug 10;25(1):637. doi: 10.1186/s12891-024-07762-x. BMC Musculoskelet Disord. 2024. PMID: 39127622 Free PMC article. Clinical Trial.
- Increasing perceived health and mental health literacy among separated refugee Ukrainian families with urgent needs occasioned by invasion-a group intervention study with participatory methodology in Sweden. Ekblad S, Gramatik O, Suprun Y. Ekblad S, et al. Front Public Health. 2024 May 9;12:1356605. doi: 10.3389/fpubh.2024.1356605. eCollection 2024. Front Public Health. 2024. PMID: 38799690 Free PMC article.
- Aerobic vaginitis is associated with carbonic anhydrase IX in cervical intraepithelial neoplasia. Grincevičienė Š, Vaitkienė D, Kanopienė D, Vansevičiūtė Petkevičienė R, Sukovas A, Celiešiūtė J, Ivanauskaitė Didžiokienė E, Čižauskas A, Laurinavičienė A, Stravinskienė D, Grincevičius J, Matulis D, Matulienė J. Grincevičienė Š, et al. Sci Rep. 2024 Apr 16;14(1):8789. doi: 10.1038/s41598-024-57427-x. Sci Rep. 2024. PMID: 38627429 Free PMC article.
- Effect of Decision-to-Delivery Time of Emergency Cesarean Section on Adverse Newborn Outcomes at East Gojjam Zone Public Hospital, Ethiopia, March 2023: Multicenter Prospective Observational Study Design. Damtew BS, Gudayu TW, Temesgan WZ, Hailu AM. Damtew BS, et al. Int J Womens Health. 2024 Mar 7;16:433-450. doi: 10.2147/IJWH.S451101. eCollection 2024. Int J Womens Health. 2024. PMID: 38469355 Free PMC article.
- Centre for Evidence-Based Medicine. Study Designs. 2016. [Last accessed on 2018 Sep 04]. Available from: https://www.cebm.net/2014/04/study-designs/
Related information
- Cited in Books
LinkOut - more resources
Full text sources.
- Europe PubMed Central
- Medknow Publications and Media Pvt Ltd
- Ovid Technologies, Inc.
- PubMed Central
- Citation Manager
NCBI Literature Resources
MeSH PMC Bookshelf Disclaimer
The PubMed wordmark and PubMed logo are registered trademarks of the U.S. Department of Health and Human Services (HHS). Unauthorized use of these marks is strictly prohibited.
Study Design Basics: What are study designs?
Study design basics, what are study designs.
- Types of study design
- Study Designs Home
Related guides
- Qualitative Study Designs
- Quantitative Study Designs
Email your Librarians
Study design refers to the methods and methodologies used in resea rch to gather the data needed to explore a specific question.
Some research questions are best approached by statistical analysis of data. This is quantitative research.
Others are better answered by looking for patterns, features or themes in the data. This is qualitative research.
Why do I need to understand study designs?
Unreliable research can still be published even with peer review processes.
Effective evaluation of research involves assessing the way a study has been designed and conducted. This helps you determine how valid the research is for your own study or practice.
Being able to identify and understand different study designs means you can spot the signposts of a good or flawed study. Essentially you are using your critical thinking to evaluate evidence, which you need to do in assessments like literature reviews.
- Next: Types of study design >>
- Last Updated: Jun 13, 2024 10:34 AM
- URL: https://deakin.libguides.com/study-design-basics
What are Analytical Study Designs?
- Research Process
- Peer Review
Analytical study designs can be experimental or observational and each type has its own features. In this article, you'll learn the main types of designs and how to figure out which one you'll need for your study.
Updated on September 19, 2022

A study design is critical to your research study because it determines exactly how you will collect and analyze your data. If your study aims to study the relationship between two variables, then an analytical study design is the right choice.
But how do you know which type of analytical study design is best for your specific research question? It's necessary to have a clear plan before you begin data collection. Lots of researchers, sadly, speed through this or don't do it at all.
When are analytical study designs used?
A study design is a systematic plan, developed so you can carry out your research study effectively and efficiently. Having a design is important because it will determine the right methodologies for your study. Using the right study design makes your results more credible, valid, and coherent.
Descriptive vs. analytical studies
Study designs can be broadly divided into either descriptive or analytical.
Descriptive studies describe characteristics such as patterns or trends. They answer the questions of what, who, where, and when, and they generate hypotheses. They include case reports and qualitative studies.
Analytical study designs quantify a relationship between different variables. They answer the questions of why and how. They're used to test hypotheses and make predictions.
Experimental and observational
Analytical study designs can be either experimental or observational. In experimental studies, researchers manipulate something in a population of interest and examine its effects. These designs are used to establish a causal link between two variables.
In observational studies, in contrast, researchers observe the effects of a treatment or intervention without manipulating anything. Observational studies are most often used to study larger patterns over longer periods.
Experimental study designs
Experimental study designs are when a researcher introduces a change in one group and not in another. Typically, these are used when researchers are interested in the effects of this change on some outcome. It's important to try to ensure that both groups are equivalent at baseline to make sure that any differences that arise are from any introduced change.
In one study, Reiner and colleagues studied the effects of a mindfulness intervention on pain perception . The researchers randomly assigned participants into an experimental group that received a mindfulness training program for two weeks. The rest of the participants were placed in a control group that did not receive the intervention.
Experimental studies help us establish causality. This is critical in science because we want to know whether one variable leads to a change, or causes another. Establishing causality leads to higher internal validity and makes results reproducible.
Experimental designs include randomized control trials (RCTs), nonrandomized control trials (non-RCTs), and crossover designs. Read on to learn the differences.
Randomized control trials
In an RCT, one group of individuals receives an intervention or a treatment, while another does not. It's then possible to investigate what happens to the participants in each group.
Another important feature of RCTs is that participants are randomly assigned to study groups. This helps to limit certain biases and retain better control. Randomization also lets researchers pinpoint any differences in outcomes to the intervention received during the trial. RTCs are considered the gold standard in biomedical research and are considered to provide the best kind of evidence.
For example, one RCT looked at whether an exercise intervention impacts depression . Researchers randomly placed patients with depressive symptoms into intervention groups containing different types of exercise (i.e., light, moderate, or strong). Another group received usual medications or no exercise interventions.
Results showed that after the 12-week trial, patients in all exercise groups had decreased depression levels compared to the control group. This means that by using an RCT design, researchers can now safely assume that the exercise variable has a positive impact on depression.
However, RCTs are not without drawbacks. In the example above, we don't know if exercise still has a positive impact on depression in the long term. This is because it's not feasible to keep people under these controlled settings for a long time.
Advantages of RCTs
- It is possible to infer causality
- Everything is properly controlled, so very little is left to chance or bias
- Can be certain that any difference is coming from the intervention
Disadvantages of RCTs
- Expensive and can be time-consuming
- Can take years for results to be available
- Cannot be done for certain types of questions due to ethical reasons, such as asking participants to undergo harmful treatment
- Limited in how many participants researchers can adequately manage in one study or trial
- Not feasible for people to live under controlled conditions for a long time

Nonrandomized controlled trials
Nonrandomized controlled trials are a type of nonrandomized controlled studies (NRS) where the allocation of participants to intervention groups is not done randomly . Here, researchers purposely assign some participants to one group and others to another group based on certain features. Alternatively, participants can sometimes also decide which group they want to be in.
For example, in one study, clinicians were interested in the impact of stroke recovery after being in an enriched versus non-enriched hospital environment . Patients were selected for the trial if they fulfilled certain requirements common to stroke recovery. Then, the intervention group was given access to an enriched environment (i.e. internet access, reading, going outside), and another group was not. Results showed that the enriched group performed better on cognitive tasks.
NRS are useful in medical research because they help study phenomena that would be difficult to measure with an RCT. However, one of their major drawbacks is that we cannot be sure if the intervention leads to the outcome. In the above example, we can't say for certain whether those patients improved after stroke because they were in the enriched environment or whether there were other variables at play.
Advantages of NRS's
- Good option when randomized control trials are not feasible
- More flexible than RCTs
Disadvantages of NRS's
- Can't be sure if the groups have underlying differences
- Introduces risk of bias and confounds
Crossover study
In a crossover design, each participant receives a sequence of different treatments. Crossover designs can be applied to RCTs, in which each participant is randomly assigned to different study groups.
For example, one study looked at the effects of replacing butter with margarine on lipoproteins levels in individuals with cholesterol . Patients were randomly assigned to a 6-week butter diet, followed by a 6-week margarine diet. In between both diets, participants ate a normal diet for 5 weeks.
These designs are helpful because they reduce bias. In the example above, each participant completed both interventions, making them serve as their own control. However, we don't know if eating butter or margarine first leads to certain results in some subjects.
Advantages of crossover studies
- Each participant serves as their own control, reducing confounding variables
- Require fewer participants, so they have better statistical power
Disadvantages of crossover studies
- Susceptible to order effects, meaning the order in which a treatment was given may have an effect
- Carry-over effects between treatments
Observational studies
In observational studies, researchers watch (observe) the effects of a treatment or intervention without trying to change anything in the population. Observational studies help us establish broad trends and patterns in large-scale datasets or populations. They are also a great alternative when an experimental study is not an option.
Unlike experimental research, observational studies do not help us establish causality. This is because researchers do not actively control any variables. Rather, they investigate statistical relationships between them. Often this is done using a correlational approach.
For example, researchers would like to examine the effects of daily fiber intake on bone density . They conduct a large-scale survey of thousands of individuals to examine correlations of fiber intake with different health measures.
The main observational studies are case-control, cohort, and cross-sectional. Let's take a closer look at each one below.
Case-control study
A case-control is a type of observational design in which researchers identify individuals with an existing health situation (cases) and a similar group without the health issue (controls). The cases and the controls are then compared based on some measurements.
Frequently, data collection in a case-control study is retroactive (i.e., backwards in time). This is because participants have already been exposed to the event in question. Additionally, researchers must go through records and patient files to obtain the records for this study design.
For example, a group of researchers examined whether using sleeping pills puts people at risk of Alzheimer's disease . They selected 1976 individuals that received a dementia diagnosis (“cases”) with 7184 other individuals (“controls”). Cases and controls were matched on specific measures such as sex and age. Patient data was consulted to find out how much sleeping pills were consumed over the course of a certain time.
Case-control is ideal for situations where cases are easy to pick out and compare. For instance, in studying rare diseases or outbreaks.
Advantages of case-control studies
- Feasible for rare diseases
- Cheaper and easier to do than an RCT
Disadvantages of case-control studies
- Relies on patient records, which could be lost or damaged
- Potential recall and selection bias
Cohort study (longitudinal)
A cohort is a group of people who are linked in some way. For instance, a birth year cohort is all people born in a specific year. In cohort studies, researchers compare what happens to individuals in the cohort that have been exposed to some variable compared with those that haven't on different variables. They're also called longitudinal studies.
The cohort is then repeatedly assessed on variables of interest over a period of time. There is no set amount of time required for cohort studies. They can range from a few weeks to many years.
Cohort studies can be prospective. In this case, individuals are followed for some time into the future. They can also be retrospective, where data is collected on a cohort from records.
One of the longest cohort studies today is The Harvard Study of Adult Development . This cohort study has been tracking various health outcomes of 268 Harvard graduates and 456 poor individuals in Boston from 1939 to 2014. Physical screenings, blood samples, brain scans and surveys were collected on this cohort for over 70 years. This study has produced a wealth of knowledge on outcomes throughout life.
A cohort study design is a good option when you have a specific group of people you want to study over time. However, a major drawback is that they take a long time and lack control.
Advantages of cohort studies
- Ethically safe
- Allows you to study multiple outcome variables
- Establish trends and patterns
Disadvantages of cohort studies
- Time consuming and expensive
- Can take many years for results to be revealed
- Too many variables to manage
- Depending on length of study, can have many changes in research personnel
Cross-sectional study
Cross-sectional studies are also known as prevalence studies. They look at the relationship of specific variables in a population in one given time. In cross-sectional studies, the researcher does not try to manipulate any of the variables, just study them using statistical analyses. Cross-sectional studies are also called snapshots of a certain variable or time.
For example, researchers wanted to determine the prevalence of inappropriate antibiotic use to study the growing concern about antibiotic resistance. Participants completed a self-administered questionnaire assessing their knowledge and attitude toward antibiotic use. Then, researchers performed statistical analyses on their responses to determine the relationship between the variables.
Cross-sectional study designs are ideal when gathering initial data on a research question. This data can then be analyzed again later. By knowing the public's general attitudes towards antibiotics, this information can then be relayed to physicians or public health authorities. However, it's often difficult to determine how long these results stay true for.
Advantages of cross-sectional studies
- Fast and inexpensive
- Provides a great deal of information for a given time point
- Leaves room for secondary analysis
Disadvantages of cross-sectional studies
- Requires a large sample to be accurate
- Not clear how long results remain true for
- Do not provide information on causality
- Cannot be used to establish long-term trends because data is only for a given time
So, how about your next study?
Whether it's an RCT, a case-control, or even a qualitative study, AJE has services to help you at every step of the publication process. Get expert guidance and publish your work for the world to see.

The AJE Team
See our "Privacy Policy"
What Are the Types of Study Design?
- Open Access
- First Online: 24 October 2021
Cite this chapter
You have full access to this open access chapter
- Samiran Nundy 4 ,
- Atul Kakar 5 &
- Zulfiqar A. Bhutta 6
34k Accesses
1 Altmetric
The quality, reliability, dependability, and publishability of a study depend on its design. A clinical study design includes the preparation of trials, ]experiments, and observations in research involving human beings. The various types of study designs are depicted in Fig. 8.1.
“Education without application is just entertainment.”Tim Sanders, American author and speaker (1959-)
You have full access to this open access chapter, Download chapter PDF
Similar content being viewed by others
The Beginning – Historical Aspects of Clinical Research, Clinical Research: Definitions, “Anatomy and Physiology,” and the Quest for “Universal Truth”
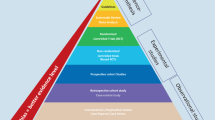
Study designs, levels of evidence, and scientific bias
Introduction to clinical research concepts, essential characteristics of clinical research, overview of clinical research study designs, 1 what are the various types of clinical study designs.
The quality, reliability, dependability, and publishability of a study depend on its design. A clinical study design includes the preparation of trials, experiments, and observations in research involving human beings. The various types of study designs are depicted in Fig. 8.1 .
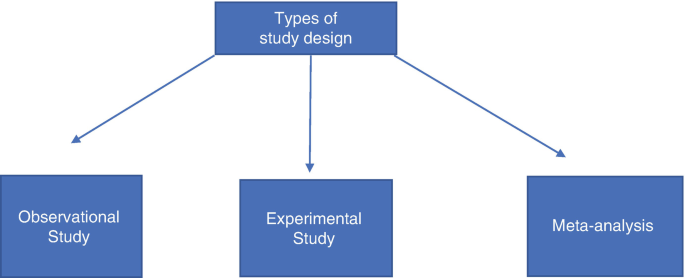
A study can be classified into three major groups: observational, experimental, and meta-analysis
2 What Are the Types of Observational Studies?
Broadly there are two types of observational studies, i.e.:
Descriptive.
Analytical.
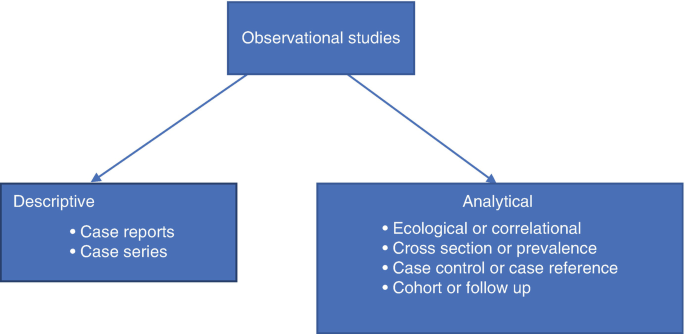
3 What Is a Descriptive Study?
This kind of study deals with observing the distribution of a given phenomenon. It generally deals with a time, place, and person distribution [ 1 , 2 ].
The procedures involved in a descriptive study include:
Definition of the population to be studied.
Naming of the illness.
Describing the disease by time, place, and person.
Quantification of the disease outcome.
Comparing this with known parameters.
The advantages of a descriptive study:
Provides information regarding the extent of the disease load.
May suggest a clue to its aetiology.
Provides background data for planning.
Contributes to research by describing the illness in relation to time, place, and persons.
Examples of descriptive studies include:
Case reports 1. Profound neutropenia in a patient with COVID-19.
2. Multiple Renal Abscesses in a Horseshoe Kidney
Case series 1. Gastrointestinal manifestations in COVID-19: A review of 30 cases.
2. Long-Term Follow-Ups of Relapses after Surgery for Astrocytoma
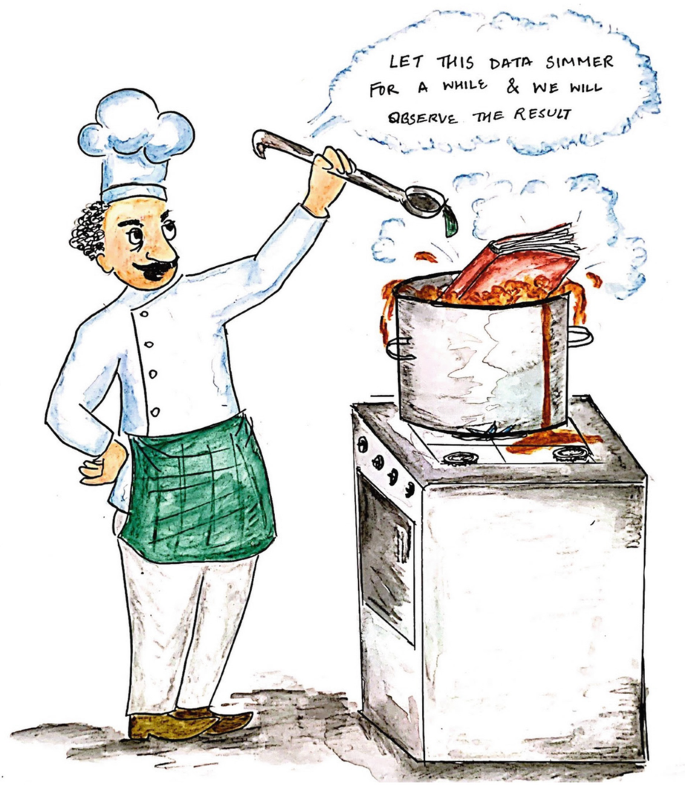
4 What Is Analytical Epidemiology?
The objective of this kind of study is to test a hypothesis and includes specific subjects of interest. There are four distinct types of investigations:
Ecological.
Cross sectional.
Case control.
5 What Are Ecological Studies?
These are observational studies often used to measure the prevalence and incidence of disease, particularly when the disease is rare, and are quite easy to conduct. The other advantage is that they are usually retrospective in nature. In them, there should be only one exposure in the population. An example of such a study would be to compare the prevalence of rheumatoid arthritis in Delhi and Manipur. This data is usually extracted from large databases which may have been used for other purposes and thus are not always reliable. Ecological studies are generally economical and serve as a preliminary point for hypothesis generation [ 2 ].
6 What Is a Case–Control Study?
This compares a population with a certain medical condition with another group of people who do not have the disease but are otherwise similar to the study population.
The basic steps include:
Proper selecting cases and controls.
Matching of cases with controls.
Measuring the exposure.
Analyzing and interpreting the results.
Case–control studies are inexpensive and frequently used kind in epidemiology. Their design allows the study of a rare illness. The preliminary data help to learn what is already known about the association between the risk factors for the disease. The measure of interest is the calculation of the odds ratio. These are also retrospective studies that cannot calculate prevalence and are usually used for rare diseases. They can also be nested within longitudinal studies but given their retrospective nature, can be prone to recall bias [ 3 ].
An example of such a study is the occurrence of cervical cancer in patients who have received Human Papillomavirus vaccine in childhood. Figure 8.2 is an example of a case–control study design and how to calculate the odd’s ratio.
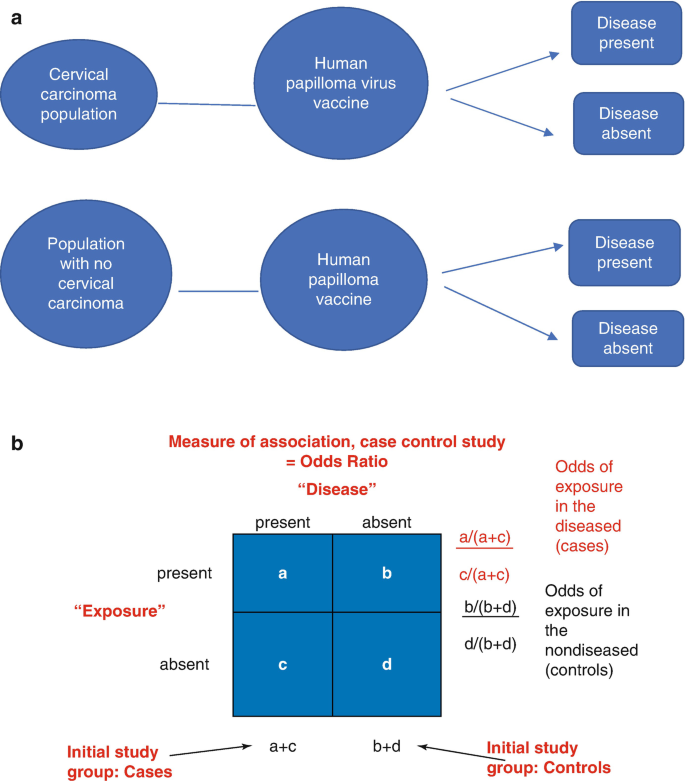
( a , b ) Case–control study and calculation of odds ratio
7 What Is a Cohort Study?
A cohort study is done on a group of people who are followed up over many years—for instance, to determine how often a certain disease occurs. It is performed to obtain evidence to support the existence of an association between a suspected cause and disease (Fig. 8.3 ).
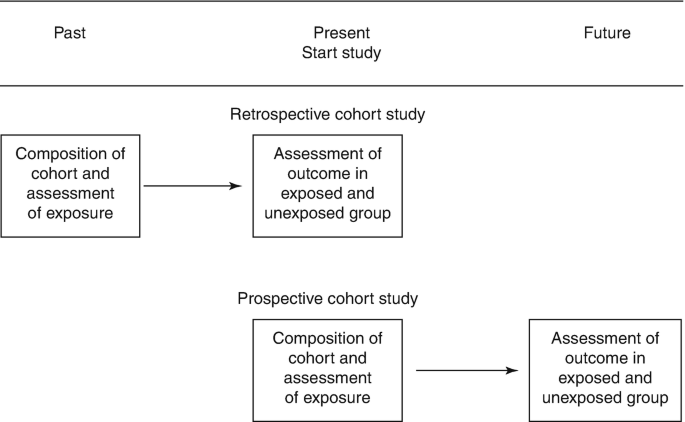
Depicts both a retrospective and a prospective cohort study
Types of cohort study:
Prospective cohorts.
Retrospective cohorts.
Combination of prospective and retrospective cohorts.
Elements of a cohort study:
Collection of study patients.
Procuring data on exposure.
Study of comparison groups.
Review visits.
Final data analysis.
These studies can help in calculating point prevalence or period prevalence. Prospective cohort studies are the ‘gold standard for observational research’.
8 What Are Cross-Sectional Studies?
These are also retrospective and study the prevalence of a disease. They are economical and easy to conduct. An example of a cross-sectional study design would be enrolling participants who are either current alcohol consumers or have never consumed alcohol, and are being assessed whether or not they have liver-related issues. The studies assess both exposure and outcome at a single point in time. Figure 8.4 shows an example of this [ 3 , 4 ].
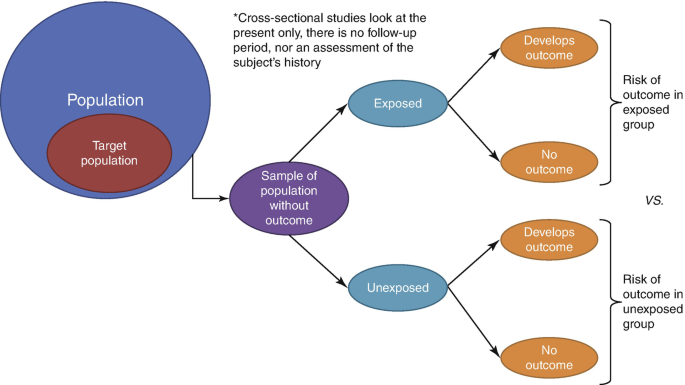
Cross-sectional study
9 What Is an Experimental Study Design?
This has a similar approach to a cohort study except that it is carried out under direct control of an investigator. The aim is to provide systematic proof of either aetiological or risk factors of the disease the modification of which can control it. Epidemiological and interventional research studies include three elements:
Definition and measure of exposure in two or more groups.
Measure of disease outcome(s) in the same groups.
Statistical comparison made between groups to assess potential relationships between the exposure and outcome, all of which are defined by the researcher.
10 What Is a Randomized Controlled Trial?
This is a study performed to avoid any bias while testing for the efficacy of, e.g., a drug. The study population is randomly divided into two groups, of which one receives the drug under study and the second group receives a placebo and acts as the control group. The experiment may be blinded, which means that any information which may influence the participant is withheld while the trial is ongoing or maybe double blinded in which the information is withheld from both the subject and the investigator [ 5 ].
The basic steps of a randomized control trial (RCT) include:
Writing a protocol.
Selecting a normal and experimental population.
Randomization.
Intervention in the study group and placebo.
Measuring the outcome of interest.
11 Design of a Randomized Control Trial (Fig. 8.5 )
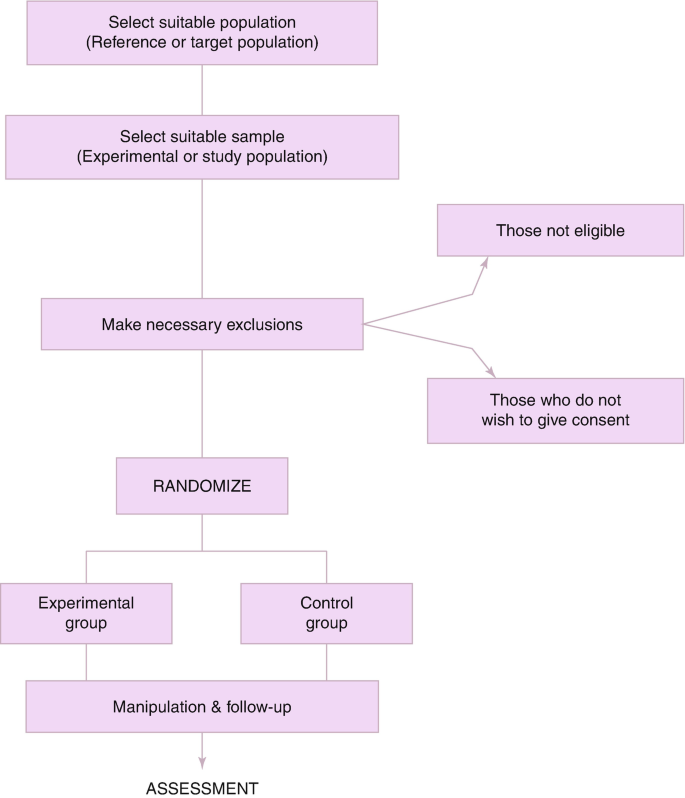
Randomized control trial
12 What Are the Standards of Research and Reporting?
There are many available guidelines on study design, execution, and how it needs to be reported in the final manuscript. This improves the quality of a research paper and allows results to be presented in a systematic manner for a sound conclusion to be drawn. Table 8.1 mentions some important reporting formats and their websites.
13 Conclusions
Formulating a study design is the most important part of the planning stage of clinical research. It is an indispensable part of new drug discovery.
Basic research is also called experimental and done in genetics, biochemistry, and physiology. Studies on drug properties are also included in this.
Clinical studies can be interventional or non-interventional. Interventional studies are done on surgery, chemotherapeutic agents, devices, or drugs.
A rare disease is best investigated by a case–control study and rare exposures by cohort studies.
A retrospective study is based on historical data, which may be obtained from past records. In prospective studies the data are collected after the work has begun.
Observational studies are divided into descriptive and analytical studies.
In cohort studies, two or more groups are selected on the basis of their exposure to a drug or environmental exposure and then followed up for outcome.
The evidence collected from randomized controlled trials is of good quality. They allow a proper evaluation of a drug. More recently adaptive designs allow for greater flexibility and pragmatic randomized trials.
Aggarwal R, Ranganathan P. Study designs: part 2 – descriptive studies. Perspect Clin Res. 2019;10(1):34–6.
Article Google Scholar
Thiese MS. Observational and interventional study design types; an overview. Biochem Med (Zagreb). 2014;24:199–210.
Anglemyer A, Horvath HT, Bero L. Healthcare outcomes assessed with observational study designs compared with those assessed in randomized trials. Cochrane Database Syst Rev. 2014;4:MR000034.
Google Scholar
Centers for disease control and prevention. Descriptive and analytic studies. Last accessed on 20th April 2020. Available on https://www.cdc.gov/globalhealth/healthprotection/fetp/training_modules/19/desc-and-analytic-studies_ppt_final_09252013.pdf .
Kendall JM. Designing a research project: randomised controlled trials and their principles. Emergency Med J. 2003;20:164–8.
Article CAS Google Scholar
CONSORT-transparent reporting of trials. Lasts accessed on 20th April 2020. Available on http://www.consort-statement.org/downloads .
PRISMA- Transparent reporting of systematic reviews and meta analyses. Lasts accessed on 20th April 2020. Available on http://www.prisma-statement.org/ .
STROBE statement- strengthening the reporting of observational studies in epidemiology. Lasts accessed on 20th April 2020. Available on https://www.strobe-statement.org/home .
CARE- case report guidelines. Lasts accessed on 20th April 2020. Available on https://www.care-statement.org/ .
OXFORD academic. Lasts accessed on 20th April 2020. Available on https://academic.oup.com
Download references
Author information
Authors and affiliations.
Department of Surgical Gastroenterology and Liver Transplantation, Sir Ganga Ram Hospital, New Delhi, India
Samiran Nundy
Department of Internal Medicine, Sir Ganga Ram Hospital, New Delhi, India
Institute for Global Health and Development, The Aga Khan University, South Central Asia, East Africa and United Kingdom, Karachi, Pakistan
Zulfiqar A. Bhutta
You can also search for this author in PubMed Google Scholar
Rights and permissions
Open Access This chapter is licensed under the terms of the Creative Commons Attribution 4.0 International License ( http://creativecommons.org/licenses/by/4.0/ ), which permits use, sharing, adaptation, distribution and reproduction in any medium or format, as long as you give appropriate credit to the original author(s) and the source, provide a link to the Creative Commons license and indicate if changes were made.
The images or other third party material in this chapter are included in the chapter's Creative Commons license, unless indicated otherwise in a credit line to the material. If material is not included in the chapter's Creative Commons license and your intended use is not permitted by statutory regulation or exceeds the permitted use, you will need to obtain permission directly from the copyright holder.
Reprints and permissions
Copyright information
© 2022 The Author(s)
About this chapter
Nundy, S., Kakar, A., Bhutta, Z.A. (2022). What Are the Types of Study Design?. In: How to Practice Academic Medicine and Publish from Developing Countries?. Springer, Singapore. https://doi.org/10.1007/978-981-16-5248-6_8
Download citation
DOI : https://doi.org/10.1007/978-981-16-5248-6_8
Published : 24 October 2021
Publisher Name : Springer, Singapore
Print ISBN : 978-981-16-5247-9
Online ISBN : 978-981-16-5248-6
eBook Packages : Medicine Medicine (R0)
Share this chapter
Anyone you share the following link with will be able to read this content:
Sorry, a shareable link is not currently available for this article.
Provided by the Springer Nature SharedIt content-sharing initiative
- Publish with us
Policies and ethics
- Find a journal
- Track your research

An official website of the United States government
The .gov means it’s official. Federal government websites often end in .gov or .mil. Before sharing sensitive information, make sure you’re on a federal government site.
The site is secure. The https:// ensures that you are connecting to the official website and that any information you provide is encrypted and transmitted securely.
- Publications
- Account settings
Preview improvements coming to the PMC website in October 2024. Learn More or Try it out now .
- Advanced Search
- Journal List
- Indian J Anaesth
- v.60(9); 2016 Sep
Types of studies and research design
Mukul chandra kapoor.
Department of Anesthesiology, Max Smart Super Specialty Hospital, New Delhi, India
Medical research has evolved, from individual expert described opinions and techniques, to scientifically designed methodology-based studies. Evidence-based medicine (EBM) was established to re-evaluate medical facts and remove various myths in clinical practice. Research methodology is now protocol based with predefined steps. Studies were classified based on the method of collection and evaluation of data. Clinical study methodology now needs to comply to strict ethical, moral, truth, and transparency standards, ensuring that no conflict of interest is involved. A medical research pyramid has been designed to grade the quality of evidence and help physicians determine the value of the research. Randomised controlled trials (RCTs) have become gold standards for quality research. EBM now scales systemic reviews and meta-analyses at a level higher than RCTs to overcome deficiencies in the randomised trials due to errors in methodology and analyses.
INTRODUCTION
Expert opinion, experience, and authoritarian judgement were the norm in clinical medical practice. At scientific meetings, one often heard senior professionals emphatically expressing ‘In my experience,…… what I have said is correct!’ In 1981, articles published by Sackett et al . introduced ‘critical appraisal’ as they felt a need to teach methods of understanding scientific literature and its application at the bedside.[ 1 ] To improve clinical outcomes, clinical expertise must be complemented by the best external evidence.[ 2 ] Conversely, without clinical expertise, good external evidence may be used inappropriately [ Figure 1 ]. Practice gets outdated, if not updated with current evidence, depriving the clientele of the best available therapy.

Triad of evidence-based medicine
EVIDENCE-BASED MEDICINE
In 1971, in his book ‘Effectiveness and Efficiency’, Archibald Cochrane highlighted the lack of reliable evidence behind many accepted health-care interventions.[ 3 ] This triggered re-evaluation of many established ‘supposed’ scientific facts and awakened physicians to the need for evidence in medicine. Evidence-based medicine (EBM) thus evolved, which was defined as ‘the conscientious, explicit and judicious use of the current best evidence in making decisions about the care of individual patients.’[ 2 ]
The goal of EBM was scientific endowment to achieve consistency, efficiency, effectiveness, quality, safety, reduction in dilemma and limitation of idiosyncrasies in clinical practice.[ 4 ] EBM required the physician to diligently assess the therapy, make clinical adjustments using the best available external evidence, ensure awareness of current research and discover clinical pathways to ensure best patient outcomes.[ 5 ]
With widespread internet use, phenomenally large number of publications, training and media resources are available but determining the quality of this literature is difficult for a busy physician. Abstracts are available freely on the internet, but full-text articles require a subscription. To complicate issues, contradictory studies are published making decision-making difficult.[ 6 ] Publication bias, especially against negative studies, makes matters worse.
In 1993, the Cochrane Collaboration was founded by Ian Chalmers and others to create and disseminate up-to-date review of randomised controlled trials (RCTs) to help health-care professionals make informed decisions.[ 7 ] In 1995, the American College of Physicians and the British Medical Journal Publishing Group collaborated to publish the journal ‘Evidence-based medicine’, leading to the evolution of EBM in all spheres of medicine.
MEDICAL RESEARCH
Medical research needs to be conducted to increase knowledge about the human species, its social/natural environment and to combat disease/infirmity in humans. Research should be conducted in a manner conducive to and consistent with dignity and well-being of the participant; in a professional and transparent manner; and ensuring minimal risk.[ 8 ] Research thus must be subjected to careful evaluation at all stages, i.e., research design/experimentation; results and their implications; the objective of the research sought; anticipated benefits/dangers; potential uses/abuses of the experiment and its results; and on ensuring the safety of human life. Table 1 lists the principles any research should follow.[ 8 ]
General principles of medical research

Types of study design
Medical research is classified into primary and secondary research. Clinical/experimental studies are performed in primary research, whereas secondary research consolidates available studies as reviews, systematic reviews and meta-analyses. Three main areas in primary research are basic medical research, clinical research and epidemiological research [ Figure 2 ]. Basic research includes fundamental research in fields shown in Figure 2 . In almost all studies, at least one independent variable is varied, whereas the effects on the dependent variables are investigated. Clinical studies include observational studies and interventional studies and are subclassified as in Figure 2 .

Classification of types of medical research
Interventional clinical study is performed with the purpose of studying or demonstrating clinical or pharmacological properties of drugs/devices, their side effects and to establish their efficacy or safety. They also include studies in which surgical, physical or psychotherapeutic procedures are examined.[ 9 ] Studies on drugs/devices are subject to legal and ethical requirements including the Drug Controller General India (DCGI) directives. They require the approval of DCGI recognized Ethics Committee and must be performed in accordance with the rules of ‘Good Clinical Practice’.[ 10 ] Further details are available under ‘Methodology for research II’ section in this issue of IJA. In 2004, the World Health Organization advised registration of all clinical trials in a public registry. In India, the Clinical Trials Registry of India was launched in 2007 ( www.ctri.nic.in ). The International Committee of Medical Journal Editors (ICMJE) mandates its member journals to publish only registered trials.[ 11 ]
Observational clinical study is a study in which knowledge from treatment of persons with drugs is analysed using epidemiological methods. In these studies, the diagnosis, treatment and monitoring are performed exclusively according to medical practice and not according to a specified study protocol.[ 9 ] They are subclassified as per Figure 2 .
Epidemiological studies have two basic approaches, the interventional and observational. Clinicians are more familiar with interventional research, whereas epidemiologists usually perform observational research.
Interventional studies are experimental in character and are subdivided into field and group studies, for example, iodine supplementation of cooking salt to prevent hypothyroidism. Many interventions are unsuitable for RCTs, as the exposure may be harmful to the subjects.
Observational studies can be subdivided into cohort, case–control, cross-sectional and ecological studies.
- Cohort studies are suited to detect connections between exposure and development of disease. They are normally prospective studies of two healthy groups of subjects observed over time, in which one group is exposed to a specific substance, whereas the other is not. The occurrence of the disease can be determined in the two groups. Cohort studies can also be retrospective
- Case–control studies are retrospective analyses performed to establish the prevalence of a disease in two groups exposed to a factor or disease. The incidence rate cannot be calculated, and there is also a risk of selection bias and faulty recall.
Secondary research
Narrative review.
An expert senior author writes about a particular field, condition or treatment, including an overview, and this information is fortified by his experience. The article is in a narrative format. Its limitation is that one cannot tell whether recommendations are based on author's clinical experience, available literature and why some studies were given more emphasis. It can be biased, with selective citation of reports that reinforce the authors' views of a topic.[ 12 ]
Systematic review
Systematic reviews methodically and comprehensively identify studies focused on a specified topic, appraise their methodology, summate the results, identify key findings and reasons for differences across studies, and cite limitations of current knowledge.[ 13 ] They adhere to reproducible methods and recommended guidelines.[ 14 ] The methods used to compile data are explicit and transparent, allowing the reader to gauge the quality of the review and the potential for bias.[ 15 ]
A systematic review can be presented in text or graphic form. In graphic form, data of different trials can be plotted with the point estimate and 95% confidence interval for each study, presented on an individual line. A properly conducted systematic review presents the best available research evidence for a focused clinical question. The review team may obtain information, not available in the original reports, from the primary authors. This ensures that findings are consistent and generalisable across populations, environment, therapies and groups.[ 12 ] A systematic review attempts to reduce bias identification and studies selection for review, using a comprehensive search strategy and specifying inclusion criteria. The strength of a systematic review lies in the transparency of each phase and highlighting the merits of each decision made, while compiling information.
Meta-analysis
A review team compiles aggregate-level data in each primary study, and in some cases, data are solicited from each of the primary studies.[ 16 , 17 ] Although difficult to perform, individual patient meta-analyses offer advantages over aggregate-level analyses.[ 18 ] These mathematically pooled results are referred to as meta-analysis. Combining data from well-conducted primary studies provide a precise estimate of the “true effect.”[ 19 ] Pooling the samples of individual studies increases overall sample size, enhances statistical analysis power, reduces confidence interval and thereby improves statistical value.
The structured process of Cochrane Collaboration systematic reviews has contributed to the improvement of their quality. For the meta-analysis to be definitive, the primary RCTs should have been conducted methodically. When the existing studies have important scientific and methodological limitations, such as smaller sized samples, the systematic review may identify where gaps exist in the available literature.[ 20 ] RCTs and systematic review of several randomised trials are less likely to mislead us, and thereby help judge whether an intervention is better.[ 2 ] Practice guidelines supported by large RCTs and meta-analyses are considered as ‘gold standard’ in EBM. This issue of IJA is accompanied by an editorial on Importance of EBM on research and practice (Guyat and Sriganesh 471_16).[ 21 ] The EBM pyramid grading the value of different types of research studies is shown in Figure 3 .

The evidence-based medicine pyramid
In the last decade, a number of studies and guidelines brought about path-breaking changes in anaesthesiology and critical care. Some guidelines such as the ‘Surviving Sepsis Guidelines-2004’[ 22 ] were later found to be flawed and biased. A number of large RCTs were rejected as their findings were erroneous. Another classic example is that of ENIGMA-I (Evaluation of Nitrous oxide In the Gas Mixture for Anaesthesia)[ 23 ] which implicated nitrous oxide for poor outcomes, but ENIGMA-II[ 24 , 25 ] conducted later, by the same investigators, declared it as safe. The rise and fall of the ‘tight glucose control’ regimen was similar.[ 26 ]
Although RCTs are considered ‘gold standard’ in research, their status is at crossroads today. RCTs have conflicting interests and thus must be evaluated with careful scrutiny. EBM can promote evidence reflected in RCTs and meta-analyses. However, it cannot promulgate evidence not reflected in RCTs. Flawed RCTs and meta-analyses may bring forth erroneous recommendations. EBM thus should not be restricted to RCTs and meta-analyses but must involve tracking down the best external evidence to answer our clinical questions.
Financial support and sponsorship
Conflicts of interest.
There are no conflicts of interest.

Organizing Academic Research Papers: Types of Research Designs
- Purpose of Guide
- Design Flaws to Avoid
- Glossary of Research Terms
- Narrowing a Topic Idea
- Broadening a Topic Idea
- Extending the Timeliness of a Topic Idea
- Academic Writing Style
- Choosing a Title
- Making an Outline
- Paragraph Development
- Executive Summary
- Background Information
- The Research Problem/Question
- Theoretical Framework
- Citation Tracking
- Content Alert Services
- Evaluating Sources
- Primary Sources
- Secondary Sources
- Tertiary Sources
- What Is Scholarly vs. Popular?
- Qualitative Methods
- Quantitative Methods
- Using Non-Textual Elements
- Limitations of the Study
- Common Grammar Mistakes
- Avoiding Plagiarism
- Footnotes or Endnotes?
- Further Readings
- Annotated Bibliography
- Dealing with Nervousness
- Using Visual Aids
- Grading Someone Else's Paper
- How to Manage Group Projects
- Multiple Book Review Essay
- Reviewing Collected Essays
- About Informed Consent
- Writing Field Notes
- Writing a Policy Memo
- Writing a Research Proposal
- Acknowledgements
Introduction
Before beginning your paper, you need to decide how you plan to design the study .
The research design refers to the overall strategy that you choose to integrate the different components of the study in a coherent and logical way, thereby, ensuring you will effectively address the research problem; it constitutes the blueprint for the collection, measurement, and analysis of data. Note that your research problem determines the type of design you can use, not the other way around!
General Structure and Writing Style
Action research design, case study design, causal design, cohort design, cross-sectional design, descriptive design, experimental design, exploratory design, historical design, longitudinal design, observational design, philosophical design, sequential design.
Kirshenblatt-Gimblett, Barbara. Part 1, What Is Research Design? The Context of Design. Performance Studies Methods Course syllabus . New York University, Spring 2006; Trochim, William M.K. Research Methods Knowledge Base . 2006.
The function of a research design is to ensure that the evidence obtained enables you to effectively address the research problem as unambiguously as possible. In social sciences research, obtaining evidence relevant to the research problem generally entails specifying the type of evidence needed to test a theory, to evaluate a program, or to accurately describe a phenomenon. However, researchers can often begin their investigations far too early, before they have thought critically about about what information is required to answer the study's research questions. Without attending to these design issues beforehand, the conclusions drawn risk being weak and unconvincing and, consequently, will fail to adequate address the overall research problem.
Given this, the length and complexity of research designs can vary considerably, but any sound design will do the following things:
- Identify the research problem clearly and justify its selection,
- Review previously published literature associated with the problem area,
- Clearly and explicitly specify hypotheses [i.e., research questions] central to the problem selected,
- Effectively describe the data which will be necessary for an adequate test of the hypotheses and explain how such data will be obtained, and
- Describe the methods of analysis which will be applied to the data in determining whether or not the hypotheses are true or false.
Kirshenblatt-Gimblett, Barbara. Part 1, What Is Research Design? The Context of Design. Performance Studies Methods Course syllabus . New Yortk University, Spring 2006.
Definition and Purpose
The essentials of action research design follow a characteristic cycle whereby initially an exploratory stance is adopted, where an understanding of a problem is developed and plans are made for some form of interventionary strategy. Then the intervention is carried out (the action in Action Research) during which time, pertinent observations are collected in various forms. The new interventional strategies are carried out, and the cyclic process repeats, continuing until a sufficient understanding of (or implement able solution for) the problem is achieved. The protocol is iterative or cyclical in nature and is intended to foster deeper understanding of a given situation, starting with conceptualizing and particularizing the problem and moving through several interventions and evaluations.
What do these studies tell you?
- A collaborative and adaptive research design that lends itself to use in work or community situations.
- Design focuses on pragmatic and solution-driven research rather than testing theories.
- When practitioners use action research it has the potential to increase the amount they learn consciously from their experience. The action research cycle can also be regarded as a learning cycle.
- Action search studies often have direct and obvious relevance to practice.
- There are no hidden controls or preemption of direction by the researcher.
What these studies don't tell you?
- It is harder to do than conducting conventional studies because the researcher takes on responsibilities for encouraging change as well as for research.
- Action research is much harder to write up because you probably can’t use a standard format to report your findings effectively.
- Personal over-involvement of the researcher may bias research results.
- The cyclic nature of action research to achieve its twin outcomes of action (e.g. change) and research (e.g. understanding) is time-consuming and complex to conduct.
Gall, Meredith. Educational Research: An Introduction . Chapter 18, Action Research. 8th ed. Boston, MA: Pearson/Allyn and Bacon, 2007; Kemmis, Stephen and Robin McTaggart. “Participatory Action Research.” In Handbook of Qualitative Research . Norman Denzin and Yvonna S. Locoln, eds. 2nd ed. (Thousand Oaks, CA: SAGE, 2000), pp. 567-605.; Reason, Peter and Hilary Bradbury. Handbook of Action Research: Participative Inquiry and Practice . Thousand Oaks, CA: SAGE, 2001.
A case study is an in-depth study of a particular research problem rather than a sweeping statistical survey. It is often used to narrow down a very broad field of research into one or a few easily researchable examples. The case study research design is also useful for testing whether a specific theory and model actually applies to phenomena in the real world. It is a useful design when not much is known about a phenomenon.
- Approach excels at bringing us to an understanding of a complex issue through detailed contextual analysis of a limited number of events or conditions and their relationships.
- A researcher using a case study design can apply a vaiety of methodologies and rely on a variety of sources to investigate a research problem.
- Design can extend experience or add strength to what is already known through previous research.
- Social scientists, in particular, make wide use of this research design to examine contemporary real-life situations and provide the basis for the application of concepts and theories and extension of methods.
- The design can provide detailed descriptions of specific and rare cases.
- A single or small number of cases offers little basis for establishing reliability or to generalize the findings to a wider population of people, places, or things.
- The intense exposure to study of the case may bias a researcher's interpretation of the findings.
- Design does not facilitate assessment of cause and effect relationships.
- Vital information may be missing, making the case hard to interpret.
- The case may not be representative or typical of the larger problem being investigated.
- If the criteria for selecting a case is because it represents a very unusual or unique phenomenon or problem for study, then your intepretation of the findings can only apply to that particular case.
Anastas, Jeane W. Research Design for Social Work and the Human Services . Chapter 4, Flexible Methods: Case Study Design. 2nd ed. New York: Columbia University Press, 1999; Stake, Robert E. The Art of Case Study Research . Thousand Oaks, CA: SAGE, 1995; Yin, Robert K. Case Study Research: Design and Theory . Applied Social Research Methods Series, no. 5. 3rd ed. Thousand Oaks, CA: SAGE, 2003.
Causality studies may be thought of as understanding a phenomenon in terms of conditional statements in the form, “If X, then Y.” This type of research is used to measure what impact a specific change will have on existing norms and assumptions. Most social scientists seek causal explanations that reflect tests of hypotheses. Causal effect (nomothetic perspective) occurs when variation in one phenomenon, an independent variable, leads to or results, on average, in variation in another phenomenon, the dependent variable.
Conditions necessary for determining causality:
- Empirical association--a valid conclusion is based on finding an association between the independent variable and the dependent variable.
- Appropriate time order--to conclude that causation was involved, one must see that cases were exposed to variation in the independent variable before variation in the dependent variable.
- Nonspuriousness--a relationship between two variables that is not due to variation in a third variable.
- Causality research designs helps researchers understand why the world works the way it does through the process of proving a causal link between variables and eliminating other possibilities.
- Replication is possible.
- There is greater confidence the study has internal validity due to the systematic subject selection and equity of groups being compared.
- Not all relationships are casual! The possibility always exists that, by sheer coincidence, two unrelated events appear to be related [e.g., Punxatawney Phil could accurately predict the duration of Winter for five consecutive years but, the fact remains, he's just a big, furry rodent].
- Conclusions about causal relationships are difficult to determine due to a variety of extraneous and confounding variables that exist in a social environment. This means causality can only be inferred, never proven.
- If two variables are correlated, the cause must come before the effect. However, even though two variables might be causally related, it can sometimes be difficult to determine which variable comes first and therefore to establish which variable is the actual cause and which is the actual effect.
Bachman, Ronet. The Practice of Research in Criminology and Criminal Justice . Chapter 5, Causation and Research Designs. 3rd ed. Thousand Oaks, CA: Pine Forge Press, 2007; Causal Research Design: Experimentation. Anonymous SlideShare Presentation ; Gall, Meredith. Educational Research: An Introduction . Chapter 11, Nonexperimental Research: Correlational Designs. 8th ed. Boston, MA: Pearson/Allyn and Bacon, 2007; Trochim, William M.K. Research Methods Knowledge Base . 2006.
Often used in the medical sciences, but also found in the applied social sciences, a cohort study generally refers to a study conducted over a period of time involving members of a population which the subject or representative member comes from, and who are united by some commonality or similarity. Using a quantitative framework, a cohort study makes note of statistical occurrence within a specialized subgroup, united by same or similar characteristics that are relevant to the research problem being investigated, r ather than studying statistical occurrence within the general population. Using a qualitative framework, cohort studies generally gather data using methods of observation. Cohorts can be either "open" or "closed."
- Open Cohort Studies [dynamic populations, such as the population of Los Angeles] involve a population that is defined just by the state of being a part of the study in question (and being monitored for the outcome). Date of entry and exit from the study is individually defined, therefore, the size of the study population is not constant. In open cohort studies, researchers can only calculate rate based data, such as, incidence rates and variants thereof.
- Closed Cohort Studies [static populations, such as patients entered into a clinical trial] involve participants who enter into the study at one defining point in time and where it is presumed that no new participants can enter the cohort. Given this, the number of study participants remains constant (or can only decrease).
- The use of cohorts is often mandatory because a randomized control study may be unethical. For example, you cannot deliberately expose people to asbestos, you can only study its effects on those who have already been exposed. Research that measures risk factors often relies on cohort designs.
- Because cohort studies measure potential causes before the outcome has occurred, they can demonstrate that these “causes” preceded the outcome, thereby avoiding the debate as to which is the cause and which is the effect.
- Cohort analysis is highly flexible and can provide insight into effects over time and related to a variety of different types of changes [e.g., social, cultural, political, economic, etc.].
- Either original data or secondary data can be used in this design.
- In cases where a comparative analysis of two cohorts is made [e.g., studying the effects of one group exposed to asbestos and one that has not], a researcher cannot control for all other factors that might differ between the two groups. These factors are known as confounding variables.
- Cohort studies can end up taking a long time to complete if the researcher must wait for the conditions of interest to develop within the group. This also increases the chance that key variables change during the course of the study, potentially impacting the validity of the findings.
- Because of the lack of randominization in the cohort design, its external validity is lower than that of study designs where the researcher randomly assigns participants.
Healy P, Devane D. “Methodological Considerations in Cohort Study Designs.” Nurse Researcher 18 (2011): 32-36; Levin, Kate Ann. Study Design IV: Cohort Studies. Evidence-Based Dentistry 7 (2003): 51–52; Study Design 101 . Himmelfarb Health Sciences Library. George Washington University, November 2011; Cohort Study . Wikipedia.
Cross-sectional research designs have three distinctive features: no time dimension, a reliance on existing differences rather than change following intervention; and, groups are selected based on existing differences rather than random allocation. The cross-sectional design can only measure diffrerences between or from among a variety of people, subjects, or phenomena rather than change. As such, researchers using this design can only employ a relative passive approach to making causal inferences based on findings.
- Cross-sectional studies provide a 'snapshot' of the outcome and the characteristics associated with it, at a specific point in time.
- Unlike the experimental design where there is an active intervention by the researcher to produce and measure change or to create differences, cross-sectional designs focus on studying and drawing inferences from existing differences between people, subjects, or phenomena.
- Entails collecting data at and concerning one point in time. While longitudinal studies involve taking multiple measures over an extended period of time, cross-sectional research is focused on finding relationships between variables at one moment in time.
- Groups identified for study are purposely selected based upon existing differences in the sample rather than seeking random sampling.
- Cross-section studies are capable of using data from a large number of subjects and, unlike observational studies, is not geographically bound.
- Can estimate prevalence of an outcome of interest because the sample is usually taken from the whole population.
- Because cross-sectional designs generally use survey techniques to gather data, they are relatively inexpensive and take up little time to conduct.
- Finding people, subjects, or phenomena to study that are very similar except in one specific variable can be difficult.
- Results are static and time bound and, therefore, give no indication of a sequence of events or reveal historical contexts.
- Studies cannot be utilized to establish cause and effect relationships.
- Provide only a snapshot of analysis so there is always the possibility that a study could have differing results if another time-frame had been chosen.
- There is no follow up to the findings.
Hall, John. “Cross-Sectional Survey Design.” In Encyclopedia of Survey Research Methods. Paul J. Lavrakas, ed. (Thousand Oaks, CA: Sage, 2008), pp. 173-174; Helen Barratt, Maria Kirwan. Cross-Sectional Studies: Design, Application, Strengths and Weaknesses of Cross-Sectional Studies . Healthknowledge, 2009. Cross-Sectional Study . Wikipedia.
Descriptive research designs help provide answers to the questions of who, what, when, where, and how associated with a particular research problem; a descriptive study cannot conclusively ascertain answers to why. Descriptive research is used to obtain information concerning the current status of the phenomena and to describe "what exists" with respect to variables or conditions in a situation.
- The subject is being observed in a completely natural and unchanged natural environment. True experiments, whilst giving analyzable data, often adversely influence the normal behavior of the subject.
- Descriptive research is often used as a pre-cursor to more quantitatively research designs, the general overview giving some valuable pointers as to what variables are worth testing quantitatively.
- If the limitations are understood, they can be a useful tool in developing a more focused study.
- Descriptive studies can yield rich data that lead to important recommendations.
- Appoach collects a large amount of data for detailed analysis.
- The results from a descriptive research can not be used to discover a definitive answer or to disprove a hypothesis.
- Because descriptive designs often utilize observational methods [as opposed to quantitative methods], the results cannot be replicated.
- The descriptive function of research is heavily dependent on instrumentation for measurement and observation.
Anastas, Jeane W. Research Design for Social Work and the Human Services . Chapter 5, Flexible Methods: Descriptive Research. 2nd ed. New York: Columbia University Press, 1999; McNabb, Connie. Descriptive Research Methodologies . Powerpoint Presentation; Shuttleworth, Martyn. Descriptive Research Design , September 26, 2008. Explorable.com website.
A blueprint of the procedure that enables the researcher to maintain control over all factors that may affect the result of an experiment. In doing this, the researcher attempts to determine or predict what may occur. Experimental Research is often used where there is time priority in a causal relationship (cause precedes effect), there is consistency in a causal relationship (a cause will always lead to the same effect), and the magnitude of the correlation is great. The classic experimental design specifies an experimental group and a control group. The independent variable is administered to the experimental group and not to the control group, and both groups are measured on the same dependent variable. Subsequent experimental designs have used more groups and more measurements over longer periods. True experiments must have control, randomization, and manipulation.
- Experimental research allows the researcher to control the situation. In so doing, it allows researchers to answer the question, “what causes something to occur?”
- Permits the researcher to identify cause and effect relationships between variables and to distinguish placebo effects from treatment effects.
- Experimental research designs support the ability to limit alternative explanations and to infer direct causal relationships in the study.
- Approach provides the highest level of evidence for single studies.
- The design is artificial, and results may not generalize well to the real world.
- The artificial settings of experiments may alter subject behaviors or responses.
- Experimental designs can be costly if special equipment or facilities are needed.
- Some research problems cannot be studied using an experiment because of ethical or technical reasons.
- Difficult to apply ethnographic and other qualitative methods to experimental designed research studies.
Anastas, Jeane W. Research Design for Social Work and the Human Services . Chapter 7, Flexible Methods: Experimental Research. 2nd ed. New York: Columbia University Press, 1999; Chapter 2: Research Design, Experimental Designs . School of Psychology, University of New England, 2000; Experimental Research. Research Methods by Dummies. Department of Psychology. California State University, Fresno, 2006; Trochim, William M.K. Experimental Design . Research Methods Knowledge Base. 2006; Rasool, Shafqat. Experimental Research . Slideshare presentation.
An exploratory design is conducted about a research problem when there are few or no earlier studies to refer to. The focus is on gaining insights and familiarity for later investigation or undertaken when problems are in a preliminary stage of investigation.
The goals of exploratory research are intended to produce the following possible insights:
- Familiarity with basic details, settings and concerns.
- Well grounded picture of the situation being developed.
- Generation of new ideas and assumption, development of tentative theories or hypotheses.
- Determination about whether a study is feasible in the future.
- Issues get refined for more systematic investigation and formulation of new research questions.
- Direction for future research and techniques get developed.
- Design is a useful approach for gaining background information on a particular topic.
- Exploratory research is flexible and can address research questions of all types (what, why, how).
- Provides an opportunity to define new terms and clarify existing concepts.
- Exploratory research is often used to generate formal hypotheses and develop more precise research problems.
- Exploratory studies help establish research priorities.
- Exploratory research generally utilizes small sample sizes and, thus, findings are typically not generalizable to the population at large.
- The exploratory nature of the research inhibits an ability to make definitive conclusions about the findings.
- The research process underpinning exploratory studies is flexible but often unstructured, leading to only tentative results that have limited value in decision-making.
- Design lacks rigorous standards applied to methods of data gathering and analysis because one of the areas for exploration could be to determine what method or methodologies could best fit the research problem.
Cuthill, Michael. “Exploratory Research: Citizen Participation, Local Government, and Sustainable Development in Australia.” Sustainable Development 10 (2002): 79-89; Taylor, P. J., G. Catalano, and D.R.F. Walker. “Exploratory Analysis of the World City Network.” Urban Studies 39 (December 2002): 2377-2394; Exploratory Research . Wikipedia.
The purpose of a historical research design is to collect, verify, and synthesize evidence from the past to establish facts that defend or refute your hypothesis. It uses secondary sources and a variety of primary documentary evidence, such as, logs, diaries, official records, reports, archives, and non-textual information [maps, pictures, audio and visual recordings]. The limitation is that the sources must be both authentic and valid.
- The historical research design is unobtrusive; the act of research does not affect the results of the study.
- The historical approach is well suited for trend analysis.
- Historical records can add important contextual background required to more fully understand and interpret a research problem.
- There is no possibility of researcher-subject interaction that could affect the findings.
- Historical sources can be used over and over to study different research problems or to replicate a previous study.
- The ability to fulfill the aims of your research are directly related to the amount and quality of documentation available to understand the research problem.
- Since historical research relies on data from the past, there is no way to manipulate it to control for contemporary contexts.
- Interpreting historical sources can be very time consuming.
- The sources of historical materials must be archived consistentally to ensure access.
- Original authors bring their own perspectives and biases to the interpretation of past events and these biases are more difficult to ascertain in historical resources.
- Due to the lack of control over external variables, historical research is very weak with regard to the demands of internal validity.
- It rare that the entirety of historical documentation needed to fully address a research problem is available for interpretation, therefore, gaps need to be acknowledged.
Savitt, Ronald. “Historical Research in Marketing.” Journal of Marketing 44 (Autumn, 1980): 52-58; Gall, Meredith. Educational Research: An Introduction . Chapter 16, Historical Research. 8th ed. Boston, MA: Pearson/Allyn and Bacon, 2007.
A longitudinal study follows the same sample over time and makes repeated observations. With longitudinal surveys, for example, the same group of people is interviewed at regular intervals, enabling researchers to track changes over time and to relate them to variables that might explain why the changes occur. Longitudinal research designs describe patterns of change and help establish the direction and magnitude of causal relationships. Measurements are taken on each variable over two or more distinct time periods. This allows the researcher to measure change in variables over time. It is a type of observational study and is sometimes referred to as a panel study.
- Longitudinal data allow the analysis of duration of a particular phenomenon.
- Enables survey researchers to get close to the kinds of causal explanations usually attainable only with experiments.
- The design permits the measurement of differences or change in a variable from one period to another [i.e., the description of patterns of change over time].
- Longitudinal studies facilitate the prediction of future outcomes based upon earlier factors.
- The data collection method may change over time.
- Maintaining the integrity of the original sample can be difficult over an extended period of time.
- It can be difficult to show more than one variable at a time.
- This design often needs qualitative research to explain fluctuations in the data.
- A longitudinal research design assumes present trends will continue unchanged.
- It can take a long period of time to gather results.
- There is a need to have a large sample size and accurate sampling to reach representativness.
Anastas, Jeane W. Research Design for Social Work and the Human Services . Chapter 6, Flexible Methods: Relational and Longitudinal Research. 2nd ed. New York: Columbia University Press, 1999; Kalaian, Sema A. and Rafa M. Kasim. "Longitudinal Studies." In Encyclopedia of Survey Research Methods . Paul J. Lavrakas, ed. (Thousand Oaks, CA: Sage, 2008), pp. 440-441; Ployhart, Robert E. and Robert J. Vandenberg. "Longitudinal Research: The Theory, Design, and Analysis of Change.” Journal of Management 36 (January 2010): 94-120; Longitudinal Study . Wikipedia.
This type of research design draws a conclusion by comparing subjects against a control group, in cases where the researcher has no control over the experiment. There are two general types of observational designs. In direct observations, people know that you are watching them. Unobtrusive measures involve any method for studying behavior where individuals do not know they are being observed. An observational study allows a useful insight into a phenomenon and avoids the ethical and practical difficulties of setting up a large and cumbersome research project.
- Observational studies are usually flexible and do not necessarily need to be structured around a hypothesis about what you expect to observe (data is emergent rather than pre-existing).
- The researcher is able to collect a depth of information about a particular behavior.
- Can reveal interrelationships among multifaceted dimensions of group interactions.
- You can generalize your results to real life situations.
- Observational research is useful for discovering what variables may be important before applying other methods like experiments.
- Observation researchd esigns account for the complexity of group behaviors.
- Reliability of data is low because seeing behaviors occur over and over again may be a time consuming task and difficult to replicate.
- In observational research, findings may only reflect a unique sample population and, thus, cannot be generalized to other groups.
- There can be problems with bias as the researcher may only "see what they want to see."
- There is no possiblility to determine "cause and effect" relationships since nothing is manipulated.
- Sources or subjects may not all be equally credible.
- Any group that is studied is altered to some degree by the very presence of the researcher, therefore, skewing to some degree any data collected (the Heisenburg Uncertainty Principle).
Atkinson, Paul and Martyn Hammersley. “Ethnography and Participant Observation.” In Handbook of Qualitative Research . Norman K. Denzin and Yvonna S. Lincoln, eds. (Thousand Oaks, CA: Sage, 1994), pp. 248-261; Observational Research. Research Methods by Dummies. Department of Psychology. California State University, Fresno, 2006; Patton Michael Quinn. Qualitiative Research and Evaluation Methods . Chapter 6, Fieldwork Strategies and Observational Methods. 3rd ed. Thousand Oaks, CA: Sage, 2002; Rosenbaum, Paul R. Design of Observational Studies . New York: Springer, 2010.
Understood more as an broad approach to examining a research problem than a methodological design, philosophical analysis and argumentation is intended to challenge deeply embedded, often intractable, assumptions underpinning an area of study. This approach uses the tools of argumentation derived from philosophical traditions, concepts, models, and theories to critically explore and challenge, for example, the relevance of logic and evidence in academic debates, to analyze arguments about fundamental issues, or to discuss the root of existing discourse about a research problem. These overarching tools of analysis can be framed in three ways:
- Ontology -- the study that describes the nature of reality; for example, what is real and what is not, what is fundamental and what is derivative?
- Epistemology -- the study that explores the nature of knowledge; for example, on what does knowledge and understanding depend upon and how can we be certain of what we know?
- Axiology -- the study of values; for example, what values does an individual or group hold and why? How are values related to interest, desire, will, experience, and means-to-end? And, what is the difference between a matter of fact and a matter of value?
- Can provide a basis for applying ethical decision-making to practice.
- Functions as a means of gaining greater self-understanding and self-knowledge about the purposes of research.
- Brings clarity to general guiding practices and principles of an individual or group.
- Philosophy informs methodology.
- Refine concepts and theories that are invoked in relatively unreflective modes of thought and discourse.
- Beyond methodology, philosophy also informs critical thinking about epistemology and the structure of reality (metaphysics).
- Offers clarity and definition to the practical and theoretical uses of terms, concepts, and ideas.
- Limited application to specific research problems [answering the "So What?" question in social science research].
- Analysis can be abstract, argumentative, and limited in its practical application to real-life issues.
- While a philosophical analysis may render problematic that which was once simple or taken-for-granted, the writing can be dense and subject to unnecessary jargon, overstatement, and/or excessive quotation and documentation.
- There are limitations in the use of metaphor as a vehicle of philosophical analysis.
- There can be analytical difficulties in moving from philosophy to advocacy and between abstract thought and application to the phenomenal world.
Chapter 4, Research Methodology and Design . Unisa Institutional Repository (UnisaIR), University of South Africa; Labaree, Robert V. and Ross Scimeca. “The Philosophical Problem of Truth in Librarianship.” The Library Quarterly 78 (January 2008): 43-70; Maykut, Pamela S. Beginning Qualitative Research: A Philosophic and Practical Guide . Washington, D.C.: Falmer Press, 1994; Stanford Encyclopedia of Philosophy . Metaphysics Research Lab, CSLI, Stanford University, 2013.
- The researcher has a limitless option when it comes to sample size and the sampling schedule.
- Due to the repetitive nature of this research design, minor changes and adjustments can be done during the initial parts of the study to correct and hone the research method. Useful design for exploratory studies.
- There is very little effort on the part of the researcher when performing this technique. It is generally not expensive, time consuming, or workforce extensive.
- Because the study is conducted serially, the results of one sample are known before the next sample is taken and analyzed.
- The sampling method is not representative of the entire population. The only possibility of approaching representativeness is when the researcher chooses to use a very large sample size significant enough to represent a significant portion of the entire population. In this case, moving on to study a second or more sample can be difficult.
- Because the sampling technique is not randomized, the design cannot be used to create conclusions and interpretations that pertain to an entire population. Generalizability from findings is limited.
- Difficult to account for and interpret variation from one sample to another over time, particularly when using qualitative methods of data collection.
Rebecca Betensky, Harvard University, Course Lecture Note slides ; Cresswell, John W. Et al. “Advanced Mixed-Methods Research Designs.” In Handbook of Mixed Methods in Social and Behavioral Research . Abbas Tashakkori and Charles Teddle, eds. (Thousand Oaks, CA: Sage, 2003), pp. 209-240; Nataliya V. Ivankova. “Using Mixed-Methods Sequential Explanatory Design: From Theory to Practice.” Field Methods 18 (February 2006): 3-20; Bovaird, James A. and Kevin A. Kupzyk. “Sequential Design.” In Encyclopedia of Research Design . Neil J. Salkind, ed. Thousand Oaks, CA: Sage, 2010; Sequential Analysis . Wikipedia.
- << Previous: Purpose of Guide
- Next: Design Flaws to Avoid >>
- Last Updated: Jul 18, 2023 11:58 AM
- URL: https://library.sacredheart.edu/c.php?g=29803
- QuickSearch
- Library Catalog
- Databases A-Z
- Publication Finder
- Course Reserves
- Citation Linker
- Digital Commons
- Our Website
Research Support
- Ask a Librarian
- Appointments
- Interlibrary Loan (ILL)
- Research Guides
- Databases by Subject
- Citation Help
Using the Library
- Reserve a Group Study Room
- Renew Books
- Honors Study Rooms
- Off-Campus Access
- Library Policies
- Library Technology
User Information
- Grad Students
- Online Students
- COVID-19 Updates
- Staff Directory
- News & Announcements
- Library Newsletter
My Accounts
- Interlibrary Loan
- Staff Site Login

FIND US ON
Skip to Content
Research in design entails the systematic study of the artifact creation process and its integration into various environments — including virtual, physical, social, psychological, economic and political. This focus area aims to enhance the practice of design engineering. It involves deepening our understanding of both the designers themselves and the users of their creations; the methodologies and techniques employed in the design process; and the broader societal impact of engineered solutions and outcomes.
Strength Areas in Design in Mechanical Engineering
- Design Theory and Methodology: Underlying science of processes that designers use to develop engineering solutions.
- Computational Design and Optimization: Development and use of technology to visualize, analyze, and make decisions during design processes.
- Systems Engineering: Multi-disciplinary approaches for the design and operation of complex systems within their broader socio-technical environments.
- Engineering for Sustainable Development: Development of design methods and processes to meet the needs of the present without compromising the ability of future generations to meet their own needs.
- Design for Manufacturing: Methodologies and processes for designing parts, components, and products for efficient, cost-effective, and sustainable manufacturing and product realization.
- Inclusive & Human-Centered Design: Methodologies and processes that place stakeholders at the heart of the design process to create solutions that understand and enable people of all backgrounds.
- Bio-Inspired Design: The process of learning from nature to inspire strategies for innovation, including principles of form, function, performance, and aesthetics.
- Design Education Research: Development and analysis of epistemology, policy, and assessment of engineering education and design skill development, including K-12, higher education, and post-education.
Associated Faculty
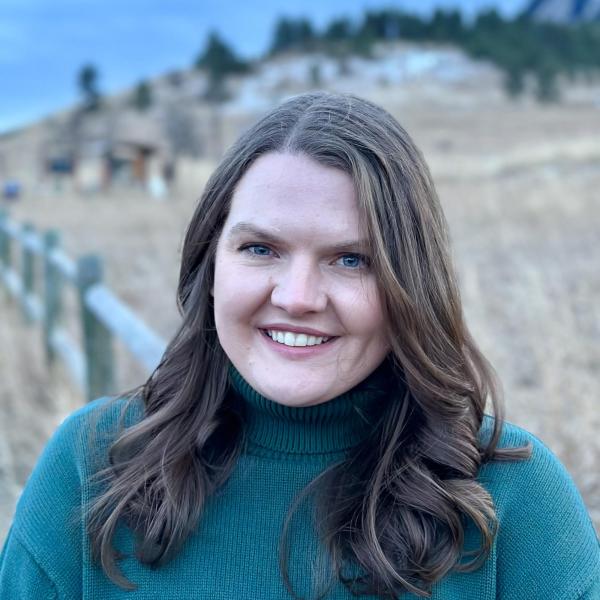
- Share via Facebook
- Share via Twitter
- Share via LinkedIn
- Air Quality Research
- Biomedical Research
- Materials Research
- Mechanics of Materials Research
- Micro/Nanoscale Research
- Robotics and Systems Design Research
- Thermo Fluid Sciences Research
- Design Engineering
- Research Facilities & Centers
Apply Visit Give
Departments
- Ann and H.J. Smead Aerospace Engineering Sciences
- Chemical & Biological Engineering
- Civil, Environmental & Architectural Engineering
- Computer Science
- Electrical, Computer & Energy Engineering
- Paul M. Rady Mechanical Engineering
- Applied Mathematics
- Biomedical Engineering
- Creative Technology & Design
- Engineering Education
- Engineering Management
- Engineering Physics
- Integrated Design Engineering
- Environmental Engineering
- Materials Science & Engineering
Affiliates & Partners
- ATLAS Institute
- BOLD Center
- Colorado Mesa University
- Colorado Space Grant Consortium
- Discovery Learning
- Engineering Honors
- Engineering Leadership
- Entrepreneurship
- Herbst Program for Engineering, Ethics & Society
- Integrated Teaching and Learning
- Global Engineering
- National Center for Women & Information Technology
- Mortenson Center for Global Engineering
- Western Colorado University
Thank you for visiting nature.com. You are using a browser version with limited support for CSS. To obtain the best experience, we recommend you use a more up to date browser (or turn off compatibility mode in Internet Explorer). In the meantime, to ensure continued support, we are displaying the site without styles and JavaScript.
- View all journals
- Explore content
- About the journal
- Publish with us
- Sign up for alerts
- Review Article
- Open access
- Published: 08 August 2024
Research progress and intellectual structure of design for digital equity (DDE): A bibliometric analysis based on citespace
- Baoyi Zhang ORCID: orcid.org/0000-0003-4479-7587 1
Humanities and Social Sciences Communications volume 11 , Article number: 1019 ( 2024 ) Cite this article
394 Accesses
Metrics details
- Cultural and media studies
- Science, technology and society
Digital equity is imperative for realizing the Sustainable Development Goals, particularly SDG9 and SDG10. Recent empirical studies indicate that Design for Digital Equity (DDE) is an effective strategy for achieving digital equity. However, before this review, the overall academic landscape of DDE remained obscure, marked by substantial knowledge gaps. This review employs a rigorous bibliometric methodology to analyze 1705 DDE-related publications, aiming to delineate DDE’s research progress and intellectual structure and identify research opportunities. The retrieval strategy was formulated based on the PICo framework, with the process adhering to the PRISMA systematic review framework to ensure transparency and replicability of the research method. CiteSpace was utilized to visually present the analysis results, including co-occurrences of countries, institutions, authors, keywords, emerging trends, clustering, timeline analyses, and dual-map overlays of publications. The results reveal eight significant DDE clusters closely related to user-centered design, assistive technology, digital health, mobile devices, evidence-based practices, and independent living. A comprehensive intellectual structure of DDE was constructed based on the literature and research findings. The current research interest in DDE lies in evidence-based co-design practices, design issues in digital mental health, acceptance and humanization of digital technologies, digital design for visually impaired students, and intergenerational relationships. Future research opportunities are identified in DDE’s emotional, cultural, and fashion aspects; acceptance of multimodal, tangible, and natural interaction technologies; needs and preferences of marginalized groups in developing countries and among minority populations; and broader interdisciplinary research. This study unveils the multi-dimensional and inclusive nature of methodological, technological, and user issues in DDE research. These insights offer valuable guidance for policy-making, educational strategies, and the development of inclusive digital technologies, charting a clear direction for future research.
Similar content being viewed by others
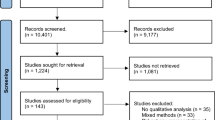
Recommendations to advance digital health equity: a systematic review of qualitative studies

Design, content validity, and inter-observer reliability of the ‘Digitization of Cultural Heritage, Identities, and Education’ (DICHIE) instrument

Digital competence in adolescents and young adults: a critical analysis of concomitant variables, methodologies and intervention strategies
Introduction.
Digital equity has emerged as a critical factor in achieving the Sustainable Development Goals (SDGs), especially SDG9 (Industry, Innovation, and Infrastructure) and SDG10 (Reduced Inequalities) (United Nations, 2021 ; UNSD 2023 ), amidst the rapid evolution of digital technologies. In our increasingly digitalized society, these technologies amplify and transform existing social inequalities while offering numerous benefits, leading to more significant disparities in access and utilization (Grybauskas et al., 2022 ). This situation highlights the critical need for strategies that promote equitable digital participation, ensuring alignment with the overarching objectives of the SDGs. Digital equity, a multi-faceted issue, involves aspects such as the influence of cultural values on digital access (Yuen et al., 2017 ), the challenges and opportunities of technology in higher education (Willems et al., 2019 ), and the vital role of government policies in shaping digital divides (King & Gonzales, 2023 ), and the impact on healthcare access and delivery (Lawson et al., 2023 ). Equally important are the socioeconomic factors that intersect with digital equity (Singh, 2017 ) and the pressing need for accessible digital technologies for disabled individuals (Park et al., 2019 ). These issues are observed globally, necessitating diverse and inclusive strategies.
Design thinking, in addressing issues of social equality and accessibility, plays an essential role in accessibility (Persson et al., 2015 ; Dragicevic et al., 2023a ); in other words, it serves as a crucial strategy for reducing social inequality. Indeed, design strategies focused on social equality, also known as Equity-Centered Design (Oliveri et al., 2020 ; Bazzano et al., 2023 ), are diverse, including universal design (Mace ( 1985 )), Barrier-free design (Cooper et al., 1991 ), inclusive design (John Clarkson, Coleman ( 2015 )), and Design for All (Bendixen & Benktzon, 2015 ). Stanford d.school has further developed the Equity-Centered Design Framework based on its design thinking model (Stanford d.school, 2016 ) to foster empathy and self-awareness among designers in promoting equality. Equity-centered approaches are also a hot topic in academia, especially in areas like education (Firestone et al., 2023 ) and healthcare (Rodriguez et al., 2023 ). While these design approaches may have distinct features and positions due to their developmental stages, national and cultural contexts, and the issues they address, Equity-Centered Design consistently plays a vital role in achieving the goal of creating accessible environments and products, making them accessible and usable by individuals with various abilities or backgrounds (Persson et al., 2015 ).
Equity-centered design initially encompassed various non-digital products, but with the rapid advancement of digitalization, it has become increasingly critical to ensure that digital technologies are accessible and equitable for all users. This can be referred to as Design for Digital Equity (DDE). However, the current landscape reveals a significant gap in comprehensive research focused on Design for Digital Equity (DDE). This gap highlights the need for more focused research and development in this area, where bibliometrics can play a significant role. Through systematic reviews and visualizations, bibliometric analysis can provide insights into this field’s intellectual structure, informing and guiding future research directions in digital equity and design.
Bibliometrics, a term first coined by Pritchard in 1969 (Broadus, 1987 ), has evolved into an indispensable quantitative tool for analyzing scholarly publications across many research fields. This method, rooted in the statistical analysis of written communication, has significantly enhanced our understanding of academic trends and patterns. Its application spans environmental studies (Wang et al., 2021 ), economics (Qin et al., 2021 ), big data (Ahmad et al., 2020 ), energy (Xiao et al., 2021 ), medical research (Ismail & Saqr, 2022 ) and technology acceptance (Wang et al., 2022 ). By distilling complex publication data into comprehensible trends and patterns, bibliometrics has become a key instrument in shaping our understanding of the academic landscape and guiding future research directions.
In bibliometrics, commonly used tools such as CiteSpace (Chen, 2006 ), VOSviewer (Van Eck, Waltman ( 2010 )), and HistCite (Garfield, 2009 ) are integral for advancing co-citation analysis and data visualization. Among these, CiteSpace, developed by Professor Chen (Chen, 2006 ), is a Java-based tool pivotal in advancing co-citation analysis for data visualization and analysis. Renowned for its integration of burst detection, betweenness centrality, and heterogeneous network analysis, it is essential in identifying research frontiers and tracking trends across various domains. Chen demonstrates the versatility of CiteSpace in various fields, ranging from regenerative medicine to scientific literature, showcasing its proficiency in extracting complex insights from data sets (Chen, 2006 ). Its structured methodology, encompassing time slicing, thresholding, and more, facilitates comprehensive analysis of co-citations and keywords. This enhances not only the analytical capabilities of CiteSpace but also helps researchers comprehend trends within specific domains. (Chen et al. 2012 ; Ping et al. 2017 ). Therefore, CiteSpace is a precious tool in academic research, particularly for disciplines that require in-depth analysis of evolving trends and patterns.
After acknowledging the significance of DDE in the rapidly evolving digital environment, it becomes imperative to explore the academic contours of this field to bridge knowledge gaps, a critical prerequisite for addressing social inequalities within digital technology development. We aim to scrutinize DDE’s research progress and intellectual structure, analyzing a broad spectrum of literature with the aid of bibliometric and CiteSpace methodologies. Accordingly, four research questions (RQs) have been identified to guide this investigation. The detailed research questions are as follows:
RQ1: What are the trends in publications in the DDE field from 1995 to 2023?
RQ2: Who are the main contributors, and what are the collaboration patterns in DDE research?
RQ3: What are the current research hotspots in DDE?
RQ4: What is the intellectual structure and future trajectory of DDE?
The remainder of this paper is structured as follows: The Methods section explains our bibliometric approach and data collection for DDE research. The Results section details our findings on publication trends and collaborative networks, addressing RQ1 and RQ2. The Discussion section delves into RQ3 and RQ4, exploring research hotspots and the intellectual structure of DDE. The Conclusion section summarizes our study’s key insights.
In this article, the systematic review of DDE follows the PRISMA (Preferred Reporting Items for Systematic Reviews and Meta-Analyses) guidelines (PRISMA 2023 ), which are evidence-based reporting guidelines for systematic review reports (Moher et al., 2010 ). PRISMA was developed to enhance the quality of systematic reviews and enhance the clarity and transparency of research findings (Liberati et al., 2009 ). To achieve this goal, the research workflow in this study incorporates an online tool based on the R package from PRISMA 2020. This tool enables researchers to rapidly generate flowcharts that adhere to the latest updates in the PRISMA statement, ensuring transparency and reproducibility in the research process. This workflow comprises three major stages: Identification, Screening, and Inclusion, as illustrated in Fig. 1 .

PRISMA flowchart for the DDE systematic review.
Additionally, to obtain high-quality data sources, the Web of Science (referred to as WOS), provided by Clarivate Analytics, was chosen. WOS is typically considered the optimal data source for bibliometric research (van Leeuwen, 2006 ). The WOS Core Collection comprises over 21,000 peer-reviewed publications spanning 254 subject categories and 1.9 billion cited references, with the earliest records traceable back to 1900 (Clarivate, 2023 ). To thoroughly explore the research on DDE, this review utilized all databases within the WOS Core Collection as the source for data retrieval.
Search strategy
Developing a rational and effective search strategy is crucial for systematic reviews (Cooper et al., 2018 ), typically necessitating a structured framework to guide the process (Sayers, 2008 ). This approach ensures comprehensive and relevant literature coverage. To comprehensively and accurately assess the current state and development of “Design for Digital Equity,” this paper employs the PICo (participants, phenomena of interest, and context) model as its search strategy, a framework typically used for identifying research questions in systematic reviews (Stern et al., 2014 ). While the PICo framework is predominantly utilized within clinical settings for systematic reviews, its structured approach to formulating research questions and search strategies is equally applicable across many disciplines beyond the clinical environment. This adaptability makes it a suitable choice for exploring the multi-faceted aspects of digital equity in a non-clinical context (Nishikawa-Pacher, 2022 ).
This review, structured around the PICo framework, sets three key concepts (search term groups): Participants (P): any potential digital users; Phenomena of Interest (I): equity-centered design; Context (Co): digital equity. To explore the development and trends of DDE comprehensively, various forms of search terms are included in each PICo element. The determination of search terms is a two-stage process. In the first stage, core terms of critical concepts like equity-centered design, digital equity, and Design for Digital Equity, along with their synonyms, different spellings, and acronyms, are included in the list of candidate search terms. Wildcards (*) are used to expand the search range to ensure the inclusion of all variants and derivatives of critical terms, thus enhancing the thoroughness and depth of the search. However, studies have indicated the challenge of identifying semantically unrelated terms relevant to the research (Chen, 2018 ). To address this issue, the second phase of developing the search strategy involves reading domain-specific literature reviews using these core terms. This literature-based discovery (LBD) approach can identify hidden, previously unknown relationships, finding significant connections between different kinds of literature (Kastrin & Hristovski, 2021 ). The candidate word list is then reviewed, refined, or expanded by domain experts. Finally, a search string (Table 1 ) is constructed with all search terms under each search term group linked by the Boolean OR (this term or that term), and the Boolean links each group AND (this group of terms and that group of terms).
Inclusion criteria
Following the PRISMA process (Fig. 1 ), literature in the identification phase was filtered using automated tools based on publication data attributes such as titles, subjects, and full texts or specific criteria like publication names, publication time ranges, and types of publication sources. Given the necessity for a systematic and extensive exploration of DDE research, this review employed an advanced search using “ALL” instead of “topic” or “Title” in the search string to ensure a broader inclusion of results. No limitations were set on other attributes of the literature. The literature search was conducted on December 5, 2023, resulting in 1747 publications exported in Excel for further screening.
During the literature screening phase, the authors reviewed titles and abstracts, excluding 11 publications unrelated to DDE research. Three papers were inaccessible in the full-text acquisition phase. The remaining 1729 publications were then subjected to full-text review based on the following inclusion and exclusion criteria. Eventually, 1705 papers meeting the criteria were imported into CiteSpace for analysis.
Papers were included in this review if they met the following criteria:
They encompassed all three elements of PICo: stakeholders or target users of DDE, design relevance, and digitalization aspects.
They had transparent research methodologies, whether empirical or review studies employing qualitative, quantitative, or mixed methods.
They were written in English.
Papers were excluded if they:
Focused solely on digital technology, unrelated to design, human, and social factors.
Contained terms with identical acronyms but different meanings, e.g., ICT stands for Inflammation of connective tissue in medicine.
Were unrelated to topics of social equality.
Were in languages other than English.
Data analysis
To comprehensively address Research Question 1: “What are the publication trends in the DDE field from 1995 to 2023?” this study utilized CiteSpace to generate annual trend line graphs for descriptive analysis. This analysis revealed the annual development trends within the DDE research field and identified vital research nodes and significant breakthroughs by analyzing the citation frequency of literature across different years. Utilizing the burst detection feature in CiteSpace, key research papers and themes were further identified, marking periods of significant increases in research activity. For Research Question 2: “Who are the main contributors to DDE research, and what are their collaboration patterns?” nodes for countries, institutions, cited authors, cited publications, and keywords were set up in CiteSpace for network analysis. Our complex network diagrams illustrate the collaboration relationships between different researchers and institutions, where the size of the nodes indicates the number of publications by each entity, and the thickness and color of the lines represent the strength and frequency of collaborations.
Additionally, critical scholars and publications that act as bridges within the DDE research network were identified through centrality analysis. In the keyword analysis, the focus was on co-occurrence, trend development, and clustering. Current research hotspots were revealed using the LSI algorithm in CiteSpace for cluster analysis, demonstrating how these hotspots have evolved over time through timeline techniques. A dual-map overlay analysis was used to reveal citation relationships between different disciplines, showcasing the interdisciplinary nature of DDE research. In the visual displays of CiteSpace, the visual attributes of nodes and links were meticulously designed to express the complex logical relationships within the data intuitively. The size of nodes typically reflects the publication volume or citation frequency of entities such as authors, institutions, countries, or keywords, with larger nodes indicating highly active or influential research focal points. The change in node color often represents the progress of research over time, with gradients from dark to light colors indicating the evolution from historical to current research. Whether solid or dashed, the outline of nodes differentiates mainstream research areas from marginal or emerging fields. The thickness and color of the lines reflect the strength of collaborations or frequency of citations, aiding in the identification of close collaborations or frequent citations. These design elements not only enhance the information hierarchy of the diagrams but also improve the usability and accuracy for users exploring and analyzing the data, effectively supporting researchers in understanding the structure and dynamics of the academic field. The subsequent research results section provides detailed descriptions for each visual element.
The first section of the Results primarily addresses RQ1: “What are the trends in publications in the DDE field from 1995 to 2023?” The subsequent sections collectively address RQ2: “Who are the main contributors, and what are the collaboration patterns in DDE research?”
Analysis of Publication Trends
Figure 2 , extracted from the WOS citation analysis report, delineates the progression of annual scholarly publications within the Design for Digital Equity field. This trend analysis resonates with de Solla Price’s model of scientific growth (Price 1963 ), beginning with a slow and steady phase before transitioning into a period of more rapid expansion. Notably, a pronounced spike in publications was observed following 2020, characterized by the global COVID-19 pandemic. This uptick indicates an acute scholarly response to the pandemic, likely propelled by the heightened need for digital equity solutions as the world adapted to unprecedented reliance on digital technologies for communication, work, and education amidst widespread lockdowns and social distancing measures. The graph presents a clear visualization of this scholarly reaction, with the peak in 2021 marking the zenith of research output, followed by a slight retraction, which may suggest a period of consolidation or a pivot towards new research frontiers in the post-pandemic era.
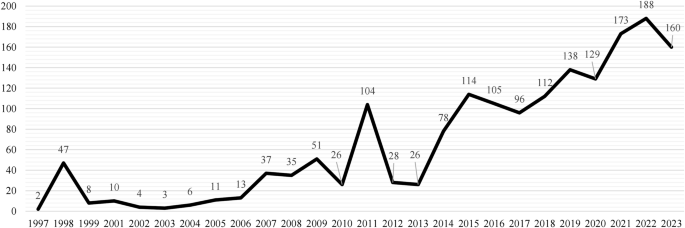
Trends in Scholarly Publications on Design for Digital Equity (1997–2023).
Visual analysis by countries or regions
Table 2 presents an illustrative overview of the diverse global contributions to research on “Design for Digital Equity,” including a breakdown of the number of publications, centrality, and the initial year of engagement for each participating country. The United States stands preeminent with 366 publications, affirming its central role in the domain since the mid-1990s. Despite fewer publications, the United Kingdom boasts the highest centrality, signaling its research as notably influential within the academic network since the late 1990s. Since China entered the DDE research arena in 2011, its publications have had explosive growth, reflecting rapid ascension and integration into the field. Furthermore, the extensive volume of publications from Canada and the notable centrality of Spain underscores their substantial and influential research endeavors. The table also recognizes the contributions from countries such as Germany, Italy, and Australia, each infusing unique strengths and perspectives into the evolution of DDE research.
Figure 3 , crafted within CiteSpace, delineates the collaborative contours of global research in Design for Digital Equity (DDE). Literature data are input with ‘country’ as the node type and annual segmentation for time slicing, employing the ‘Cosine’ algorithm to gauge the strength of links and the ‘g-index’ ( K = 25) for selection criteria. The visualization employs a color gradient to denote the years of publication, with the proximity of nodes and the thickness of the interconnecting links articulating the intensity and frequency of collaborative efforts among nations. For instance, the close-knit ties between the United States, Germany, and France underscore a robust tripartite research collaboration within the DDE domain. The size of the nodes corresponds directly to the proportion of DDE publications contributed by each country. Larger nodes, such as those representing the USA and Germany, suggest more publications, indicating significant research activity and influence within the field. Purple nodes, such as those representing England and Canada, signal a strong centrality within the network, suggesting these countries contribute significantly and play a pivotal role in disseminating research findings throughout the network. The intertwining links of varying thickness reveal the nuanced interplay of collaboration: dense webs around European countries, for instance, underscore a rich tradition of continental cooperation, while transatlantic links point to ongoing exchanges between North American and European researchers. Moreover, the appearance of vibrant links extending toward Asian countries such as China and South Korea reflects the expanding scope of DDE research to encompass a truly global perspective, integrating diverse methodologies and insights as the research community tackles the universal challenges of digital equity.
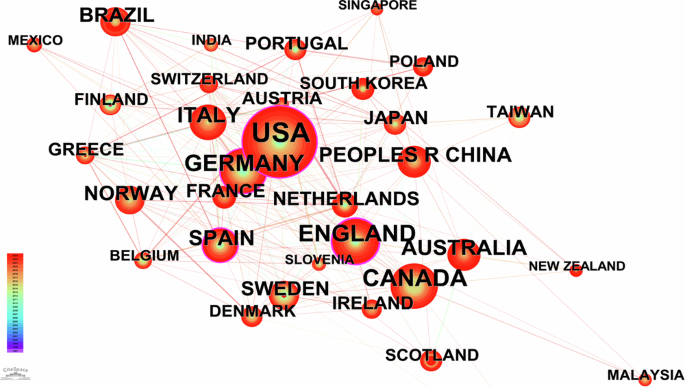
Collaborative networks between countries and regions in DDE research.
Visual analysis by institutions
Table 3 presents a quantified synopsis of institutional research productivity and centrality within the Design for Digital Equity field. The University of Toronto emerges as the most prolific contributor, with 64 publications and a centrality score of 0.06, indicating a significant impact on the field since 2008. The University System of Georgia and the Georgia Institute of Technology, each with 27 and 25 publications, respectively, registering a centrality of 0.01 since 2006, denoting their sustained scholarly activity over time. The Oslo Metropolitan University, with 23 publications and a centrality of 0.02 since 2016, and the Consiglio Nazionale delle Ricerche, with 17 publications since 2009, highlight the diverse international engagement in DDE research. The table also notes the early contributions of the Pennsylvania Commonwealth System of Higher Education, with 17 publications since 2004, although its centrality remains at 0.01. Institutions such as Laval University, Monash University, and the Polytechnic University of Milan show emergent centrality in the field, with recent increases in scholarly output, as indicated by their respective publication counts of 13, 12, and 12 since 2019, 2020, and 2018. This data evidences a dynamic and growing research domain characterized by historical depth and contemporary expansion.
Figure 4 displays a network map highlighting the collaborative landscape among institutions in the field of DDE. The University of Toronto commands a central node with a substantial size, indicating its leading volume of research output. The University of Alberta and CNR exhibit nodes colored to represent earlier works in the field, establishing their roles as foundational contributors. Inter-institutional links, albeit pleasing, are observable, suggesting research collaborations. Nodes such as the University of London and the Polytechnic University of Milan, while smaller, are nonetheless integral, denoting their active engagement in DDE research. The color coding of nodes corresponds to publication years, with warmer colors indicating more recent research, providing a temporal dimension to the map. This network visualization is an empirical tool to assess the scope and scale of institutional contributions and collaborations in DDE research.

Network Map of Institutional Collaboration in DDE.
Analysis by publications
Table 4 delineates the pivotal academic publications contributing to the field, as evidenced by citation count, centrality, and publication year, offering a longitudinal perspective of influence and relevance. ‘Lecture Notes in Computer Science’ leads the discourse with 354 citations and the highest centrality of 0.10 since 2004, indicating its foundational and central role over nearly two decades. This is followed by the ‘Journal of Medical Internet Research,’ with 216 citations since 2013 and centrality of 0.05, evidencing a robust impact in a shorter timeframe. The relationship between citation count and centrality reveals a pattern of influential cores within the field. Publications with higher citation counts generally exhibit greater centrality, suggesting that they are reference points within the academic network and instrumental in shaping the digital equity narrative. The thematic diversity of the publications—from technology-focused to health-oriented publications like ‘Computers in Human Behavior’ and ‘Disability and Rehabilitation’—reflects the interdisciplinary nature of research in digital equity, encompassing a range of issues from technological access to health disparities. ‘CoDesign,’ despite its lower position with 101 citations since 2016 and centrality of 0.01, represents the burgeoning interest in participatory design practices within the field. Its presence underscores the evolving recognition of collaborative design processes as essential to achieving digital equity, particularly in the later years where user-centered design principles are increasingly deemed critical for inclusivity in digital environments.
Visual analysis by authors
Table 5 enumerates the most influential authors in the domain of DDE research, ranked by citation count and centrality within the academic network from the year of their first cited work. The table is led by Braun V., with a citation count of 103 and a centrality of 0.13 since 2015, indicating a strong influence in the recent scholarly conversation on DDE. Close behind, the World Health Organization (WHO), with 97 citations and a centrality of 0.10 since 2012, and Nielsen J., with an impressive centrality of 0.32 and 89 citations since 1999, denote long-standing and significant contributions to the field. The high centrality scores, remarkably Nielsen’s, suggest these authors’ works are central nodes in the network of citations, acting as crucial reference points for subsequent research. Further down the list, authors such as Davis F.D. and Venkatesh V. are notable for their scholarly impact, with citation counts of 74 and 59, respectively, and corresponding centrality measures that reflect their substantial roles in shaping DDE discourse. The table also recognizes the contributions of authoritative entities like the United Nations and the World Health Organization, reflecting digital equity research’s global and policy-oriented dimensions. The presence of ISO in the table, with a citation count of 25 since 2015, underscores the importance of standardization in the digital equity landscape. The diversity in authors and entities—from individual researchers to global organizations—highlights the multi-faceted nature of research in DDE, encompassing technical, social, and policy-related studies.
Figure 5 illustrates the collaborative network between cited authors in the DDE study. The left side of the network map is characterized by authors with cooler-colored nodes, indicating earlier contributions to digital equity research. Among these, Wright Ronald stands out with a significantly large node and a purple outline, highlighting his seminal role and the exceptional citation burst in his work. Cool colors suggest these authors laid the groundwork for subsequent research, with their foundational ideas and theories continuing to be pivotal in the field. Transitioning across the network to the right, a gradual shift to warmer node colors is observed, representing more recent contributions to the field. Here, the nodes increase in size, notably for authors such as Braun V. and the WHO, indicating a high volume of publications and a more contemporary impact on the field. The links between these recent large nodes and the earlier contributors, such as Wright Ronald, illustrate a scholarly lineage and intellectual progression within the research community. The authors with purple outlines on the right side of the map indicate recent citation bursts, signifying that their work has quickly become influential in the academic discourse of digital equity research. These bursts are likely a response to the evolution of digital technologies and the emerging challenges of equality within the digital space.
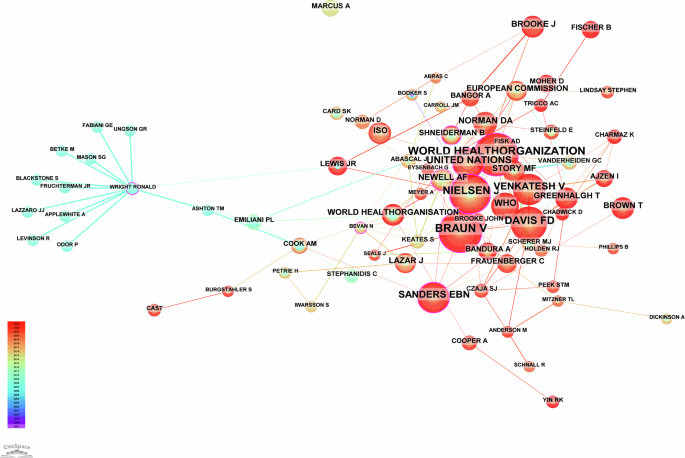
Collaborative networks of globally cited authors in DDE research.
Visual analysis by keywords
The concurrent keywords reflect the research hotspots in the field of DDE. Table 6 presents the top 30 keywords with the highest frequency and centrality, while Fig. 6 shows the co-occurrence network of these keywords. Within the purview of Fig. 6 , the visualization elucidates the developmental trajectory of pivotal terms in the digital equity research domain. The nodes corresponding to ‘universal design,’ ‘assistive technology,’ and ‘user-centered design’ are characterized by lighter centers within their larger structures, signifying an established presence and a maturation over time within scholarly research. The robust, blue-hued link connecting ‘universal design’ and ‘assistive technology’ underscores these foundational concepts’ strong and historical interrelation. The nodes encircled by purple outlines, such as ‘universal design,’ ‘inclusive design,’ and ‘participatory design,’ denote a high degree of centrality. This indicates their role as critical junctions within the research network, reflecting a widespread citation across diverse studies and underscoring their integral position within the thematic constellation of the field. Of particular note are the nodes with red cores, such as ‘design for all,’ ‘digital health,’ ‘visual impairment,’ ‘mobile phone,’ and ‘digital divide.’ These nodes signal emergent focal points of research, indicating recent academic interest and citation frequency surges. Such bursts are emblematic of the field’s dynamic nature, pointing to evolving hotspots of scholarly investigation. For instance, the red core of ‘digital health’ suggests an intensifying dialogue around integrating digital technology in health-related contexts, a pertinent issue in modern discourse.
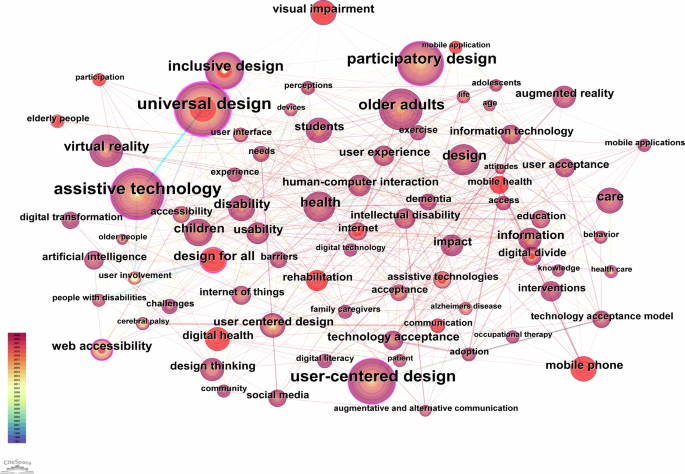
Keyword co-occurrence networks in the DDE domain.
Building upon the highlighted red-core nodes denoting keyword bursts in Figs. 6 , 7 , “Top 17 Keywords with the Strongest Citation Bursts in DDE,” offers a quantified analysis of such emergent trends. This figure tabulates the keywords that have experienced the most significant surges in academic citations within the field of DDE from 1997 to 2023. Keywords such as ‘design for all’ and ‘universal design’ anchor the list, showcasing their foundational bursts starting from 1997, with ‘design for all’ maintaining a high citation strength of 20.66 until 2015 and ‘universal design’ demonstrating enduring relevance through 2016. This signifies the long-standing and evolving discourse surrounding these concepts. In contrast, terms like ‘mobile phone,’ ‘digital health,’ and ‘participation’ represent the newest fronts in DDE research, with citation bursts emerging as late as 2020 and 2021, reflecting the rapid ascent of these topics in the recent scholarly landscape. The strength of these bursts, particularly the 7.07 for ‘mobile phone,’ suggests a burgeoning field of study responsive to technological advancements and societal shifts. The bar graph component of the figure visually represents the duration of each burst, with red bars marking the start and end years. The length and position of these bars corroborate the temporal analysis, mapping the lifecycle of each keyword’s impact.

Top 17 Keywords with the Strongest Citation Bursts in DDE.
The authors have conducted a keyword clustering analysis on the data presented in Fig. 6 , aiming to discern the interrelationships between keywords and delineate structured knowledge domains within the field of DDE. Utilizing the Latent Semantic Indexing (LSI) algorithm to derive the labeling of clusters, they have effectively crystallized seven distinct clusters in DDE research, as depicted in Fig. 8 . The cluster represented in red, labeled ‘#0 universal design,’ signifies a group of closely related concepts that have been pivotal in discussions on making design accessible to all users. This cluster’s central placement within the figure suggests its foundational role in DDE. Adjacent to this, in a lighter shade of orange, is the ‘#1 user-centered design’ cluster, indicating a slightly different but related set of terms emphasizing the importance of designing with the end-user’s needs and experiences in mind. The ‘#2 assistive technology’ cluster, shown in yellow, groups terms around technologies designed to aid individuals with disabilities, signifying its specialized yet crucial role in promoting digital equity. Notably, the #3 digital health cluster in green and the #4 mobile phone cluster in turquoise highlight the intersection of digital technology with health and mobile communication, illustrating the field’s expansion into these dynamic research areas. The ‘#6 participatory design’ cluster in purple and ‘#7 independent living’ cluster in pink emphasize collaboration in design processes and the empowerment of individuals to live independently, respectively.

Keyword clustering analysis map for DDE research.
In addition, the timeline function in CiteSpace was used to present the seven clusters in Fig. 8 and the core keywords they contain (the threshold for Label was set to 6) annually, as shown in Fig. 9 . The timeline graph delves deeper into the clusters’ developmental stages and interconnections of keywords. In the #0 universal design cluster, the term ‘universal design’ dates back to 1997, alongside ‘assistive technology,’ ‘user participation,’ and ‘PWDs,’ which together marked the inception phase of DDE research within the universal design cluster, where the focus was on creating accessible environments and products for the broadest possible audience. With the advancement of digital technologies, terms like ‘artificial intelligence’ in 2015, ‘digital accessibility’ in 2018, and the more recent ‘students with disabilities’ have emerged as new topics within this cluster. Along with #0 universal design, the #6 participatory design cluster has a similarly lengthy history, with terms like ‘computer access’ and ‘design process’ highlighting the significance of digital design within this cluster. Moreover, within this timeline network, many terms are attributed to specific populations, such as ‘PWDs,’ ‘children,’ ‘aging users,’ ‘adults,’ ‘students,’ ‘blind people,’ ‘stroke patients,’ ‘family caregivers,’ ‘persons with mild cognitive impairments,’ ‘active aging,’ and ‘students with disabilities,’ revealing the user groups that DDE research needs to pay special attention to, mainly the recent focus on ‘mild cognitive impairments’ and ‘students with disabilities,’ which reflect emerging issues. Then, the particularly dense links in the graph hint at the correlations between keywords; for instance, ‘children’ and ‘affective computing’ within the #6 participatory design cluster are strongly related, and the latest terms ‘education’ and ‘autism spectrum disorder occupational therapy’ are strongly related, revealing specific issues within the important topic of education in DDE research. Other nodes with dense links include ‘digital divide,’ ‘user acceptance,’ ‘social participation,’ ‘interventions,’ ‘social inclusion,’ and ‘design for independence,’ reflecting the issues that have received scholarly attention in social sciences. Finally, on the digital technology front, ‘smart home’ emerged in 2006, followed by the terms ‘digital divide’ and ‘user interface’ in the same year. The emergence of ‘VR’ in 2014, ‘AR’ in 2016, and ‘wearable computing’ in 2017 also explain the digital technology focal points worth attention in DDE research.
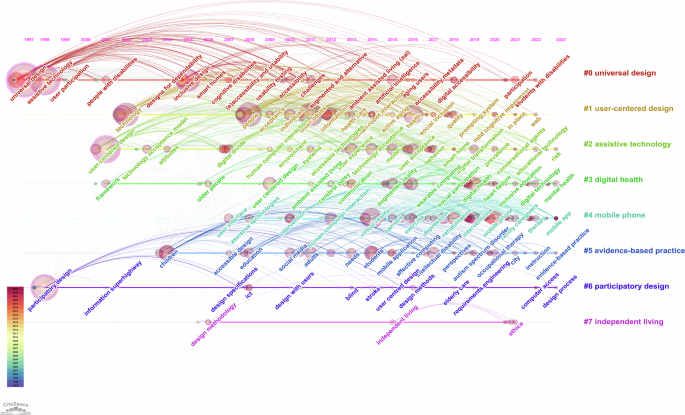
Timeline plot of 8 clusters of DDE keywords.
Dual-map overlays analysis of publications clusters
The double map overlay functionality of CiteSpace has been utilized to present a panoramic visualization of the knowledge base in DDE research (Fig. 10 ). This technique maps the citation dynamics between clusters of cited and cited publications, revealing the field’s interdisciplinary nature and scholarly communication. The left side of the figure depicts clusters of citing publications, showcasing newer disciplinary domains within DDE research. In contrast, the right side represents clusters of cited publications, reflecting the research foundations of DDE studies. Different colored dots within each cluster indicate the distribution of publications in that cluster. Notably, the arcs spanning the visualization illustrate the citation relationships between publications, with the thickness of the arcs corresponding to the citation volume. These citation trajectories from citing to cited clusters demonstrate the knowledge transfer and intellectual lineage of current DDE research within and across disciplinary boundaries. Notably, the Z-score algorithm converged on those arcs with stronger associations, yielding thicker arcs in green and blue. This indicates that the foundation of DDE research stems from two main disciplinary areas, namely ‘5.
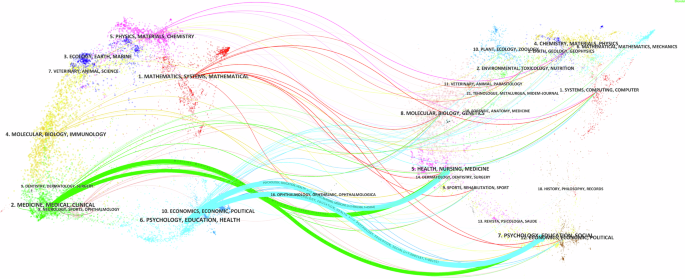
The left side represents citing publication clusters and the right side represents cited publication clusters.
Health, nursing, medicine’ and ‘7. psychology, education, and social on the right side of the figure. Publications from ‘2. MEDICINE, MEDICAL, CLINICAL’ and ‘10. ECONOMICS, ECONOMIC POLITICAL,’ and ‘6. On the left side, PSYCHOLOGY, EDUCATION, and health cite these two disciplinary areas extensively. In other words, the knowledge frontier of DDE research is concentrated in medicine and psychology, and their knowledge bases are also in the domains of health and psychology. However, there is a bidirectional cross-disciplinary citation relationship between the two areas—additionally, the red arcs from the ‘1. MATHEMATICS, SYSTEMS, MATHEMATICAL’ publications cluster showcase another facet of the knowledge frontier in DDE research, as they cite multiple clusters on the right side, forming a divergent structure, which confirms that some of the frontiers of MATHEMATICS in DDE research are based on a broader range of disciplines. The different network structures macroscopically reveal the overall developmental pattern of DDE research.
Hotspots and emerging trends
To answer RQ3, based on the research findings, the literature was re-engaged to reveal the research hotspots and emerging trends of DDE. These hotspots and trends are primarily concentrated in the following areas:
Embracing co-design and practical implementation in inclusive and universal design research
Research in inclusive and universal design increasingly emphasizes co-design with stakeholders, reflecting significant growth in publication (Table 4 on Co-design). In the digital context, transitioning from theory to practice in equity-centered design calls for enhanced adaptability and feasibility of traditional design theories. This shift requires a pragmatic and progressive approach, aligning with recent research (Zhang et al., 2023 ). Furthermore, the evidence-based practices in DDE (Cluster #6) are integral to this dimension, guiding the pragmatic application of design theories.
Focusing on digital mental health and urban-rural inequalities
In DDE, critical issues like the digital divide and mental health are central concerns. The focus on digital and mobile health, highlighted in Fig. 9 , shows a shift towards using technology to improve user engagement and address health challenges. As highlighted by Cosco (Cosco et al., 2021 ), mental health has emerged as a crucial focus in DDE, underscoring the need for designs that support psychological well-being. Additionally, ageism (Mannheim et al., 2023 ) and stereotypes (Nicosia et al., 2022 ) influence technology design in DDE, pointing to societal challenges that need addressing for more inclusive digital solutions. Patten’s (Patten et al., 2022 ) focus on smoking cessation in rural communities indicates a growing emphasis on reducing health disparities, ensuring that digital health advancements are inclusive and far-reaching. These trends in DDE highlight the importance of a holistic approach that considers technological, societal, and health-related factors.
Integration of empathetic, contextualized, and non-visual digital technologies
In the realm of DDE, the technology dimension showcases a range of emerging trends and research hotspots characterized by advancements in immersive technologies, assistive devices, and interactive systems. Technologies like VR (Bortkiewicz et al., 2023 ) and AR (Creed et al., 2023 ) are revolutionizing user experiences, offering enhanced empathy and engagement while raising new challenges. The growth in mobile phone usage (Cluster #4) and the development of 3D-printed individualized assistive devices (IADs) (Lamontagne et al., 2023 ) reflect an increasing emphasis on personalization and catering to diverse user needs. Tangible interfaces (Aguiar et al., 2023 ) and haptic recognition systems (Lu et al., 2023 ) make digital interactions more intuitive. The integration of cognitive assistive technology (Roberts et al., 2023 ) and brain-computer interfaces (BCI) (Padfield et al., 2023 ) is opening new avenues for user interaction, particularly for those with cognitive or physical limitations. The exploration of Social Assistive Robots (SAR) (Kaplan et al., 2024 ) and the application of IoT (Almukadi, 2023 ) illustrate a move towards socially aware and interconnected digital ecosystems, while voice recognition technologies (Berner & Alves, 2023 ) are enhancing accessibility. Edge computing (Walczak et al., 2023 ) represents a shift towards decentralized and user-oriented solutions.
For intergenerational relationships, students with disabilities and the visually impaired
The concurrent digitization trends and rapid global aging closely resemble the growth curve of DDE publications, as shown in Fig. 2 . The concept of active aging, championed by WHO (World Health Organization 2002 ), exerts a substantial impact. This effect is evident across multiple indicators, including a significant number of DDE papers published in the journal GERONTOLOGIST (109 articles), the prominent node of “elderly people” in keyword co-occurrence, and the notable mention of “elderly people” in keyword analysis (strength=3.62). Moreover, in 2011, China, the country with the largest elderly population globally, contributed 73 articles related to DDE (Table 2 ), further emphasizing the growing demand for future DDE research focusing on the elderly. Within DDE studies on the elderly, intergenerational relationships (Li & Cao, 2023 ) represent an emerging area of research. Additionally, two other emerging trends are centered on the educational and visually impaired populations. The term ‘students with disabilities’ in Fig. 9 illustrates this trend. This is reflected in the focus on inclusive digital education (Lazou & Tsinakos, 2023 ) and the digital health needs of the visually impaired (Yeong et al., 2021 ), highlighting the expanding scope of user-centric DDE research.
The intellectual structure of DDE
Previous studies have dissected DDE through various disciplinary lenses, often yielding isolated empirical findings. However, a comprehensive synthesis that contemplates the intricate interplay among DDE constructs has yet to be conspicuously absent. To fill this gap and answer RQ4, an intellectual structure that encapsulates the entirety of DDE was developed, amalgamating user demographics, design strategies, interdisciplinary approaches, and the overarching societal implications. This holistic structure, depicted in Fig. 11 , The DDE structure elucidates the multi-faceted approach required to achieve digital equity, integrating diverse user needs with tailored design strategies and bridging technological innovation with interdisciplinary methodologies. Its core function is to guide the creation of inclusive digital environments that are accessible, engaging, and responsive to the varied demands of a broad user spectrum.
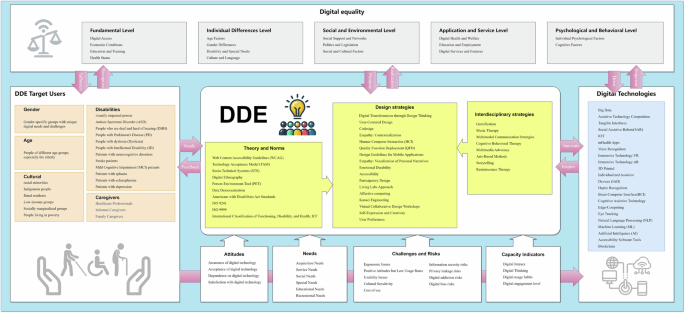
Design for Digital Equity (DDE) intellectual structure.
At the core of discussions surrounding digital equity lies the extensively examined and articulated issue of the digital divide, a well-documented challenge that scholars have explored (Gallegos-Rejas et al., 2023 ). This is illustrated in the concentric circles of the red core within the keyword contribution analysis, as depicted in Fig. 6 . It reflects the persistent digital access and literacy gaps that disproportionately affect marginalized groups. This divide extends beyond mere connectivity to encompass the nuances of social engagement (Almukadi, 2023 ), where the ability to participate meaningfully in digital spaces becomes a marker of societal inclusion. As noted by (Bochicchio et al., 2023 ; Jetha et al., 2023 ), employment is a domain where digital inequities manifest, creating barriers to employment inclusion. Similarly, feelings of loneliness, social isolation (Chung et al., 2023 ), and deficits in social skills (Estival et al., 2023 ) are exacerbated in the digital realm, where interactions often require different competencies. These social dimensions of DDE underscore the need for a more empathetic and user-informed approach to technology design, one that can cater to the nuanced needs of diverse populations, including medication reminders and telehealth solutions (Gallegos-Rejas et al., 2023 ) while minimizing cognitive load (Gomez-Hernandez et al., 2023 ) and advancing digital health equity (Ha et al., 2023 ).
The critical element of the DDE intellectual structure is the design strategy, as evidenced by the two categories #0 generic design and #6 participatory design, which contain the most prominent nodes in the keyword clustering in Part IV of this paper. Digital transformation through design thinking (Oliveira et al., 2024 ), user-centered design (Stawarz et al., 2023 ), and the co-design of 3D printed assistive technologies (Aflatoony et al., 2023 ; Benz et al., 2023 ; Ghorayeb et al., 2023 ) reflect the trend towards personalized and participatory design processes. Empathy emerges as a recurrent theme, both in contextualizing user experiences (Bortkiewicz et al., 2023 ) and in visualizing personal narratives (Gui et al., 2023 ), reinforcing the need for emotional durability (Huang et al., 2023 ) and accessible design (Jonsson et al., 2023 ). These approaches are not merely theoretical but are grounded in the pragmatics of participatory design (Kinnula et al., 2023 ), the living labs approach (Layton et al., 2023 ), and virtual collaborative design workshops (Peters et al., 2023 ), all of which facilitate the co-creation of solutions that resonate with the lived experiences of users.
One of the significant distinctions between DDE and traditional fairness-centered design lies in technical specifications. Supporting these strategies are fundamental theories and standards such as the Web Content Accessibility Guidelines (WCAG) (Jonsson et al., 2023 ), the Technology Acceptance Model (TAM) (Alvarez-Melgarejo et al., 2023 ), and socio-technical systems (STS) (Govers & van Amelsvoort, 2023 ), which provide the ethical and methodological framework for DDE initiatives. Additionally, digital ethnography (Joshi et al., 2023 ) and the Person-Environment-Tool (PET) framework (Jarl, Lundqvist 2020 ) offer valuable perspectives to analyze and design for the intricate interplay of human, technological, and environmental interactions.
Another noteworthy discovery highlighted by the previously mentioned findings is the rich interdisciplinary approach within the field of DDE. This interdisciplinary nature, exemplified by the integration of diverse knowledge domains, is evident in publications analysis of DDE (Table 4 ) and is visually demonstrated through the overlay of disciplinary citation networks (Fig. 10 ). Strategies such as gamification (Aguiar et al., 2023 ), music therapy (Chen & Norgaard, 2023 ), and multimodal communication strategies (Given et al., 2023 ) underline the synergistic potential of integrating diverse knowledge domains to foster more inclusive digital environments. Cognitive Behavioral Therapy (Kayser et al., 2023 ), multimedia advocacy (Watts et al., 2023 ), arts-based methods (Miller & Zelenko, 2022 ), storytelling (Ostrowski et al., 2021 ), and reminiscence therapy (Unbehaun et al., 2021 ) are not merely adjuncts but integral components that enhance the relevance and efficacy of DDE interventions.
Equally important, the relationship between the target users of DDE and digital technologies needs to be focused on as a design strategy. This includes attitudes, needs, challenges, risks, and capacity indicators. Positive outlooks envision digital transformation as a new norm post-pandemic for individuals with disabilities (Aydemir-Döke et al., 2023 ), while others display varied sentiments (Bally et al., 2023 ) or even hostile attitudes, as seen in the challenges of visually impaired with online shopping (Cohen et al., 2023 ). These attitudes interplay with ‘Needs’ that span essential areas, from service to recreational, highlighting the importance of ‘Capacity Indicators’ like digital literacy and digital thinking (Govers & van Amelsvoort, 2023 ) to bridge these gaps. The ‘Challenges and Risks’ associated with DDE, such as the adverse impacts of apps in medical contexts (Babbage et al., 2023 ) and ergonomic issues due to immersive technologies (Creed et al., 2023 ), present barriers that need to be mitigated to foster a conducive environment for digital engagement. Despite a generally positive attitude toward digital transformation, the low usage rates (Dale et al., 2023 ), usability concerns (Davoody et al., 2023 ), cultural differences in thinking, and the need for a humanizing digital transformation (Dragicevic et al., 2023b ) underscore the complexity of achieving digital equity. The widespread resistance and abandonment of rehabilitative technologies (Mitchell et al., 2023 ) further emphasize the need for DDE strategies that are culturally sensitive and user-friendly.
Going deeper, the arrows signify dynamic interrelationships among various components within the DDE intellectual structure. “Needs” drive the design and application of “Digital Technologies,” which in turn inspire “Innovative” solutions and approaches. Feedback from these innovations influences “Attitudes,” which, along with “Needs,” can pose “Challenges and Risks,” thereby shaping the “Capacity Indicators” that gauge proficiency in navigating the digital landscape. This cyclical interplay ensures that the DDE framework is not static but an evolving guide responsive to the changing landscape of digital equity.
future research direction
In the process of identifying research gaps and future directions, innovative research opportunities were determined from the results of temporal attributes in the visual, intellectual graph:
Emotional, cultural, and aesthetic factors in human-centered design: Universal Design (UD) and Design for All (DFA) will remain central themes in DDE. However, affective computing and user preferences must be explored (Alves et al., 2020 ). Beyond functional needs, experiential demands such as aesthetics, self-expression, and creativity, often overlooked in accessibility guidelines, are gaining recognition (Recupero et al., 2021 ). The concept of inclusive fashion (Oliveira & Okimoto, 2022 ) underscores the need to address multi-faceted user requirements, including fashion needs, cultural sensitivity, and diversity.
Digital technology adoption and improving digital literacy: The adoption of multimodal and multisensory interactions is gaining increased attention, with a growing focus on voice, tangible, and natural interaction technologies, alongside research into technology acceptance, aligning with the findings (Li et al., 2023 ). Exploring these interactive methods is crucial for enhancing user engagement and experience. However, there is a notable gap in research on the acceptance of many cutting-edge digital technologies. Additionally, investigating how design strategies can enhance digital literacy represents a valuable study area.
Expanding the Geographic and Cultural Scope: Literature indicates that the situation of DDE in developing countries (Abbas et al., 2014 ; Nahar et al., 2015 ) warrants in-depth exploration. Current literature and the distribution of research institutions show a significant gap in DDE research in these regions, especially in rural areas (as seen in Tables 2 and 3 and Figs. 3 and 4 ). Most research and literature is concentrated in developed countries, with insufficient focus on developing nations. Conversely, within developed countries, research on DDE concerning minority groups (Pham et al., 2022 ) and affluent Indigenous populations (Henson et al., 2023 ) is almost nonexistent. This situation reveals a critical research gap: even in economically advanced nations, the needs and preferences of marginalized groups are often overlooked. These groups may face unique challenges and needs, which should be explored or understood in mainstream research.
Multi-disciplinary Research in Digital Equity Design: While publication analysis (Table 4 ) and knowledge domain flow (Fig. 10 ) reveal the interdisciplinary nature of DDE, the current body of research predominantly focuses on computer science, medical and health sciences, sociology, and design. This review underscores the necessity of expanding research efforts across a broader spectrum of disciplines to address the diverse needs inherent in DDE adequately. For instance, the fusion of art, psychology, and computer technology could lead to research topics such as “Digital Equity Design Guidelines for Remote Art Therapy.” Similarly, the amalgamation of education, computer science, design, and management studies might explore subjects like “Integrating XR in Inclusive Educational Service Design: Technological Acceptance among Special Needs Students.” These potential research areas not only extend the scope of DDE but also emphasize the importance of a holistic and multi-faceted approach to developing inclusive and accessible digital solutions.
Practical implication
This study conducted an in-depth bibliometric and visualization analysis of the Digital Equity Design (DDE) field, revealing key findings on publication trends, significant contributors and collaboration patterns, key clusters, research hotspots, and intellectual structures. These insights directly affect policy-making, interdisciplinary collaboration, design optimization, and educational resource allocation. Analysis of publication trends provides policymakers with data to support digital inclusivity policies, particularly in education and health services, ensuring fair access to new technologies for all social groups, especially marginalized ones. The analysis of significant contributors and collaboration patterns highlights the role of interdisciplinary cooperation in developing innovative solutions, which is crucial for organizations and businesses designing products for specific groups, such as the disabled and elderly, in promoting active aging policies. Identifying key clusters and research hotspots guides the focus of future technological developments, enhancing the social adaptability and market competitiveness of designs. The construction of intellectual structures showcases the critical dimensions of user experience within DDE and the internal logic between various elements, providing a foundation for promoting deeper user involvement and more precise responses to needs in design research and practice, particularly in developing solutions and assessing their effectiveness to ensure that design outcomes truly reflect and meet end-user expectations and actual use scenarios.
Limitations
Nevertheless, this systematic review is subject to certain limitations. Firstly, the data sourced exclusively from the WOS is a constraint, as specific functionalities like dual-map overlays are uniquely tailored for WOS bibliometric data. Future studies could expand the scope by exploring DDE research in databases such as Scopus, Google Scholar, and grey literature. Additionally, while a comprehensive search string for DDE was employed, the results were influenced by the search timing and the subscription range of different research institutions to the database. Moreover, the possibility of relevant terms existing beyond the search string cannot be discounted. Secondly, despite adhering to the PRISMA guidelines for literature acquisition and screening, subjectivity may have influenced the authors during the inclusion and exclusion process, particularly while reviewing abstracts and full texts to select publications. Furthermore, the reliance solely on CiteSpace as the bibliometric tool introduces another limitation. The research findings are contingent on the features and algorithms of the current version of CiteSpace (6.2.r6 advanced). Future research could incorporate additional or newer versions of bibliometric tools to provide a more comprehensive analysis.
This systematic review aims to delineate the academic landscape of DDE by exploring its known and unknown aspects, including research progress, intellectual structure, research hotspots and trends, and future research recommendations. Before this review, these facets could have been clearer. To address these questions, a structured retrieval strategy set by PICo and a PRISMA process yielded 1705 publications, which were analyzed using CiteSpace for publication trends, geographic distribution of research collaborations, core publications, keyword co-occurrence, emergence, clustering, timelines, and dual-map overlays of publication disciplines. These visual presentations propose a DDE intellectual structure, although the literature data is focused on the WOS database. This framework could serve as a guide for future research to address these crucial issues. The DDE intellectual structure integrates research literature, particularly eight thematic clusters. It not only displays the overall intellectual structure of DDE on a macro level but also reveals the intrinsic logic between various elements. Most notably, as pointed out at the beginning of this review, digital equity, as a critical factor in achieving sustainable development goals, requires human-centered design thinking. An in-depth discussion of the research findings reveals that the development of DDE is characterized by a multi-dimensional approach, encompassing a wide range of societal, technological, and user-specific issues. Furthermore, emerging trends indicate that the future trajectory of DDE will be more diverse and inclusive, targeting a broad spectrum of user needs and societal challenges. Another significant aspect of this review is the proposition of four specific directions for future research, guiding researchers dedicated to related disciplines.
Data availability
The datasets generated or analyzed during the current study are available in the Dataverse repository: https://dataverse.harvard.edu/dataset.xhtml?persistentId=doi:10.7910/DVN/S5XXFB .
Abbas A, Hussain M, Iqbal M, Arshad S, Rasool S, Shafiq M, Ali W, Yaqub N (2014) Barriers and reforms for promoting ICTs in rural areas of Pakistan. In: Marcus A (ed), pp 391–399
Aflatoony L, Lee SJ, Sanford J (2023) Collective making: Co-designing 3D printed assistive technologies with occupational therapists, designers, and end-users. Assist Technol 35:153–162. https://doi.org/10.1080/10400435.2021.1983070
Article PubMed Google Scholar
Aguiar LR, Rodríguez FJ, Aguilar JR, Plascencia VN, Mendoza LM, Valdez JR, Pech JR, am Leon, Ortiz LE (2023) Implementing gamification for blind and autistic people with tangible interfaces, extended reality, and universal design for learning: two case studies. Appl. Sci.-Basel 13. https://doi.org/10.3390/app13053159
Ahmad I, Ahmed G, Shah SAA, Ahmed E (2020) A decade of big data literature: analysis of trends in light of bibliometrics. J Supercomput 76:3555–3571. https://doi.org/10.1007/s11227-018-2714-x
Article Google Scholar
Almukadi W (2023) Smart scarf: An IOT-based solution for emotion recognition. Eng Technol Appl Sci Res 13:10870–10874. https://doi.org/10.48084/etasr.5952
Alvarez-Melgarejo M, Pedraza-Avella AC, Torres-Barreto ML (2023) Acceptance assessment of the software MOTIVATIC WEB by university educators. Int J Learn Technol 18:344–363. https://doi.org/10.1504/IJLT.2023.134585
Alves T, Natálio J, Henriques-Calado J, Gama S (2020) Incorporating personality in user interface design: A review. Personality and Individual Differences 155. https://doi.org/10.1016/j.paid.2019.109709
Aydemir-Döke D, Owenz M, Spencer B (2023) Being a disabled woman in a global pandemic: A focus group study in the United States and policy recommendations. Disability & Society. https://doi.org/10.1080/09687599.2023.2225207
Babbage, Drown J, van Solkema M, Armstrong J, Levack W, Kayes N (2023) Inpatient trial of a tablet app for communicating brain injury rehabilitation goals. Disabil. Rehabil.-Assist. Technol. https://doi.org/10.1080/17483107.2023.2167009
Bally EL, Cheng DM, van Grieken A, Sanz MF, Zanutto O, Carroll A, Darley A, Roozenbeek B, Dippel DW, Raat H (2023) Patients’ Perspectives Regarding Digital Health Technology to Support Self-management and Improve Integrated Stroke Care: Qualitative Interview Study. J Med Internet Res 25. https://doi.org/10.2196/42556
Bazzano AN, Noel L-A, Patel T, Dominique CC, Haywood C, Moore S, Mantsios A, Davis PA (2023) Improving the engagement of underrepresented people in health research through equity-centered design thinking: qualitative study and process evaluation for the development of the grounding health research in design toolkit. JMIR Form Res 7:e43101. https://doi.org/10.2196/43101
Article PubMed PubMed Central Google Scholar
Bendixen K, Benktzon M (2015) Design for all in Scandinavia – A strong concept. Appl Erg 46:248–257. https://doi.org/10.1016/j.apergo.2013.03.004
Benz C, Scott-Jeffs W, Revitt J, Brabon C, Fermanis C, Hawkes M, Keane C, Dyke R, Cooper S, Locantro M, Welsh M, Norman R, Hendrie D, Robinson S (2023) Co-designing a telepractice journey map with disability customers and clinicians: Partnering with users to understand challenges from their perspective. Health Expect. https://doi.org/10.1111/hex.13919
Berner K, Alves (2023) A scoping review of the literature using speech recognition technologies by individuals with disabilities in multiple contexts. Disabil Rehabil -Assist Technol 18:1139–1145. https://doi.org/10.1080/17483107.2021.1986583
Bochicchio V, Lopez A, Hase A, Albrecht J, Costa B, Deville A, Hensbergen R, Sirfouq J, Mezzalira S (2023) The psychological empowerment of adaptive competencies in individuals with Intellectual Disability: Literature-based rationale and guidelines for best training practices. Life Span Disabil 26:129–157. https://doi.org/10.57643/lsadj.2023.26.1_06
Bortkiewicz A, Józwiak Z, Laska-Lesniewicz A (2023) Ageing and its consequences - the use of virtual reality (vr) as a tool to visualize the problems of elderly. Med Pr 74:159–170. https://doi.org/10.13075/mp.5893.01406
Broadus RN (1987) Toward a definition of “bibliometrics. Scientometrics 12:373–379. https://doi.org/10.1007/BF02016680
Chen C (2006) CiteSpace II: Detecting and visualizing emerging trends and transient patterns in scientific literature. J Am Soc Inf Sci 57:359–377. https://doi.org/10.1002/asi.20317
Chen C (2018) Eugene Garfield’s scholarly impact: a scientometric review. Scientometrics 114:489–516. https://doi.org/10.1007/s11192-017-2594-5
Chen C, Hu Z, Liu S, Tseng H (2012) Emerging trends in regenerative medicine: a scientometric analysis in CiteSpace. Expert Opin Biol Ther 12:593–608. https://doi.org/10.1517/14712598.2012.674507
Article CAS PubMed Google Scholar
Chen YA, Norgaard M (2023) Important findings of a technology-assisted in-home music-based intervention for individuals with stroke: a small feasibility study. Disabil. Rehabil.-Assist. Technol. https://doi.org/10.1080/17483107.2023.2274397
Chung JE, Gendron T, Winship J, Wood RE, Mansion N, Parsons P, Demiris G (2023) Smart Speaker and ICT Use in Relationship With Social Connectedness During the Pandemic: Loneliness and Social Isolation Found in Older Adults in Low-Income Housing. Gerontologist. https://doi.org/10.1093/geront/gnad145
Clarivate (2023) Web of Science Core Collection - Clarivate. https://clarivate.com/products/scientific-and-academic-research/research-discovery-and-workflow-solutions/webofscience-platform/web-of-science-core-collection/ . Accessed December 14, 2023
Cohen AH, Fresneda JE, Anderson RE (2023) How inaccessible retail websites affect blind and low vision consumers: their perceptions and responses. J Serv Theory Pract 33:329–351. https://doi.org/10.1108/JSTP-08-2021-0167
Cooper C, Booth A, Varley-Campbell J, Britten N, Garside R (2018) Defining the process to literature searching in systematic reviews: a literature review of guidance and supporting studies. BMC Med Res Methodol 18:85. https://doi.org/10.1186/s12874-018-0545-3
Cooper BA, Cohen U, Hasselkus BR (1991) Barrier-free design: a review and critique of the occupational therapy perspective. Am J Occup Ther 45:344–350. https://doi.org/10.5014/ajot.45.4.344
Cosco TD, Fortuna K, Wister A, Riadi I, Wagner K, Sixsmith A (2021) COVID-19, Social Isolation, and Mental Health Among Older Adults: A Digital Catch-22. J Med Internet Res 23. https://doi.org/10.2196/21864
Creed C, Al-Kalbani M, Theil A, Sarcar S, Williams I (2023) Inclusive AR/VR: accessibility barriers for immersive technologies. Univ Access Inf Soc. https://doi.org/10.1007/s10209-023-00969-0
Dale J, Nanton V, Day T, Apenteng P, Bernstein CJ, Smith GG, Strong P, Procter R (2023) Uptake and use of care companion, a web-based information resource for supporting informal carers of older people: mixed methods study. JMIR Aging 6. https://doi.org/10.2196/41185
Davoody N, Eghdam A, Koch S, Hägglund M (2023) Evaluation of an electronic care and rehabilitation planning tool with stroke survivors with Aphasia: Usability study. JMIR Human Factors 10. https://doi.org/10.2196/43861
Dragicevic N, Vladova G, Ullrich A (2023a) Design thinking capabilities in the digital world: A bibliometric analysis of emerging trends. Front Educ 7. https://doi.org/10.3389/feduc.2022.1012478
Dragicevic N, Hernaus T, Lee RW (2023b) Service innovation in Hong Kong organizations: Enablers and challenges to design thinking practices. Creat Innov Manag 32:198–214. https://doi.org/10.1111/caim.12555
Estival S, Demulier V, Renaud J, Martin JC (2023) Training work-related social skills in adults with Autism Spectrum Disorder using a tablet-based intervention. Human-Comput Interact. https://doi.org/10.1080/07370024.2023.2242344
Firestone AR, Cruz RA, Massey D (2023) Developing an equity-centered practice: teacher study groups in the preservice context. J Teach Educ 74:343–358. https://doi.org/10.1177/00224871231180536
Gallegos-Rejas VM, Thomas EE, Kelly JT, Smith AC (2023) A multi-stakeholder approach is needed to reduce the digital divide and encourage equitable access to telehealth. J Telemed Telecare 29:73–78. https://doi.org/10.1177/1357633X221107995
Garfield E (2009) From the science of science to Scientometrics visualizing the history of science with HistCite software. J Informetr 3:173–179. https://doi.org/10.1016/j.joi.2009.03.009
Ghorayeb A, Comber R, Gooberman-Hill R (2023) Development of a smart home interface with older adults: multi-method co-design study. JMIR Aging 6. https://doi.org/10.2196/44439
Given F, Allan M, Mccarthy S, Hemsley B (2023) Digital health autonomy for people with communication or swallowing disability and the sustainable development goal 10 of reducing inequalities and goal 3 of good health and well-being. Int J Speech-Lang Pathol 25:72–76. https://doi.org/10.1080/17549507.2022.2092212
Gomez-Hernandez M, Ferre X, Moral C, Villalba-Mora E (2023) Design guidelines of mobile apps for older adults: systematic review and thematic analysis. JMIR Mhealth and Uhealth 11. https://doi.org/10.2196/43186
Govers M, van Amelsvoort P (2023) A theoretical essay on socio-technical systems design thinking in the era of digital transformation. Gio-Gr -Interakt -Organ -Z Fuer Angew Org Psychol 54:27–40. https://doi.org/10.1007/s11612-023-00675-8
Grybauskas A, Stefanini A, Ghobakhloo M (2022) Social sustainability in the age of digitalization: A systematic literature Review on the social implications of industry 4.0. Technol Soc 70:101997. https://doi.org/10.1016/j.techsoc.2022.101997
Gui F, Yang JY, Wu QL, Liu Y, Zhou J, An N (2023) Enhancing caregiver empowerment through the story mosaic system: human-centered design approach for visualizing older adult life stories. JMIR Aging 6. https://doi.org/10.2196/50037
Ha S, Ho SH, Bae YH, Lee M, Kim JH, Lee J (2023) Digital health equity and tailored health care service for people with disability: user-centered design and usability study. J Med Internet Res 25. https://doi.org/10.2196/50029
Henson C, Chapman F, Cert G, Shepherd G, Carlson B, Rambaldini B, Gwynne K (2023) How older indigenous women living in high-income countries use digital health technology: systematic review. J Med Internet Res 25. https://doi.org/10.2196/41984
Huang XY, Kettley S, Lycouris S, Yao Y (2023) Autobiographical design for emotional durability through digital transformable fashion and textiles. Sustainability 15. https://doi.org/10.3390/su15054451
Ismail II, Saqr M (2022) A quantitative synthesis of eight decades of global multiple sclerosis research using bibliometrics. Front Neurol 13:845539. https://doi.org/10.3389/fneur.2022.845539
Jarl G, Lundqvist LO (2020) An alternative perspective on assistive technology: The person-environment-tool (PET) model. Assist Technol 32:47–53. https://doi.org/10.1080/10400435.2018.1467514
Jetha A, Bonaccio S, Shamaee A, Banks CG, Bültmann U, Smith PM, Tompa E, Tucker LB, Norman C, Gignac MA (2023) Divided in a digital economy: Understanding disability employment inequities stemming from the application of advanced workplace technologies. SSM-Qual Res Health 3. https://doi.org/10.1016/j.ssmqr.2023.100293
John Clarkson P, Coleman R (2015) History of inclusive design in the UK. Appl Erg 46(Pt B):235–247. https://doi.org/10.1016/j.apergo.2013.03.002
Jonsson M, Johansson S, Hussain D, Gulliksen J, Gustavsson C (2023) Development and evaluation of ehealth services regarding accessibility: scoping literature review. J Med Internet Res 25. https://doi.org/10.2196/45118
Joshi D, Panagiotou A, Bisht M, Udalagama U, Schindler A (2023) Digital Ethnography? Our experiences in the use of sensemaker for understanding gendered climate vulnerabilities amongst marginalized Agrarian communities. Sustainability 15. https://doi.org/10.3390/su15097196
Kaplan A, Barkan-Slater S, Zlotnik Y, Levy-Tzedek S (2024) Robotic technology for Parkinson’s disease: Needs, attitudes, and concerns of individuals with Parkinson’s disease and their family members. A focus group study. Int J Human-Comput Stud 181. https://doi.org/10.1016/j.ijhcs.2023.103148
Kastrin A, Hristovski D (2021) Scientometric analysis and knowledge mapping of literature-based discovery (1986–2020). Scientometrics 126:1415–1451. https://doi.org/10.1007/s11192-020-03811-z
Article CAS Google Scholar
Kayser J, Wang X, Wu ZK, Dimoji A, Xiang XL (2023) Layperson-facilitated internet-delivered cognitive behavioral therapy for homebound older adults with depression: protocol for a randomized controlled trial. JMIR Res Protocols 12. https://doi.org/10.2196/44210
King J, Gonzales AL (2023) The influence of digital divide frames on legislative passage and partisan sponsorship: A content analysis of digital equity legislation in the US from 1990 to 2020. Telecommun Policy 47:102573. https://doi.org/10.1016/j.telpol.2023.102573
Kinnula M, Iivari N, Kuure L, Molin-Juustila T (2023) Educational Participatory Design in the Crossroads of Histories and Practices - Aiming for digital transformation in language pedagogy. Comput Support Coop Work- J Collab Comput Work Pract. https://doi.org/10.1007/s10606-023-09473-8
Lamontagne ME, Pellichero A, Tostain V, Routhier F, Flamand V, Campeau-Lecours A, Gherardini F, Thébaud M, Coignard P, Allègre W (2023) The REHAB-LAB model for individualized assistive device co-creation and production. Assist Technol. https://doi.org/10.1080/10400435.2023.2229880
Lawson McLean A, Lawson McLean AC (2023) Exploring the digital divide: Implications for teleoncology implementation. Patient Educ Couns 115:107939. https://doi.org/10.1016/j.pec.2023.107939
Layton N, Harper K, Martinez K, Berrick N, Naseri C (2023) Co-creating an assistive technology peer-support community: learnings from AT Chat. Disabil Rehabil -Assist Technol 18:603–609. https://doi.org/10.1080/17483107.2021.1897694
Lazou C, Tsinakos A (2023) Critical Immersive-triggered literacy as a key component for inclusive digital education. Educ Sci 13. https://doi.org/10.3390/educsci13070696
Li G, Li D, Tang T (2023) Bibliometric review of design for digital inclusion. Sustainability 15. https://doi.org/10.3390/su151410962
Li C, Cao M (2023) Designing for intergenerational communication among older adults: A systematic inquiry in old residential communities of China’s Yangtze River Delta. Systems 11. https://doi.org/10.3390/systems11110528
Liberati A, Altman DG, Tetzlaff J, Mulrow C, Gøtzsche PC, Ioannidis JPA, Clarke M, Devereaux PJ, Kleijnen J, Moher D (2009) The PRISMA statement for reporting systematic reviews and meta-analyses of studies that evaluate health care interventions: explanation and elaboration. PLoS Med 6:e1000100. https://doi.org/10.1371/journal.pmed.1000100
Lu JY, Liu Y, Lv TX, Meng L (2023) An emotional-aware mobile terminal accessibility-assisted recommendation system for the elderly based on haptic recognition. International J Hum–Comput Interact. https://doi.org/10.1080/10447318.2023.2266793
Mace R (1985) Universal design: barrier-free environments for everyone. Design West 33:147–152
Google Scholar
Mannheim I, Wouters EJ, Köttl H, van Boekel LC, Brankaert R, van Zaalen Y (2023) Ageism in the discourse and practice of designing digital technology for older persons: a scoping review. Gerontologist 63:1188–1200. https://doi.org/10.1093/geront/gnac144
Miller E, Zelenko O (2022) The Caregiving Journey: Arts-based methods as tools for participatory co-design of health technologies. Social Sci-Basel 11. https://doi.org/10.3390/socsci11090396
Mitchell J, Shirota C, Clanchy K (2023) Factors that influence the adoption of rehabilitation technologies: a multi-disciplinary qualitative exploration. J NeuroEng Rehabil 20. https://doi.org/10.1186/s12984-023-01194-9
Moher D, Liberati A, Tetzlaff J, Altman DG (2010) Preferred reporting items for systematic reviews and meta-analyses: the PRISMA statement. Int J Surg 8:336–341. https://doi.org/10.1016/j.ijsu.2010.02.007
Nahar L, Jaafar A, Ahamed E, Kaish A (2015) Design of a Braille learning application for visually impaired students in Bangladesh. Assist Technol 27:172–182. https://doi.org/10.1080/10400435.2015.1011758
Nicosia J, Aschenbrenner AJ, Adams SL, Tahan M, Stout SH, Wilks H, Balls-Berry JE, Morris JC, Hassenstab J (2022) Bridging the technological divide: stigmas and challenges with technology in digital brain health studies of older adults. Front Digit Health 4. https://doi.org/10.3389/fdgth.2022.880055
Nishikawa-Pacher A (2022) Research questions with PICO: A universal mnemonic. Publications 10:21. https://doi.org/10.3390/publications10030021
Oliveira M, Zancul E, Salerno MS (2024) Capability building for digital transformation through design thinking. Technol Forecast Soc Change 198. https://doi.org/10.1016/j.techfore.2023.122947
de Oliveira RD, Okimoto M (2022) Fashion-related assistive technologies for visually impaired people: a systematic review. Dobras:183–205
Oliveri ME, Nastal J, Slomp D (2020) Reflections on Equity‐Centered Design. ETS Research Report Series 2020:1–11. https://doi.org/10.1002/ets2.12307
Ostrowski AK, Harrington CN, Breazeal C, Park HW (2021). Personal narratives in technology design: the value of sharing older adults’ stories in the design of social robots. Front Robot AI 8. https://doi.org/10.3389/frobt.2021.716581
Padfield N, Anastasi AA, Camilleri T, Fabri S, Bugeja M, Camilleri K (2023). BCI-controlled wheelchairs: end-users’ perceptions, needs, and expectations, an interview-based study. Disabil. Rehabil.-Assist. Technol. https://doi.org/10.1080/17483107.2023.2211602
Park K, So H-J, Cha H (2019) Digital equity and accessible MOOCs: Accessibility evaluations of mobile MOOCs for learners with visual impairments. AJET 35:48–63. https://doi.org/10.14742/ajet.5521
Patten C, Brockman T, Kelpin S, Sinicrope P, Boehmer K, St Sauver J, Lampman M, Sharma P, Reinicke N, Huang M, McCoy R, Allen S, Pritchett J, Esterov D, Kamath C, Decker P, Petersen C, Cheville A (2022) Interventions for Increasing Digital Equity and Access (IDEA) among rural patients who smoke: Study protocol for a pragmatic randomized pilot trial. Contemp Clin Trials 119. https://doi.org/10.1016/j.cct.2022.106838
Persson H, Åhman H, Yngling AA, Gulliksen J (2015) Universal design, inclusive design, accessible design, design for all: different concepts—one goal? On the concept of accessibility—historical, methodological and philosophical aspects. Univ Access Inf Soc 14:505–526. https://doi.org/10.1007/s10209-014-0358-z
Peters D, Sadek M, Ahmadpour N (2023) Collaborative workshops at scale: a method for non-facilitated virtual collaborative design workshops. Int J Hum–Comput Interact. https://doi.org/10.1080/10447318.2023.2247589
Pham Q, El-Dassouki N, Lohani R, Jebanesan A, Young K (2022) The future of virtual care for older ethnic adults beyond the COVID-19 pandemic. J Med Internet Res 24. https://doi.org/10.2196/29876
Ping Q, He J, Chen C (2017) How many ways to use CiteSpace? A study of user interactive events over 14 months. Assoc Info Sci Tech 68:1234–1256. https://doi.org/10.1002/asi.23770
Price DDS (1963) Science since Babylon. Philos Sci 30:93–94
PRISMA (2023) Transparent reporting of systematic reviews and meta-analyses. http://www.prisma-statement.org/ . Accessed December 14, 2023
Qin Y, Wang X, Xu Z, Škare M (2021) The impact of poverty cycles on economic research: evidence from econometric analysis. Econ Res -Ekonomska Istraživanja 34:152–171. https://doi.org/10.1080/1331677X.2020.1780144
Recupero A, Marti P, Guercio S (2021) Enabling inner creativity to surface: the design of an inclusive handweaving loom to promote self-reliance, autonomy, and well-being. Behav Inf Technol 40:497–505. https://doi.org/10.1080/0144929X.2021.1909654
Roberts E, Fan GL, Chen XW (2023) In-lab development of a mobile interface for cognitive assistive technology to support instrumental activities of daily living in dementia homecare. J Aging Environ 37:127–141. https://doi.org/10.1080/26892618.2021.2001710
Rodriguez NM, Burleson G, Linnes JC, Sienko KH (2023) Thinking beyond the device: an overview of human- and equity-centered approaches for health technology design. Annu Rev Biomed Eng 25:257–280. https://doi.org/10.1146/annurev-bioeng-081922-024834
Article CAS PubMed PubMed Central Google Scholar
Sayers A (2008) Tips and tricks in performing a systematic review–Chapter 4. Br J Gen Pr 58:136. https://doi.org/10.3399/bjgp08X277168
Singh S (2017) Bridging the gender digital divide in developing countries. J Child Media 11:245–247. https://doi.org/10.1080/17482798.2017.1305604
Stanford d.school (2016) Equity-Centered Design Framework. https://dschool.stanford.edu/resources/equity-centered-design-framework . Accessed December 14, 2023
Stawarz K, Liang IJ, Alexander L, Carlin A, Wijekoon A, Western MJ (2023) Exploring the potential of technology to promote exercise snacking for older adults who are prefrail in the home setting: user-centered design study. JMIR Aging 6. https://doi.org/10.2196/41810
Stern C, Jordan Z, McArthur A (2014) Developing the review question and inclusion criteria. Am J Nurs 114:53–56. https://doi.org/10.1097/01.NAJ.0000445689.67800.86
Unbehaun D, Taugerbeck S, Aal K, Vaziri DD, Lehmann J, Tolmie P, Wieching R, Wulf V (2021) Notes of memories: Fostering social interaction, activity and reminiscence through an interactive music exergame developed for people with dementia and their caregivers. Hum-Comput Interact 36:439–472. https://doi.org/10.1080/07370024.2020.1746910
United Nations (2021) International Day of Older Persons: Digital equity for all ages. ITU/UN tech agency
UNSD UNS (2023) — SDG Indicators. https://unstats.un.org/sdgs/report/2022/ . Accessed December 13, 2023
Van Eck NJ, Waltman L (2010) Software survey: VOSviewer, a computer program for bibliometric mapping. Scientometrics 84:523–538. https://doi.org/10.1007/s11192-009-0146-3
van Leeuwen T (2006) The application of bibliometric analyses in the evaluation of social science research. Who benefits from it, and why it is still feasible. Scientometrics 66:133–154. https://doi.org/10.1007/s11192-006-0010-7
Walczak R, Koszewski K, Olszewski R, Ejsmont K, Kálmán A (2023) Acceptance of IoT Edge-computing-based sensors in smart cities for universal design purposes. Energies 16. https://doi.org/10.3390/en16031024
Wang Z, Zhou Z, Xu W, Yang D, Xu Y, Yang L, Ren J, Li Y, Huang Y (2021) Research status and development trends in the field of marine environment corrosion: a new perspective. Environ Sci Pollut Res Int 28:54403–54428. https://doi.org/10.1007/s11356-021-15974-0
Wang J, Li X, Wang P, Liu Q, Deng Z, Wang J (2022) Research trend of the unified theory of acceptance and use of technology theory: a bibliometric analysis. Sustainability 14:10. https://doi.org/10.3390/su14010010
Watts P, Kwiatkowska G, Minnion A (2023) Using multimedia technology to enhance self-advocacy of people with intellectual disabilities: Introducing a theoretical framework for ‘Multimedia Advocacy. J Appl Res Intellect Disabil 36:739–749. https://doi.org/10.1111/jar.13107
Willems J, Farley H, Campbell C (2019) The increasing significance of digital equity in higher education. AJET 35:1–8. https://doi.org/10.14742/ajet.5996
World Health Organization (2002) Active aging: A policy framework. WHO, Geneva, Switzerland
Xiao Y, Wu H, Wang G, Mei H (2021) Mapping the Worldwide Trends on Energy Poverty Research: A Bibliometric Analysis (1999–2019). Int J Environ Res Public Health 18. https://doi.org/10.3390/ijerph18041764
Yeong JL, Thomas P, Buller J, Moosajee M (2021) A newly developed web-based resource on genetic eye disorders for users with visual impairment (Gene Vis): Usability Study. J Med Internet Res 23. https://doi.org/10.2196/19151
Yuen AHK, Park JH, Chen L, Cheng M (2017) Digital equity in cultural context: exploring the influence of Confucian heritage culture on Hong Kong families. Educ Tech Res Dev 65:481–501. https://doi.org/10.1007/s11423-017-9515-4
Zhang BY, Ma MY, Wang ZS (2023) Promoting active aging through assistive product design innovation: a preference-based integrated design framework. Front Public Health 11. https://doi.org/10.3389/fpubh.2023.1203830
Download references
Acknowledgements
This research was funded by the Humanities and Social Sciences Youth Foundation, Ministry of Education of the People’s Republic of China (21YJC760101).
Author information
Authors and affiliations.
Xiamen University of Technology, Xiamen, China
Baoyi Zhang
You can also search for this author in PubMed Google Scholar
Contributions
The author was responsible for all aspects of the work, including the conception, research, analysis, manuscript drafting, critical revision, and final approval of the version to be published. The author ensures the accuracy and integrity of the entire study.
Corresponding author
Correspondence to Baoyi Zhang .
Ethics declarations
Competing interests.
The author declares no competing interests.
Ethical approval
Ethical approval was not required as the study did not involve human participants.
Informed consent
Informed consent was not required as this study did not involve human participants.
Additional information
Publisher’s note Springer Nature remains neutral with regard to jurisdictional claims in published maps and institutional affiliations.
Rights and permissions
Open Access This article is licensed under a Creative Commons Attribution-NonCommercial-NoDerivatives 4.0 International License, which permits any non-commercial use, sharing, distribution and reproduction in any medium or format, as long as you give appropriate credit to the original author(s) and the source, provide a link to the Creative Commons licence, and indicate if you modified the licensed material. You do not have permission under this licence to share adapted material derived from this article or parts of it. The images or other third party material in this article are included in the article’s Creative Commons licence, unless indicated otherwise in a credit line to the material. If material is not included in the article’s Creative Commons licence and your intended use is not permitted by statutory regulation or exceeds the permitted use, you will need to obtain permission directly from the copyright holder. To view a copy of this licence, visit http://creativecommons.org/licenses/by-nc-nd/4.0/ .
Reprints and permissions
About this article
Cite this article.
Zhang, B. Research progress and intellectual structure of design for digital equity (DDE): A bibliometric analysis based on citespace. Humanit Soc Sci Commun 11 , 1019 (2024). https://doi.org/10.1057/s41599-024-03552-x
Download citation
Received : 27 December 2023
Accepted : 01 August 2024
Published : 08 August 2024
DOI : https://doi.org/10.1057/s41599-024-03552-x
Share this article
Anyone you share the following link with will be able to read this content:
Sorry, a shareable link is not currently available for this article.
Provided by the Springer Nature SharedIt content-sharing initiative
Quick links
- Explore articles by subject
- Guide to authors
- Editorial policies


Title Page Setup
A title page is required for all APA Style papers. There are both student and professional versions of the title page. Students should use the student version of the title page unless their instructor or institution has requested they use the professional version. APA provides a student title page guide (PDF, 199KB) to assist students in creating their title pages.
Student title page
The student title page includes the paper title, author names (the byline), author affiliation, course number and name for which the paper is being submitted, instructor name, assignment due date, and page number, as shown in this example.
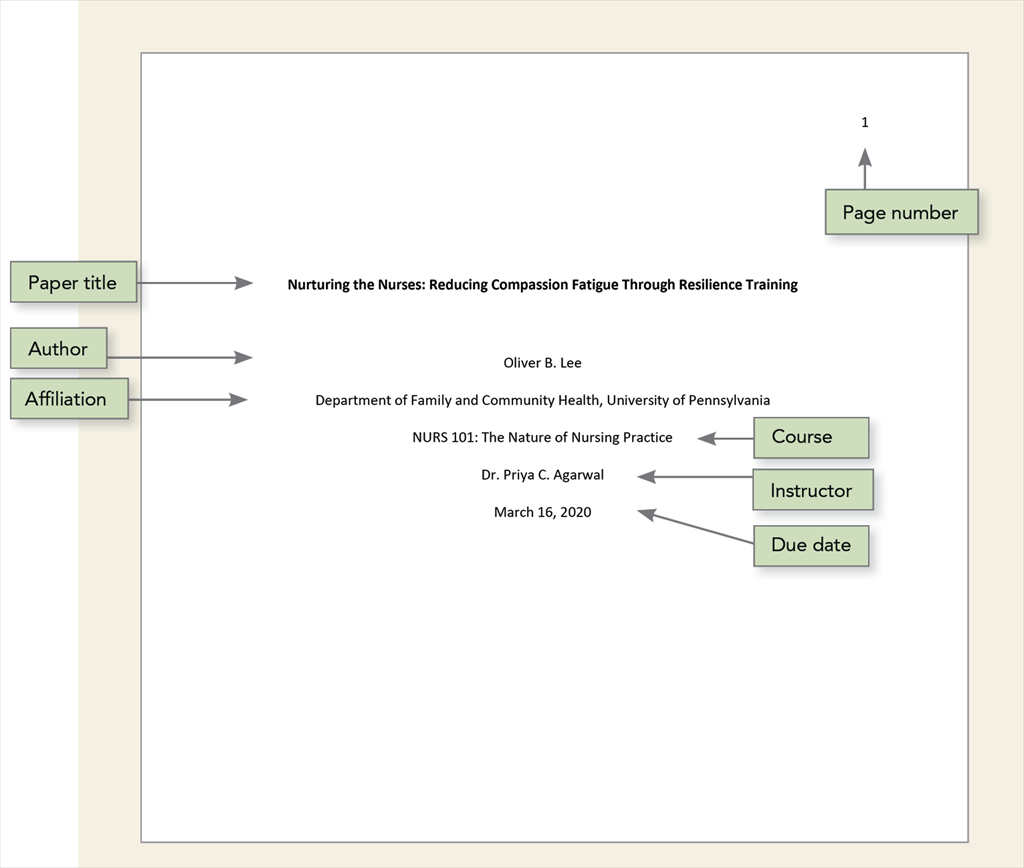
Title page setup is covered in the seventh edition APA Style manuals in the Publication Manual Section 2.3 and the Concise Guide Section 1.6

Related handouts
- Student Title Page Guide (PDF, 263KB)
- Student Paper Setup Guide (PDF, 3MB)
Student papers do not include a running head unless requested by the instructor or institution.
Follow the guidelines described next to format each element of the student title page.
|
|
|
---|---|---|
Paper title | Place the title three to four lines down from the top of the title page. Center it and type it in bold font. Capitalize of the title. Place the main title and any subtitle on separate double-spaced lines if desired. There is no maximum length for titles; however, keep titles focused and include key terms. |
|
Author names | Place one double-spaced blank line between the paper title and the author names. Center author names on their own line. If there are two authors, use the word “and” between authors; if there are three or more authors, place a comma between author names and use the word “and” before the final author name. | Cecily J. Sinclair and Adam Gonzaga |
Author affiliation | For a student paper, the affiliation is the institution where the student attends school. Include both the name of any department and the name of the college, university, or other institution, separated by a comma. Center the affiliation on the next double-spaced line after the author name(s). | Department of Psychology, University of Georgia |
Course number and name | Provide the course number as shown on instructional materials, followed by a colon and the course name. Center the course number and name on the next double-spaced line after the author affiliation. | PSY 201: Introduction to Psychology |
Instructor name | Provide the name of the instructor for the course using the format shown on instructional materials. Center the instructor name on the next double-spaced line after the course number and name. | Dr. Rowan J. Estes |
Assignment due date | Provide the due date for the assignment. Center the due date on the next double-spaced line after the instructor name. Use the date format commonly used in your country. | October 18, 2020 |
| Use the page number 1 on the title page. Use the automatic page-numbering function of your word processing program to insert page numbers in the top right corner of the page header. | 1 |
Professional title page
The professional title page includes the paper title, author names (the byline), author affiliation(s), author note, running head, and page number, as shown in the following example.
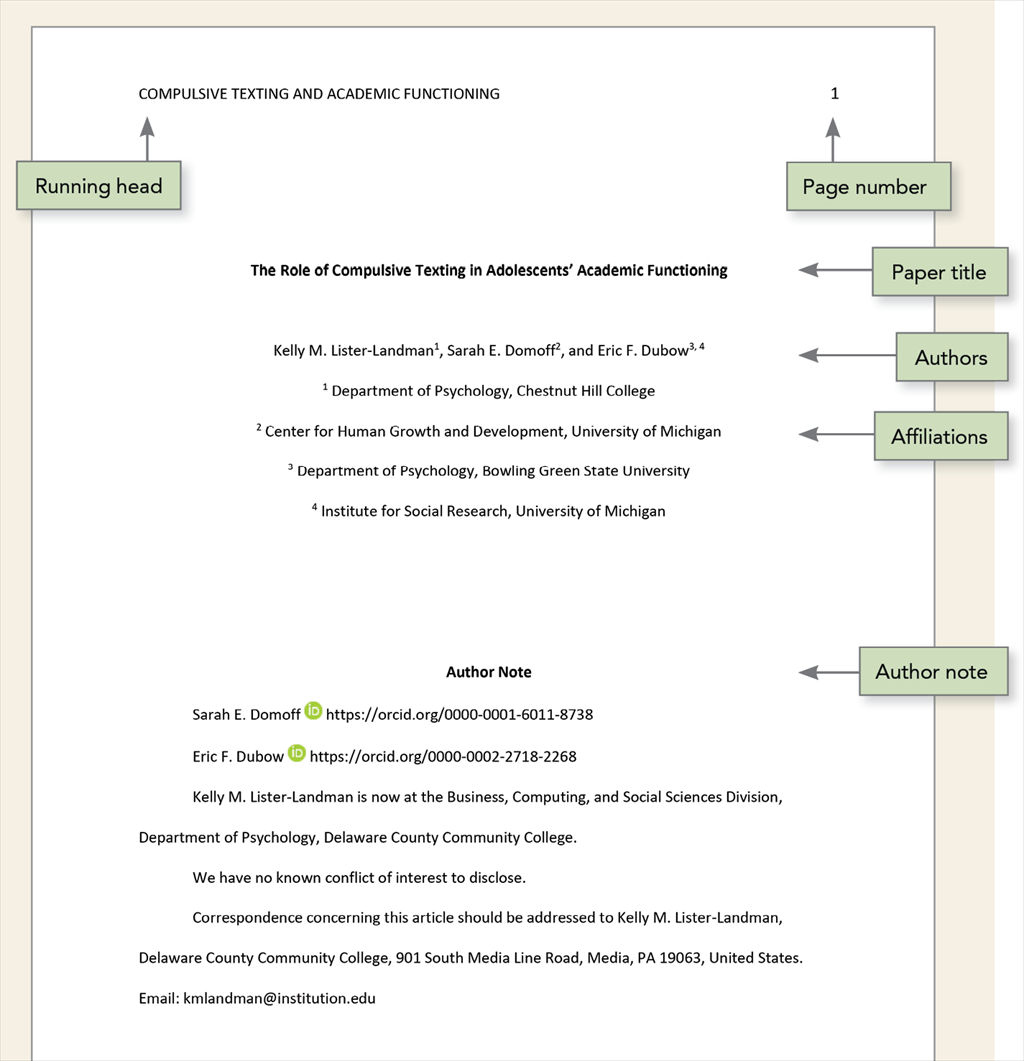
Follow the guidelines described next to format each element of the professional title page.
|
|
|
---|---|---|
Paper title | Place the title three to four lines down from the top of the title page. Center it and type it in bold font. Capitalize of the title. Place the main title and any subtitle on separate double-spaced lines if desired. There is no maximum length for titles; however, keep titles focused and include key terms. |
|
Author names
| Place one double-spaced blank line between the paper title and the author names. Center author names on their own line. If there are two authors, use the word “and” between authors; if there are three or more authors, place a comma between author names and use the word “and” before the final author name. | Francesca Humboldt |
When different authors have different affiliations, use superscript numerals after author names to connect the names to the appropriate affiliation(s). If all authors have the same affiliation, superscript numerals are not used (see Section 2.3 of the for more on how to set up bylines and affiliations). | Tracy Reuter , Arielle Borovsky , and Casey Lew-Williams | |
Author affiliation
| For a professional paper, the affiliation is the institution at which the research was conducted. Include both the name of any department and the name of the college, university, or other institution, separated by a comma. Center the affiliation on the next double-spaced line after the author names; when there are multiple affiliations, center each affiliation on its own line.
| Department of Nursing, Morrigan University |
When different authors have different affiliations, use superscript numerals before affiliations to connect the affiliations to the appropriate author(s). Do not use superscript numerals if all authors share the same affiliations (see Section 2.3 of the for more). | Department of Psychology, Princeton University | |
Author note | Place the author note in the bottom half of the title page. Center and bold the label “Author Note.” Align the paragraphs of the author note to the left. For further information on the contents of the author note, see Section 2.7 of the . | n/a |
| The running head appears in all-capital letters in the page header of all pages, including the title page. Align the running head to the left margin. Do not use the label “Running head:” before the running head. | Prediction errors support children’s word learning |
| Use the page number 1 on the title page. Use the automatic page-numbering function of your word processing program to insert page numbers in the top right corner of the page header. | 1 |
Have a language expert improve your writing
Run a free plagiarism check in 10 minutes, generate accurate citations for free.
- Knowledge Base
Methodology
- What Is Quantitative Research? | Definition, Uses & Methods
What Is Quantitative Research? | Definition, Uses & Methods
Published on June 12, 2020 by Pritha Bhandari . Revised on June 22, 2023.
Quantitative research is the process of collecting and analyzing numerical data. It can be used to find patterns and averages, make predictions, test causal relationships, and generalize results to wider populations.
Quantitative research is the opposite of qualitative research , which involves collecting and analyzing non-numerical data (e.g., text, video, or audio).
Quantitative research is widely used in the natural and social sciences: biology, chemistry, psychology, economics, sociology, marketing, etc.
- What is the demographic makeup of Singapore in 2020?
- How has the average temperature changed globally over the last century?
- Does environmental pollution affect the prevalence of honey bees?
- Does working from home increase productivity for people with long commutes?
Table of contents
Quantitative research methods, quantitative data analysis, advantages of quantitative research, disadvantages of quantitative research, other interesting articles, frequently asked questions about quantitative research.
You can use quantitative research methods for descriptive, correlational or experimental research.
- In descriptive research , you simply seek an overall summary of your study variables.
- In correlational research , you investigate relationships between your study variables.
- In experimental research , you systematically examine whether there is a cause-and-effect relationship between variables.
Correlational and experimental research can both be used to formally test hypotheses , or predictions, using statistics. The results may be generalized to broader populations based on the sampling method used.
To collect quantitative data, you will often need to use operational definitions that translate abstract concepts (e.g., mood) into observable and quantifiable measures (e.g., self-ratings of feelings and energy levels).
Research method | How to use | Example |
---|---|---|
Control or manipulate an to measure its effect on a dependent variable. | To test whether an intervention can reduce procrastination in college students, you give equal-sized groups either a procrastination intervention or a comparable task. You compare self-ratings of procrastination behaviors between the groups after the intervention. | |
Ask questions of a group of people in-person, over-the-phone or online. | You distribute with rating scales to first-year international college students to investigate their experiences of culture shock. | |
(Systematic) observation | Identify a behavior or occurrence of interest and monitor it in its natural setting. | To study college classroom participation, you sit in on classes to observe them, counting and recording the prevalence of active and passive behaviors by students from different backgrounds. |
Secondary research | Collect data that has been gathered for other purposes e.g., national surveys or historical records. | To assess whether attitudes towards climate change have changed since the 1980s, you collect relevant questionnaire data from widely available . |
Note that quantitative research is at risk for certain research biases , including information bias , omitted variable bias , sampling bias , or selection bias . Be sure that you’re aware of potential biases as you collect and analyze your data to prevent them from impacting your work too much.
Prevent plagiarism. Run a free check.
Once data is collected, you may need to process it before it can be analyzed. For example, survey and test data may need to be transformed from words to numbers. Then, you can use statistical analysis to answer your research questions .
Descriptive statistics will give you a summary of your data and include measures of averages and variability. You can also use graphs, scatter plots and frequency tables to visualize your data and check for any trends or outliers.
Using inferential statistics , you can make predictions or generalizations based on your data. You can test your hypothesis or use your sample data to estimate the population parameter .
First, you use descriptive statistics to get a summary of the data. You find the mean (average) and the mode (most frequent rating) of procrastination of the two groups, and plot the data to see if there are any outliers.
You can also assess the reliability and validity of your data collection methods to indicate how consistently and accurately your methods actually measured what you wanted them to.
Quantitative research is often used to standardize data collection and generalize findings . Strengths of this approach include:
- Replication
Repeating the study is possible because of standardized data collection protocols and tangible definitions of abstract concepts.
- Direct comparisons of results
The study can be reproduced in other cultural settings, times or with different groups of participants. Results can be compared statistically.
- Large samples
Data from large samples can be processed and analyzed using reliable and consistent procedures through quantitative data analysis.
- Hypothesis testing
Using formalized and established hypothesis testing procedures means that you have to carefully consider and report your research variables, predictions, data collection and testing methods before coming to a conclusion.
Despite the benefits of quantitative research, it is sometimes inadequate in explaining complex research topics. Its limitations include:
- Superficiality
Using precise and restrictive operational definitions may inadequately represent complex concepts. For example, the concept of mood may be represented with just a number in quantitative research, but explained with elaboration in qualitative research.
- Narrow focus
Predetermined variables and measurement procedures can mean that you ignore other relevant observations.
- Structural bias
Despite standardized procedures, structural biases can still affect quantitative research. Missing data , imprecise measurements or inappropriate sampling methods are biases that can lead to the wrong conclusions.
- Lack of context
Quantitative research often uses unnatural settings like laboratories or fails to consider historical and cultural contexts that may affect data collection and results.
Here's why students love Scribbr's proofreading services
Discover proofreading & editing
If you want to know more about statistics , methodology , or research bias , make sure to check out some of our other articles with explanations and examples.
- Chi square goodness of fit test
- Degrees of freedom
- Null hypothesis
- Discourse analysis
- Control groups
- Mixed methods research
- Non-probability sampling
- Inclusion and exclusion criteria
Research bias
- Rosenthal effect
- Implicit bias
- Cognitive bias
- Selection bias
- Negativity bias
- Status quo bias
Quantitative research deals with numbers and statistics, while qualitative research deals with words and meanings.
Quantitative methods allow you to systematically measure variables and test hypotheses . Qualitative methods allow you to explore concepts and experiences in more detail.
In mixed methods research , you use both qualitative and quantitative data collection and analysis methods to answer your research question .
Data collection is the systematic process by which observations or measurements are gathered in research. It is used in many different contexts by academics, governments, businesses, and other organizations.
Operationalization means turning abstract conceptual ideas into measurable observations.
For example, the concept of social anxiety isn’t directly observable, but it can be operationally defined in terms of self-rating scores, behavioral avoidance of crowded places, or physical anxiety symptoms in social situations.
Before collecting data , it’s important to consider how you will operationalize the variables that you want to measure.
Reliability and validity are both about how well a method measures something:
- Reliability refers to the consistency of a measure (whether the results can be reproduced under the same conditions).
- Validity refers to the accuracy of a measure (whether the results really do represent what they are supposed to measure).
If you are doing experimental research, you also have to consider the internal and external validity of your experiment.
Hypothesis testing is a formal procedure for investigating our ideas about the world using statistics. It is used by scientists to test specific predictions, called hypotheses , by calculating how likely it is that a pattern or relationship between variables could have arisen by chance.
Cite this Scribbr article
If you want to cite this source, you can copy and paste the citation or click the “Cite this Scribbr article” button to automatically add the citation to our free Citation Generator.
Bhandari, P. (2023, June 22). What Is Quantitative Research? | Definition, Uses & Methods. Scribbr. Retrieved August 19, 2024, from https://www.scribbr.com/methodology/quantitative-research/
Is this article helpful?
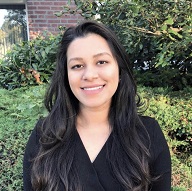
Pritha Bhandari
Other students also liked, descriptive statistics | definitions, types, examples, inferential statistics | an easy introduction & examples, what is your plagiarism score.
- Open access
- Published: 16 August 2024
Going virtual: mixed methods evaluation of online versus in-person learning in the NIH mixed methods research training program retreat
- Joseph J. Gallo 1 ,
- Sarah M. Murray 1 ,
- John W. Creswell 2 ,
- Charles Deutsch 3 &
- Timothy C. Guetterman 2
BMC Medical Education volume 24 , Article number: 882 ( 2024 ) Cite this article
63 Accesses
Metrics details
Despite the central role of mixed methods in health research, studies evaluating online methods training in the health sciences are nonexistent. The focused goal was to evaluate online training by comparing the self-rated skills of scholars who experienced an in-person retreat to scholars in an online retreat in specific domains of mixed methods research for the health sciences from 2015–2023.
The authors administered a scholar Mixed Methods Skills Self-Assessment instrument based on an educational competency scale that included domains on: “research questions,” “design/approach,” “sampling,” “analysis,” and “dissemination” to participants of the Mixed Methods Research Training Program for the Health Sciences (MMRTP). Self-ratings on confidence on domains were compared before and after retreat participation within cohorts who attended in person ( n = 73) or online ( n = 57) as well as comparing across in-person to online cohorts. Responses to open-ended questions about experiences with the retreat were analyzed.
Scholars in an interactive program to improve mixed methods skills reported significantly increased confidence in ability to define or explain concepts and in ability to apply the concepts to practical problems, whether the program was attended in-person or synchronously online. Scholars in the online retreat had self-rated skill improvements as good or better than scholars who participated in person. With the possible exception of networking, scholars found the online format was associated with advantages such as accessibility and reduced burden of travel and finding childcare. No differences in difficulty of learning concepts was described.
Conclusions
Keeping in mind that the retreat is only one component of the MMRTP, this study provides evidence that mixed methods training online was associated with the same increases in self-rated skills as persons attending online and can be a key component to increasing the capacity for mixed methods research in the health sciences.
Peer Review reports
Introduction
The coronavirus pandemic accelerated interest in distance or remote learning. While the acute nature of the pandemic has abated, changes in the way people work have largely remained, with hybrid conferences and trainings more commonly implemented now than during the pre-pandemic period. Studies of health-related online teaching have focused on medical students [ 1 , 2 , 3 ], health professionals [ 4 , 5 ], and medical conferences [ 6 , 7 , 8 ] and have touted the advantages of virtual training and conferences in health education, but few studies have assessed relative growth in skills and competencies in health research methods for synchronous online vs. in-person training.
The National Institutes of Health (NIH)-funded Mixed Methods Research Training Program (MMRTP) for the Health Sciences provided training to faculty-level investigators across health disciplines from 2015–2023. The NIH is a major funder of health-related research in the United States. Its institutes span diseases and conditions (e.g., mental health, environmental health) in addition to focus areas (e.g., minority health and health disparities, nursing) and developing research capacity. Scholars in the MMRTP seek to develop skills in mixed methods research through participation in a summer retreat followed by ongoing mentorship for one year from a mixed methods expert matched to the scholar to support their development of a research proposal. Webinars leading up to the retreat include didactic sessions taught by the same faculty each year, and the retreat itself contains multiple interactive small group sessions in which each scholar presents their project and receives feedback on their grant proposal. Due to pandemic restrictions on gatherings and travel, in 2020 the MMRTP retained all components of the program but transitioned the in-person retreat to a synchronous online retreat.
The number of NIH agencies funding mixed methods research increased from 23 in 1997–2008 to 36 in 2009–2014 [ 9 ]. The usefulness of mixed methods research aligns with several Institutes’ strategic priories, including improving health equity, enhancing feasibility, acceptability, and sustainability of interventions, and addressing patient-centeredness. However, there is a tension between growing interest in mixed methods for health sciences research and a lack of training for investigators to acquire mixed methods research skills. Mixed methods research is not routinely taught in doctoral programs, institutional grant-writing programs, nor research training that academic physicians receive. The relative lack of researchers trained in mixed methods research necessitates ongoing research capacity building and mentorship [ 10 ]. Online teaching has the potential to meet growing demand for training and mentoring in mixed methods, as evidenced by the growth of online offerings by the Mixed Methods International Research Association [ 11 ]. Yet, the nature of skills and attitudes required for doing mixed methods research, such as integration of quantitative and qualitative data collection, analysis, and epistemologies, may make this type of training difficult to adapt to an online format without compromising its effectiveness.
Few studies have attempted to evaluate mixed methods training [ 12 , 13 , 14 , 15 ] and none appear to have evaluated online trainings in mixed methods research. Our goal was to evaluate our online MMRTP by comparing the self-rated skills of scholars who experienced an in-person retreat to an online retreat across specific domains. While the MMRTP retreat is only one component of the program, assessment before and after the retreat among persons who experienced the synchronous retreat online compared to in-person provides an indication of the effectiveness of online instruction in mixed methods for specific domains critical to the design of research in health services. We hypothesized that scholars who attended the retreat online would exhibit improvements in self-rated skills comparable to scholars who attended in person.
Participants
Five cohorts with a total of 73 scholars participated in the MMRTP in person (2015–2019), while four cohorts with a total of 57 scholars participated online (2020–2023). Scholars are faculty-level researchers in the health sciences in the United States. The scholars are from a variety of disciplines in the health sciences; namely, pediatrics, psychiatry, general medicine, oncology, nursing, human development, music therapy, nutrition, psychology, and social work.
The mixed methods research training program
Formal program activities include two webinars leading up to a retreat followed by ongoing mentorship support. The mixed methods content taught in webinars and the retreat is informed by a widely used textbook by Creswell and Plano Clark [ 18 ] in addition to readings on methodological topics and the practice of mixed methods. The webinars introduce mixed methods research and integration concepts, with the goal of imparting foundational knowledge and ensuring a common language. Specifically, the first webinar introduces mixed methods concepts, research designs, scientific rigor, and becoming a resource at one’s institution, while the second focuses on strategies for the integration of qualitative and quantitative research. Retreats provide an active workshop blending lectures, one-on-one meetings, and interactive faculty-led small workgroups. In addition to scholars, core program faculty who serve as investigators and mentors for the MMRTP, supplemented with consultants and former scholars, lead the retreat. The retreat has covered the state-of-the-art topics within the context of mixed methods research: rationale for use of mixed methods, procedural diagrams, study aims, use of theory, integration strategies, sampling strategies, implementation science, randomized trials, ethics, manuscript and proposal writing, and becoming a resource at one’s home institution. In addition to lectures, the retreat includes multiple interactive small group sessions in which each scholar presents their project and receives feedback on their grant proposal and is expected to make revisions based on feedback and lectures.
Scholars are matched for one year with a mentor based on the Scholar’s needs, career level, and area of health research from a national list of affiliated experienced mixed methods investigators with demonstrated success in obtaining independent funding for research related to the health sciences and a track record and commitment to mentoring. The purpose of this arrangement is to provide different perspectives on mixed methods design while also providing specific feedback on the scholar's research proposal, reviewing new ideas, and together developing a strategy and timeline for submission.
From 2015–2019 (in-person cohorts) the retreat was held over 3 days at the Johns Hopkins University Bloomberg School of Public Health (in 2016 Harvard Catalyst, the Harvard Clinical and Translational Science Center, hosted the retreat at Harvard Medical School). Due to pandemic restrictions, from 2020–2023 the retreat activities were conducted via Zoom with the same number of lecture sessions (over 3 days in 2020 and 4 days thereafter). We made adaptations for the online retreat based on continuous feedback from attendees. We had to rapidly transition to online in 2020 with the same structure as in person, but feedback from scholars led us to extend the retreat to 4 days online from 2021–2023. The extra day allowed for more breaks from Zoom sessions with time for scholars to consider feedback from small groups and to have one-on-one meetings with mentors. Discussion during interactive presentations was encouraged and facilitated by using breakout rooms at breaks mid-presentation. Online resources were available to participants through CoursePlus, the teaching and learning platform used for courses at the Johns Hopkins Bloomberg School of Public Health, hosting publications, presentation materials, recordings of lectures, sharing proposals, email, and discussion boards that scholars have access to before, during, and after the retreat.
Measurement strategy
Before and after the retreat in each year, we distributed a self-administered scholar Mixed Methods Skills Self-Assessment instrument (Supplement 1) to all participating scholars [ 15 ]; we have reported results from this pre-post assessment for the first two cohorts [ 14 ]. The Mixed Methods Skills Self-Assessment instrument has been previously used and has established reliability for the total items (α = 0.95) and evidence of criterion-related validity between experiences and ability ratings [ 15 ]. In each year, the pre-assessment is completed upon entry to the program, approximately four months prior to the retreat, and the post-assessment is administered two weeks after the retreat. The instrument consists of three sections: 1) professional experiences with mixed methods, including background, software, and resource familiarity; 2) a quantitative, qualitative, and mixed methods skills self-assessment; and 3) open-ended questions focused on learning goals for the MMRTP. The skills assessment contains items for each of the following domains: “research questions,” “design/approach,” “sampling,” “analysis,” and “dissemination.” Each skill was assessed via three items drawn from an educational competency ratings scale that ask scholars to rate: [ 16 ] “My ability to define/explain,” “My ability to apply to practical problems,” and “Extent to which I need to improve my skill.” Response options were on a five-point Likert-type scale that ranged from “Not at all” (coded ‘1’) to “To a great extent” (coded ‘5’), including a mid-point [ 17 ]. We took the mean of the scholar’s item ratings over all component items within each domain (namely, “research questions,” “design/approach,” “sampling,” “analysis,” and “dissemination”).
Open-ended questions
The baseline survey included two open-ended prompts: 1) What skills and goals are most important to you?, and 2) What would you like to learn? The post-assessment survey also included two additional open-ended questions about the retreat: 1) What aspects of the retreat were helpful?, and 2) What would you like to change about the retreat? In addition, for the online cohorts (2020–2023), we wanted to understand reactions to the online training and added three questions for this purpose: (1) In general, what did you think of the online format for the MMRTP retreat?, 2) What mixed methods concepts are easier or harder to learn virtually?, and 3) What do you think was missing from having the retreat online rather than in person?
Data analysis
Our evaluation employed a convergent mixed methods design [ 18 ], integrating an analysis of ratings pre- and post-retreat with analysis of open-ended responses provided by scholars after the retreat. Our quantitative analysis proceeded in 3 steps. First, we analyzed item-by-item baseline ratings of the extent to which scholars thought they “need to improve skills,” stratified into two groups (5 cohorts who attended in-person and 4 cohorts who attended online). The purpose of comparing the two groups at baseline on learning needs was to assess how similar the scholars in the in-person or online groups were in self-assessment of learning needs before attending the program. Second, to examine the change in scholar ratings of ability to “define or explain a concept” and in their ability to “apply to practical problems,” from before to after the retreat, we conducted paired t-tests. The goal was to compare the ratings before and after the retreat among scholars who attended the program in person to scholars who attended online. Third, we compared post-retreat ratings among in-person cohorts to online cohorts to gauge the effectiveness of the online training. We set statistical significance at α < 0.05 as a guide to inference. We calculated Cohen’s d as a guide to the magnitude of differences [ 19 ]. SPSS Version 28 was employed for all analyses.
We analyzed qualitative data using a thematic analysis approach that consisted of reviewing all open-ended responses, conducting open coding based on the data, developing and refining a codebook, and identifying major themes [ 20 ]. We then compared the qualitative results for the in-person versus online cohorts to understand any thematic differences concerning retreat experiences and reactions.
Background and experiences of scholars
Scholars in the in-person ( n = 59, 81%) and online ( n = 52, 91%) cohorts reported their primary training was quantitative rather than qualitative or mixed methods, and scholars across cohorts commonly reported at least some exposure to mixed methods research (Table 1 ). However, most scholars did not have previous mixed methods training with 17 (23%) and 16 (28%) of the in-person and online cohorts, respectively, having previously completed a mixed methods course. While experiences were similar across in-person vs. online cohorts, there were two areas in which the scholars reported a statistically significant difference: a larger portion of the online cohorts reported writing a mixed methods application that received funding ( n = 35, 48% in person; n = 46, 81% online), and a smaller proportion of the online cohorts had given a local or institutional mixed methods presentation ( n = 32, 44% in person; n = 15, 26% online).
Self-identified need to improve skills in mixed methods
At baseline, scholars rated the extent to which they needed to improve specific mixed methods skills (Table 2 ). Overall, scholars endorsed a strong need to improve all mixed methods skills. The ratings between the in-person and online cohorts were not statistically significant for any item.
Change in self-ratings of skills after the retreat
Within cohorts.
For all domains, the differences in pre-post assessment scores were statistically significant for both the in-person and online cohorts in ability to define or explain concepts and to apply concepts to practical problems (left side of Table 3 ). In other words, on average scholars improved in both in-person and online cohorts.
Across cohorts
Online cohorts had significantly better self-ratings after the retreat than did in-person cohorts in ability to define or explain concepts and to apply concepts to practical problems (in sampling, data collection, analysis, and dissemination) but no significant differences in research questions and design / approach (rightmost column of Table 3 ).
Scholar reflections about online and in-person retreats
Goals of training.
In comparing in-person to online cohorts, discussions of the skills that scholars wanted to improve had no discernable differences. Scholars mentioned wanting to develop skills in the foundations of mixed methods research, how to write competitive proposals for funding, the use of the terminology of mixed methods research, and integrative analysis. In addition, some scholars expressed wanting to become a resource at their own institutions and providing training and mentoring to others.
Small group sessions
Scholars consistently reported appreciating being able to talk through their project and gaining feedback from experts in small group sessions. Some scholars expressed a preference for afternoon small group sessions, “The small group sessions felt the most helpful, but only because we can apply what we were learning from the morning lecture sessions” (online cohort 9). How participants discussed the benefits of the small group sessions or how they used the sessions did not depend on whether they had experienced the session in person or online.
Online participants described a tradeoff between the accessibility of a virtual retreat versus advantages of in-person training. One participant explained, “I liked the online format, as I do not have reliable childcare” (online cohort 8). Many of the scholars felt that there was an aspect of networking missing when the retreat was held fully online. As one scholar described, when learning online they, “miss getting to know the other fellows and forming lasting connections” (online cohort 9). However, an equal number of others reported that having a virtual retreat meant less hassle; for instance, they were able to join from their preferred location and did not have to travel. Some individuals specifically described the tradeoff of fewer networking opportunities for ease of attendance. One scholar wrote, being online “certainly loses some of the perks of in person connection building but made it equitable to attend” (online cohort 8).
Learning online
No clear difference in ease of learning concepts was described. A scholar explained: “Learning most concepts is essentially the same virtually versus in person” (online cohort 8). However, scholars described some concepts as easier to learn in one modality versus the other, for example, simpler concepts being more suited to learning virtually while complex concepts were better suited to in-person learning. There was notable variation though in the topics which scholars considered to be simple versus complex. For instance, one scholar noted that “I suppose developing the joint displays were a bit tougher virtually since you were not literally elbow to elbow” (online cohort 7) while another explained, “joint displays lend themselves to the zoom format” (online cohort 8).
Integrating survey responses and scholar reflections
In-person and online cohorts were comparable in professional experiences and ratings of the need to improve skills before attending the retreat, sharpening the focus on differences in self-rated skills associated with attendance online compared to in person. If anything, online attendees rated skills as good or better than in-person attendees. Open-ended questions revealed that, for the most part, scholar reflections on learning were similar across in-person and online cohorts. Whether learning the concept of “mixed methods integration” was more difficult online was a source of disagreement. Online attendance was associated with numerous advantages, and small group sessions were valued, regardless of format. Taken together, the evidence from nine cohorts shows that the online retreat was acceptable and as effective in improving self-rated skills as meeting in person.
Mixed methods have become indispensable to health services research from intervention development and testing [ 21 ] to implementation science [ 22 , 23 , 24 ]. We found that scholars participating in an interactive program to improve mixed methods skills reported significantly increased confidence in their ability to define or explain concepts and in their ability to apply the concepts to practical problems, whether the program was attended in-person or synchronously online. Scholars who participated in the online retreat had self-rated skill improvements as good or better than scholars who participated in person, and these improvements were relatively large as indicated by the Cohen’s d estimates. The online retreat appeared to be effective in increasing confidence in the use of mixed methods research in the health sciences and was acceptable to scholars. Our study deserves attention because the national need is so great for investigators with training in mixed methods to address complex behavioral health problems, community- and patient-centered research, and implementation research. No program has been evaluated as we have done here.
Aside from having written a funded mixed methods proposal, the online compared to earlier in person cohorts were comparable in experiences and need to improve specific skills. Within each cohort, scholars reported significant gains in self-rated skills on their ability to “define or explain” a concept and on their ability to “apply to practical problems” in domains essential to mixed methods research. However, consistent with our hypothesis that online training would be as effective as in person we found that online scholars reported better improvement in self-ratings in ability to define or explain concepts and to apply concepts to practical problems in sampling, data collection, analysis, and dissemination but no significant differences in research questions and design / approach. Better ratings in online cohorts could reflect differences in experience with mixed methods, secular changes in knowledge and availability of resources in mixed methods, and maturation of the program facilitated by continued modifications based on feedback from scholars and participating faculty [ 13 , 14 , 15 ].
Ratings related to the “analysis” domain, which includes the central concept of mixed methods integration, deserve notice since scholars rated this skill well below other domains at baseline. While both in-person and online cohorts improved after the retreat, and online cohorts improved substantially more than in-person cohorts, ratings for analysis after the retreat remained lower than for other domains. Scholars consistently have mentioned integration as a difficult concept, and our analysis here is limited to the retreat alone. Continued mentoring one year after the retreat and work on their proposal is built in to the MMRTP to enhance understanding of integration.
Several reviews point out the advantages of online training including savings in time, money, and greenhouse emissions [ 1 , 7 , 8 ]. Online conferences may increase the reach of training to international audiences, improve the diversity of speakers and attendees, facilitate attendance of persons with disabilities, and ease the burden of finding childcare [ 1 , 8 , 25 ]. Online training in health also appears to be effective [ 2 , 4 , 5 , 25 ], though studies are limited because often no skills were evaluated, no comparison groups were used, the response rate was low, or the sample size was small [ 1 , 6 ]. With the possible exception of networking, scholars found the online format was associated with advantages, including saving travel, maintaining work-family balance, and learning effectively. As scholars did discuss perceived increase in difficulty networking, deliberate effort needs to be directed at enhancing collaborations and mentorship [ 8 ]. The MMRTP was designed with components to facilitate networking during and beyond the retreat (e.g., small group sessions, one-on-one meetings, working with a consultant on a specific proposal).
Limitations of our study should be considered. First, the retreat was only one of several components of a mentoring program for faculty in the health sciences. Second, in-person and online cohorts represent different time periods spanning 9 years during which mixed methods applications to NIH and other funders have been increasing [ 9 ]. Third, the pre- and post-evaluations of ability to explain or define concepts, or to apply the concepts to practical problems, were based on self-report. Nevertheless, the pre-post retreat survey on self-rated skills uses a skills self-assessment form we developed [ 15 ], drawing from educational theory related to the epistemology of knowledge [ 26 , 27 ].
Despite the central role of mixed methods in health research, studies evaluating online methods training in the health sciences are nonexistent. Our study provides evidence that mixed methods training online was associated with the same increases in self-rated skills as persons attending online and can be a key component to increasing the capacity for mixed methods research in the health sciences.
Availability of data and materials
The datasets used and analysed during the current study are available from the corresponding author on reasonable request.
Abbreviations
Mixed Methods Research Training Program
Wilcha RJ. Effectiveness of Virtual Medical Teaching During the COVID-19 Crisis: Systematic Review. JMIR Med Educ. 2020;6(2):e20963.
Article Google Scholar
Pei L, Wu H. Does online learning work better than offline learning in undergraduate medical education? A systematic review and meta-analysis. Medical Education Online. 2019;24(1) https://doi.org/10.1080/10872981.2019.1666538
Barche A, Nayak V, Pandey A, Bhandarkar A, Nayak K. Student perceptions towards online learning in medical education during the COVID-19 pandemic: a mixed-methods study. F1000Res. 2022;11:979. https://doi.org/10.12688/f1000research.123582.1 .
Ebner C, Gegenfurtner A. Learning and Satisfaction in Webinar, Online, and Face-to-Face Instruction: A Meta-Analysis. Frontiers in Education. 2019;4(92) https://doi.org/10.3389/feduc.2019.00092
Randazzo M, Preifer R, Khamis-Dakwar R. Project-Based Learning and Traditional Online Teaching of Research Methods During COVID-19: An Investigation of Research Self-Efficacy and Student Satisfaction. Frontiers in Education. 2021;6(662850) https://doi.org/10.3389/feduc.2021.662850
Chan A, Cao A, Kim L, et al. Comparison of perceived educational value of an in-person versus virtual medical conference. Can Med Educ J. 2021;12(4):65–9. https://doi.org/10.36834/cmej.71975 .
Rubinger L, Gazendam A, Ekhtiari S, et al. Maximizing virtual meetings and conferences: a review of best practices. Int Orthop. 2020;44(8):1461–6. https://doi.org/10.1007/s00264-020-04615-9 .
Sarabipour S. Virtual conferences raise standards for accessibility and interactions. Elife. Nov 4 2020;9 https://doi.org/10.7554/eLife.62668
Coyle CE, Schulman-Green D, Feder S, et al. Federal funding for mixed methods research in the health sciences in the United States: Recent trends. J Mixed Methods Res. 2018;12(3):1–20.
Poth C, Munce SEP. Commentary – preparing today’s researchers for a yet unknown tomorrow: promising practices for a synergistic and sustainable mentoring approach to mixed methods research learning. Int J Multiple Res Approaches. 2020;12(1):56–64.
Creswell JW. Reflections on the MMIRA The Future of Mixed Methods Task Force Report. J Mixed Methods Res. 2016;10(3):215–9. https://doi.org/10.1177/1558689816650298 .
Hou S. A Mixed Methods Process Evaluation of an Integrated Course Design on Teaching Mixed Methods Research. Int J Sch Teach Learn. 2021;15(2):Article 8. https://doi.org/10.20429/ijsotl.2021.150208 .
Guetterman TC, Creswell J, Deutsch C, Gallo JJ. Process Evaluation of a Retreat for Scholars in the First Cohort: The NIH Mixed Methods Research Training Program for the Health Sciences. J Mix Methods Res. 2019;13(1):52–68. https://doi.org/10.1177/1558689816674564 .
Guetterman T, Creswell JW, Deutsch C, Gallo JJ. Skills Development and Academic Productivity of Scholars in the NIH Mixed Methods Research Training Program for the Health Sciences (invited publication). Int J Multiple Res Approach. 2018;10(1):1–17.
Guetterman T, Creswell JW, Wittink MN, et al. Development of a Self-Rated Mixed Methods Skills Assessment: The NIH Mixed Methods Research Training Program for the Health Sciences. J Contin Educ Health Prof. 2017;37(2):76–82.
Harnisch D, Shope RJ. Developing technology competencies to enhance assessment literate teachers. AACE; 2007:3053–3055.
DeVellis RF. Scale development: Theory and applications. 3rd ed. Sage; 2012.
Creswell JW, Plano Clark VL. Designing and Conducting Mixed Methods Research. 3rd ed. Sage Publications; 2017.
Cohen J. Statistical power analysis for the behavioral sciences. 3rd ed. Academic Press; 1988.
Boeije H. A purposeful approach to the constant comparative method in the analysis of qualitative interviews. Qual Quant. 2002;36:391–409.
Aschbrenner KA, Kruse G, Gallo JJ, Plano Clark VL. Applying mixed methods to pilot feasibility studies to inform intervention trials. Pilot Feasibility Stud. 2022;8(1):217–24. https://doi.org/10.1186/s40814-022-01178-x .
Palinkas LA. Qualitative and mixed methods in mental health services and implementation research. J Clin Child Adolesc Psychol. 2014;43(6):851–61.
Albright K, Gechter K, Kempe A. Importance of mixed methods in pragmatic trials and dissemination and implementation research. Acad Pediatr Sep-Oct. 2013;13(5):400–7. https://doi.org/10.1016/j.acap.2013.06.010 .
Palinkas L, Aarons G, Horwitz S, Chamberlain P, Hurlburt M, Landsverk J. Mixed methods designs in implementation research. Adm Policy Ment Health. 2011;38:44–53.
Ni AY. Comparing the Effectiveness of Classroom and Online Learning: Teaching Research Methods. J Public Affairs Educ. 2013;19(2):199–215. https://doi.org/10.1080/15236803.2013.12001730 .
Harnisch D, Shope RJ. Developing technology competencies to enhance assessment literate teachers. presented at: Society for Information Technology & Teacher Education International Conference; March 26, 2007 2007; San Antonio, Texas.
Guetterman TC. What distinguishes a novice from an expert mixed methods researcher? Qual Quantity. 2017;51:377–98.
Download references
Acknowledgements
The Mixed Methods Research Training Program is supported by the Office of Behavioral and Social Sciences Research under Grant R25MH104660. Participating institutes are the National Institute of Mental Health, National Heart, Lung, and Blood Institute, National Institute of Nursing Research, and the National Institute on Aging.
Author information
Authors and affiliations.
Johns Hopkins University, Baltimore, MD, USA
Joseph J. Gallo & Sarah M. Murray
University of Michigan, Ann Arbor, MI, USA
John W. Creswell & Timothy C. Guetterman
Harvard University, Boston, MA, USA
Charles Deutsch
You can also search for this author in PubMed Google Scholar
Contributions
All authors conceptualized the design of this study. TG analyzed the scholar data in evaluation of the program. TG and JG interpreted results and were major contributors in writing the manuscript. All authors read and approved the final manuscript.
Corresponding author
Correspondence to Timothy C. Guetterman .
Ethics declarations
Ethics approval and consent to participate.
The program was reviewed by the Johns Hopkins Institutional Review Board and was deemed exempt as educational research under United States 45 CFR 46.101(b), Category (2). Data were collected through an anonymous survey. Consent to participate was waived.
Consent for publication
Not applicable.
Competing interests
The authors declare no competing interests.
Additional information
Publisher’s note.
Springer Nature remains neutral with regard to jurisdictional claims in published maps and institutional affiliations.
Supplementary Information
Supplementary material 1, rights and permissions.
Open Access This article is licensed under a Creative Commons Attribution 4.0 International License, which permits use, sharing, adaptation, distribution and reproduction in any medium or format, as long as you give appropriate credit to the original author(s) and the source, provide a link to the Creative Commons licence, and indicate if changes were made. The images or other third party material in this article are included in the article's Creative Commons licence, unless indicated otherwise in a credit line to the material. If material is not included in the article's Creative Commons licence and your intended use is not permitted by statutory regulation or exceeds the permitted use, you will need to obtain permission directly from the copyright holder. To view a copy of this licence, visit http://creativecommons.org/licenses/by/4.0/ . The Creative Commons Public Domain Dedication waiver ( http://creativecommons.org/publicdomain/zero/1.0/ ) applies to the data made available in this article, unless otherwise stated in a credit line to the data.
Reprints and permissions
About this article
Cite this article.
Gallo, J.J., Murray, S.M., Creswell, J.W. et al. Going virtual: mixed methods evaluation of online versus in-person learning in the NIH mixed methods research training program retreat. BMC Med Educ 24 , 882 (2024). https://doi.org/10.1186/s12909-024-05877-2
Download citation
Received : 15 January 2024
Accepted : 08 August 2024
Published : 16 August 2024
DOI : https://doi.org/10.1186/s12909-024-05877-2
Share this article
Anyone you share the following link with will be able to read this content:
Sorry, a shareable link is not currently available for this article.
Provided by the Springer Nature SharedIt content-sharing initiative
- Research training
- Mixed methods research
- Research capacity building
- Online education
- Teaching methods
BMC Medical Education
ISSN: 1472-6920
- Submission enquiries: [email protected]
- General enquiries: [email protected]

IMAGES
COMMENTS
Learn how to create a research design for your study using empirical data. Find out the differences between qualitative and quantitative approaches, types of research designs, and how to choose your methods.
Research study design is a framework, or the set of methods and procedures used to collect and analyze data on variables specified in a particular research problem. Research study designs are of many types, each with its advantages and limitations. The type of study design used to answer a particular research question is determined by the ...
Learn about the two main types of study designs: descriptive and analytical. Descriptive studies describe characteristics in a population, while analytical studies compare groups or interventions. See examples of cross-sectional, cohort, case-control, and experimental studies.
A research design is defined as the overall plan or structure that guides the process of conducting research. It is a critical component of the research process and serves as a blueprint for how a study will be carried out, including the methods and techniques that will be used to collect and analyze data.
Ranganathan P. Understanding Research Study Designs. Indian J Crit Care Med 2019;23 (Suppl 4):S305-S307. Keywords: Clinical trials as topic, Observational studies as topic, Research designs. We use a variety of research study designs in biomedical research. In this article, the main features of each of these designs are summarized. Go to:
Introduction. In clinical research, our aim is to design a study, which would be able to derive a valid and meaningful scientific conclusion using appropriate statistical methods that can be translated to the "real world" setting. 1 Before choosing a study design, one must establish aims and objectives of the study, and choose an appropriate target population that is most representative of ...
Learn what research design is and how to write one. Find out the different types of research design, such as descriptive, correlational, experimental, and case study, and see an example of each.
Learn the basics of research design, the overall plan that guides a research project from start to finish. Explore the four types of quantitative research designs (descriptive, correlational, experimental, and quasi-experimental) and how to choose the best one for your study.
Research Study Designs in the Health Sciences (29:36 min): An overview of research study designs used by health sciences researchers. Covers case reports/case series, case control studies, cohort studies, correlational studies, cross-sectional studies, experimental studies (including randomized control trials), systematic reviews and meta-analysis.
Introduction. Study designs are frameworks used in medical research to gather data and explore a specific research question.. Choosing an appropriate study design is one of many essential considerations before conducting research to minimise bias and yield valid results.. This guide provides a summary of study designs commonly used in medical research, their characteristics, advantages and ...
A research design is the plan or framework used to conduct a research study. It involves outlining the overall approach and methods that will be used to collect and analyze data in order to answer research questions or test hypotheses.
The research design refers to the overall strategy and analytical approach that you have chosen in order to integrate, in a coherent and logical way, the different components of the study, thus ensuring that the research problem will be thoroughly investigated. It constitutes the blueprint for the collection, measurement, and interpretation of ...
Types of Research Designs Compared | Guide & Examples. Published on June 20, 2019 by Shona McCombes.Revised on June 22, 2023. When you start planning a research project, developing research questions and creating a research design, you will have to make various decisions about the type of research you want to do.. There are many ways to categorize different types of research.
If your study system doesn't match these criteria, there are other types of research you can use to answer your research question. Step 3: Design your experimental treatments How you manipulate the independent variable can affect the experiment's external validity - that is, the extent to which the results can be generalized and applied ...
First, by the specific research question. That is, if the question is one of 'prevalence' (disease burden) then the ideal is a cross-sectional study; if it is a question of 'harm' - a case-control study; prognosis - a cohort and therapy - a RCT. Second, by what resources are available to you. This includes budget, time, feasibility re-patient ...
Research study design is a framework, or the set of methods and procedures used to collect and analyze data on variables specified in a particular research problem. Research study designs are of many types, each with its advantages and limitations. The type of study design used to answer a particular research question is determined by the ...
The study design used to answer a particular research question depends on the nature of the question and the availability of resources. In this article, which is the first part of a series on "study designs," we provide an overview of research study designs and their classification. The subsequent articles will focus on individual designs.
Study design refers to the methods and methodologies used in resea rch to gather the data needed to explore a specific question. Some research questions are best approached by statistical analysis of data. This is quantitative research. Others are better answered by looking for patterns, features or themes in the data.
When are analytical study designs used? A study design is a systematic plan, developed so you can carry out your research study effectively and efficiently. Having a design is important because it will determine the right methodologies for your study. Using the right study design makes your results more credible, valid, and coherent.
A clinical study design includes the preparation of trials, experiments, and observations in research involving human beings. The various types of study designs are depicted in Fig. 8.1. Fig. 8.1. A study can be classified into three major groups: observational, experimental, and meta-analysis. Full size image.
Types of study design. Medical research is classified into primary and secondary research. Clinical/experimental studies are performed in primary research, whereas secondary research consolidates available studies as reviews, systematic reviews and meta-analyses. Three main areas in primary research are basic medical research, clinical research ...
The research design refers to the overall strategy that you choose to integrate the different components of the study in a coherent and logical way, thereby, ensuring you will effectively address the research problem; it constitutes the blueprint for the collection, measurement, and analysis of data.
II Overview of Study Design, Research Aims and Hypotheses Overview of Study Design. Our study design was based on our conceptual model proposing that helping and sharing are key prosocial behaviors that are supported by cognitive-affective processes and collaborative social contexts. We developed a multifaceted intervention protocol that ...
The chapter sets out the research design and questions for the case studies in the second part of the book. It discusses what is being examined with respect to all the systems studied and how it ...
Research in design entails the systematic study of the artifact creation process and its integration into various environments — including virtual, physical, social, psychological, economic and political. This focus area aims to enhance the practice of design engineering.
The current research interest in DDE lies in evidence-based co-design practices, design issues in digital mental health, acceptance and humanization of digital technologies, digital design for ...
For a professional paper, the affiliation is the institution at which the research was conducted. Include both the name of any department and the name of the college, university, or other institution, separated by a comma. Center the affiliation on the next double-spaced line after the author names; when there are multiple affiliations, center ...
Quantitative research methods. You can use quantitative research methods for descriptive, correlational or experimental research. In descriptive research, you simply seek an overall summary of your study variables.; In correlational research, you investigate relationships between your study variables.; In experimental research, you systematically examine whether there is a cause-and-effect ...
Despite the central role of mixed methods in health research, studies evaluating online methods training in the health sciences are nonexistent. The focused goal was to evaluate online training by comparing the self-rated skills of scholars who experienced an in-person retreat to scholars in an online retreat in specific domains of mixed methods research for the health sciences from 2015-2023.
These include study designs for two-stage analyses, distributed lag linear and non-linear models as well as other regression methods, advanced meta-analytical techniques for pooling complex association estimates and explore heterogeneity across locations, quantification of excess risk, spatio-temporal analysis and extrapolation methods, and ...